Open Access
ARTICLE
Modeling and TOPSIS-GRA Algorithm for Autonomous Driving Decision-Making Under 5G-V2X Infrastructure
1 Department of Logistics Engineering, Chongqing University of Arts and Sciences, Chongqing, 402160, China
2 School of Mechanical & Automotive Engineering, South China University of Technology, Guangzhou, 510641, China
* Corresponding Author: Shijun Fu. Email:
Computers, Materials & Continua 2023, 75(1), 1051-1071. https://doi.org/10.32604/cmc.2023.034495
Received 17 July 2022; Accepted 23 November 2022; Issue published 06 February 2023
Abstract
This paper is to explore the problems of intelligent connected vehicles (ICVs) autonomous driving decision-making under a 5G-V2X structured road environment. Through literature review and interviews with autonomous driving practitioners, this paper firstly puts forward a logical framework for designing a cerebrum-like autonomous driving system. Secondly, situated on this framework, it builds a hierarchical finite state machine (HFSM) model as well as a TOPSIS-GRA algorithm for making ICV autonomous driving decisions by employing a data fusion approach between the entropy weight method (EWM) and analytic hierarchy process method (AHP) and by employing a model fusion approach between the technique for order preference by similarity to an ideal solution (TOPSIS) and grey relational analysis (GRA). The HFSM model is composed of two layers: the global FSM model and the local FSM model. The decision of the former acts as partial input information of the latter and the result of the latter is sent forward to the local path-planning module, meanwhile pulsating feedback to the former as real-time refresh data. To identify different traffic scenarios in a cerebrum-like way, the global FSM model is designed as 7 driving behavior states and 17 driving characteristic events, and the local FSM model is designed as 16 states and 8 characteristic events. In respect to designing a cerebrum-like algorithm for state transition, this paper firstly fuses AHP weight and EWM weight at their output layer to generate a synthetic weight coefficient for each characteristic event; then, it further fuses TOPSIS method and GRA method at the model building layer to obtain the implementable order of state transition. To verify the feasibility, reliability, and safety of the HFSM model as well as its TOPSIS-GRA state transition algorithm, this paper elaborates on a series of simulative experiments conducted on the PreScan8.50 platform. The results display that the accuracy of obstacle detection gets 98%, lane line prediction is beyond 70 m, the speed of collision avoidance is higher than 45 km/h, the distance of collision avoidance is less than 5 m, path planning time for obstacle avoidance is averagely less than 50 ms, and brake deceleration is controlled under 6 m/s2. These technical indexes support that the driving states set and characteristic events set for the HFSM model as well as its TOPSIS-GRA algorithm may bring about cerebrum-like decision-making effectiveness for ICV autonomous driving under 5G-V2X intelligent road infrastructure.Keywords
Cite This Article
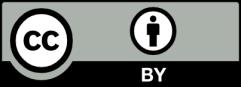
This work is licensed under a Creative Commons Attribution 4.0 International License , which permits unrestricted use, distribution, and reproduction in any medium, provided the original work is properly cited.