Open Access
ARTICLE
Adaptive Dynamic Dipper Throated Optimization for Feature Selection in Medical Data
1 Department of Information Technology, College of Computer and Information Sciences, Princess Nourah bint Abdulrahman University, P. O. Box 84428, Riyadh, 11671, Saudi Arabia
2 Department of Communications and Electronics, Delta Higher Institute of Engineering and Technology, Mansoura, 35111, Egypt
3 Faculty of Artificial Intelligence, Delta University for Science and Technology, Mansoura, 35712, Egypt
4 Department of Computer Sciences, College of Computer and Information Sciences, Princess Nourah bint Abdulrahman University, P. O. Box 84428, Riyadh, 11671, Saudi Arabia
5 Computer Engineering and Control Systems Department, Faculty of Engineering, Mansoura University, Mansoura, 35516, Egypt
6 Department of Computer Science, Faculty of Computer and Information Sciences, Ain Shams University, Cairo, 11566, Egypt
7 Department of Computer Science, College of Computing and Information Technology, Shaqra University, 11961, Saudi Arabia
8 Faculty of Computers and Artificial Intelligence, Benha University, Benha, 13518, Egypt
9 College of Computer and Information Sciences, Prince Sultan University, Riyadh, 11586, Saudi Arabia
10 Department of Civil Engineering, University of Science and Technology, Miami, 33101, USA
11 Department of Civil and Environmental Engineering, Florida International University, Miami, USA
12 Oral Biology Department, Faculty of Oral and Dental Medicine, Delta University for Science and Technology, Gamasa, Egypt
13 Faculty of Artificial Intelligence, Kafrelsheikh University, Kafrelsheikh, 33511, Egypt
* Corresponding Author: Nagwan Abdel Samee. Email:
Computers, Materials & Continua 2023, 75(1), 1883-1900. https://doi.org/10.32604/cmc.2023.031723
Received 25 April 2022; Accepted 08 June 2022; Issue published 06 February 2023
Abstract
The rapid population growth results in a crucial problem in the early detection of diseases in medical research. Among all the cancers unveiled, breast cancer is considered the second most severe cancer. Consequently, an exponential rising in death cases incurred by breast cancer is expected due to the rapid population growth and the lack of resources required for performing medical diagnoses. Utilizing recent advances in machine learning could help medical staff in diagnosing diseases as they offer effective, reliable, and rapid responses, which could help in decreasing the death risk. In this paper, we propose a new algorithm for feature selection based on a hybrid between powerful and recently emerged optimizers, namely, guided whale and dipper throated optimizers. The proposed algorithm is evaluated using four publicly available breast cancer datasets. The evaluation results show the effectiveness of the proposed approach from the accuracy and speed perspectives. To prove the superiority of the proposed algorithm, a set of competing feature selection algorithms were incorporated into the conducted experiments. In addition, a group of statistical analysis experiments was conducted to emphasize the superiority and stability of the proposed algorithm. The best-achieved breast cancer prediction average accuracy based on the proposed algorithm is 99.453%. This result is achieved in an average time of 3.6725 s, the best result among all the competing approaches utilized in the experiments.Keywords
Cite This Article
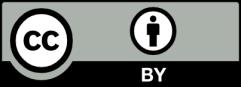
This work is licensed under a Creative Commons Attribution 4.0 International License , which permits unrestricted use, distribution, and reproduction in any medium, provided the original work is properly cited.