Open Access
ARTICLE
Arithmetic Optimization with Ensemble Deep Transfer Learning Based Melanoma Classification
1 Department of Computer Science, Dr. Nalli Kuppusamy Arts College (Affiliated to Bharathidasan University, Tiruchirappalli), Thanjavur, 613003, India
2 Department of Computer Science, College of Computer and Information Sciences, Majmaah University, Al-Majmaah, 11952, Saudi Arabia
3 Lepartment of Computer Engineering, College of Computer Engineering & Sciences, Prince Sattam Bin Abdulaziz University, Al-Kharj, 11942, Saudi Arabia
4 Department of Computer Science and Engineering, Vignan’s Institute of Information Technology, Visakhapatnam, 530049, India
5 Department of Applied Data Science, Noroff University College, Kristiansand, Norway
6 Department of ICT Convergence, Soonchunhyang University, Korea
* Corresponding Author: Yunyoung Nam. Email:
Computers, Materials & Continua 2023, 75(1), 149-164. https://doi.org/10.32604/cmc.2023.033005
Received 04 June 2022; Accepted 05 July 2022; Issue published 06 February 2023
Abstract
Melanoma is a skin disease with high mortality rate while early diagnoses of the disease can increase the survival chances of patients. It is challenging to automatically diagnose melanoma from dermoscopic skin samples. Computer-Aided Diagnostic (CAD) tool saves time and effort in diagnosing melanoma compared to existing medical approaches. In this background, there is a need exists to design an automated classification model for melanoma that can utilize deep and rich feature datasets of an image for disease classification. The current study develops an Intelligent Arithmetic Optimization with Ensemble Deep Transfer Learning Based Melanoma Classification (IAOEDTT-MC) model. The proposed IAOEDTT-MC model focuses on identification and classification of melanoma from dermoscopic images. To accomplish this, IAOEDTT-MC model applies image preprocessing at the initial stage in which Gabor Filtering (GF) technique is utilized. In addition, U-Net segmentation approach is employed to segment the lesion regions in dermoscopic images. Besides, an ensemble of DL models including ResNet50 and ElasticNet models is applied in this study. Moreover, AO algorithm with Gated Recurrent Unit (GRU) method is utilized for identification and classification of melanoma. The proposed IAOEDTT-MC method was experimentally validated with the help of benchmark datasets and the proposed model attained maximum accuracy of 92.09% on ISIC 2017 dataset.Keywords
Cite This Article
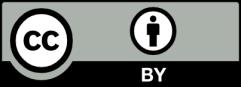
This work is licensed under a Creative Commons Attribution 4.0 International License , which permits unrestricted use, distribution, and reproduction in any medium, provided the original work is properly cited.