Open Access
ARTICLE
Combined Effect of Concept Drift and Class Imbalance on Model Performance During Stream Classification
1 Department of Computer and Information Sciences, Universiti Teknologi PETRONAS (UTP), Seri Iskandar, 32610, Malaysia
2 Centre for Research in Data Science, UTP, Perak, 32610, Malaysia
3 High Performance Cloud Computing Centre (HPC3), UTP, Perak, 32610, Malaysia
4 School of Engineering and Computer Science, Victoria University of Wellington, Wellington, 6012, New Zealand
5 AI Institute, University of Waikato Wellington, Hamilton, 3240, New Zealand
6 Anti-Narcotics Force, Ministry of Narcotics Control, Islamabad, 46000, Pakistan
7 College of Computer Science and Engineering, Taibah University, Madinah, 42353, Saudi Arabia
* Corresponding Author: Abdul Sattar Palli. Email:
Computers, Materials & Continua 2023, 75(1), 1827-1845. https://doi.org/10.32604/cmc.2023.033934
Received 01 July 2022; Accepted 12 October 2022; Issue published 06 February 2023
Abstract
Every application in a smart city environment like the smart grid, health monitoring, security, and surveillance generates non-stationary data streams. Due to such nature, the statistical properties of data changes over time, leading to class imbalance and concept drift issues. Both these issues cause model performance degradation. Most of the current work has been focused on developing an ensemble strategy by training a new classifier on the latest data to resolve the issue. These techniques suffer while training the new classifier if the data is imbalanced. Also, the class imbalance ratio may change greatly from one input stream to another, making the problem more complex. The existing solutions proposed for addressing the combined issue of class imbalance and concept drift are lacking in understating of correlation of one problem with the other. This work studies the association between concept drift and class imbalance ratio and then demonstrates how changes in class imbalance ratio along with concept drift affect the classifier’s performance. We analyzed the effect of both the issues on minority and majority classes individually. To do this, we conducted experiments on benchmark datasets using state-of-the-art classifiers especially designed for data stream classification. Precision, recall, F1 score, and geometric mean were used to measure the performance. Our findings show that when both class imbalance and concept drift problems occur together the performance can decrease up to 15%. Our results also show that the increase in the imbalance ratio can cause a 10% to 15% decrease in the precision scores of both minority and majority classes. The study findings may help in designing intelligent and adaptive solutions that can cope with the challenges of non-stationary data streams like concept drift and class imbalance.Keywords
Cite This Article
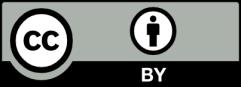