Open Access
ARTICLE
Automated Artificial Intelligence Empowered White Blood Cells Classification Model
1 Faculty of Economics and Administration, King Abdulaziz University, Jeddah, Saudi Arabia
2 Department of Management Information Systems, College of Business Administration, Taibah University, Al-Madinah, Saudi Arabia
3 E-commerce Department, College of Administrative and Financial Sciences, Saudi Electronic University, Jeddah, Saudi Arabia
4 School of Computer Science & Engineering (SCOPE), VIT-AP University, Amaravati, Andhra Pradesh, India
5 Department of Computer Science and Engineering, Vignan’s Institute of Information Technology, (Autonomous), Visakhapatnam, Andhra Pradesh, 530049, India
* Corresponding Author: E. Laxmi Lydia. Email:
Computers, Materials & Continua 2023, 75(1), 409-425. https://doi.org/10.32604/cmc.2023.032432
Received 18 May 2022; Accepted 21 June 2022; Issue published 06 February 2023
Abstract
White blood cells (WBC) or leukocytes are a vital component of the blood which forms the immune system, which is accountable to fight foreign elements. The WBC images can be exposed to different data analysis approaches which categorize different kinds of WBC. Conventionally, laboratory tests are carried out to determine the kind of WBC which is erroneous and time consuming. Recently, deep learning (DL) models can be employed for automated investigation of WBC images in short duration. Therefore, this paper introduces an Aquila Optimizer with Transfer Learning based Automated White Blood Cells Classification (AOTL-WBCC) technique. The presented AOTL-WBCC model executes data normalization and data augmentation process (rotation and zooming) at the initial stage. In addition, the residual network (ResNet) approach was used for feature extraction in which the initial hyperparameter values of the ResNet model are tuned by the use of AO algorithm. Finally, Bayesian neural network (BNN) classification technique has been implied for the identification of WBC images into distinct classes. The experimental validation of the AOTL-WBCC methodology is performed with the help of Kaggle dataset. The experimental results found that the AOTL-WBCC model has outperformed other techniques which are based on image processing and manual feature engineering approaches under different dimensions.Keywords
Cite This Article
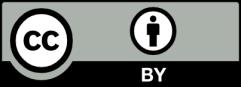
This work is licensed under a Creative Commons Attribution 4.0 International License , which permits unrestricted use, distribution, and reproduction in any medium, provided the original work is properly cited.