Open Access
ARTICLE
Optimal Machine Learning Driven Sentiment Analysis on COVID-19 Twitter Data
1 Information Systems Department, Faculty of Computing and Information Technology, King Abdulaziz University, Jeddah, 21589, Saudi Arabia
2 Center of Excellence in Smart Environment Research, King Abdulaziz University, Jeddah, 21589, Saudi Arabia
3 Information Systems Department, HECI School, Dar Alhekma University, Jeddah, Saudi Arabia
4 Information Technology Department, Faculty of Computing and Information Technology, King Abdulaziz University, Jeddah, 21589, Saudi Arabia
5 Department of Mathematics, Faculty of Science, Al-Azhar University, Naser City, 11884, Cairo, Egypt
6 Centre for Artificial Intelligence in Precision Medicines, King Abdulaziz University, Jeddah, 21589, Saudi Arabia
* Corresponding Author: Mahmoud Ragab. Email:
Computers, Materials & Continua 2023, 75(1), 81-97. https://doi.org/10.32604/cmc.2023.033406
Received 15 June 2022; Accepted 15 September 2022; Issue published 06 February 2023
Abstract
The outbreak of the pandemic, caused by Coronavirus Disease 2019 (COVID-19), has affected the daily activities of people across the globe. During COVID-19 outbreak and the successive lockdowns, Twitter was heavily used and the number of tweets regarding COVID-19 increased tremendously. Several studies used Sentiment Analysis (SA) to analyze the emotions expressed through tweets upon COVID-19. Therefore, in current study, a new Artificial Bee Colony (ABC) with Machine Learning-driven SA (ABCML-SA) model is developed for conducting Sentiment Analysis of COVID-19 Twitter data. The prime focus of the presented ABCML-SA model is to recognize the sentiments expressed in tweets made upon COVID-19. It involves data pre-processing at the initial stage followed by n-gram based feature extraction to derive the feature vectors. For identification and classification of the sentiments, the Support Vector Machine (SVM) model is exploited. At last, the ABC algorithm is applied to fine tune the parameters involved in SVM. To demonstrate the improved performance of the proposed ABCML-SA model, a sequence of simulations was conducted. The comparative assessment results confirmed the effectual performance of the proposed ABCML-SA model over other approaches.Keywords
Cite This Article
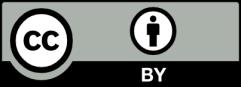
This work is licensed under a Creative Commons Attribution 4.0 International License , which permits unrestricted use, distribution, and reproduction in any medium, provided the original work is properly cited.