Open Access
ARTICLE
Automated Brain Hemorrhage Classification and Volume Analysis
1 Department of Computer Science, Air University Multan Campus, Multan, 60000, Pakistan
2 Department of Information Technology, Bahauddin Zakariya University, Multan, 60800, Pakistan
3 Department of Engineering and Computer Science, College of Computer Science and Information Technology, Al Baha University, Al Baha, Saudi Arabia
4 School of Computer Science & Information Technology, King Abdulaziz University, Jeddah, Saudi Arabia
5 Department of Intelligent Mechatronics Engineering, Sejong University, Seoul, 05006, Korea
* Corresponding Author: Muhammad Mateen. Email:
Computers, Materials & Continua 2023, 75(1), 2283-2299. https://doi.org/10.32604/cmc.2023.030706
Received 31 March 2022; Accepted 14 July 2022; Issue published 06 February 2023
Abstract
Brain hemorrhage is a serious and life-threatening condition. It can cause permanent and lifelong disability even when it is not fatal. The word hemorrhage denotes leakage of blood within the brain and this leakage of blood from capillaries causes stroke and adequate supply of oxygen to the brain is hindered. Modern imaging methods such as computed tomography (CT) and magnetic resonance imaging (MRI) are employed to get an idea regarding the extent of the damage. An early diagnosis and treatment can save lives and limit the adverse effects of a brain hemorrhage. In this case, a deep neural network (DNN) is an effective choice for the early identification and classification of brain hemorrhage for the timely recovery and treatment of an affected person. In this paper, the proposed research work is divided into two novel approaches, where, one for the classification and the other for volume calculation of brain hemorrhage. Two different datasets are used for two different techniques classification and volume. A novel algorithm is proposed to calculate the volume of hemorrhage using CT scan images. In the first approach, the ‘RSNA’ dataset is used to classify the brain hemorrhage types using transfer learning and achieved an accuracy of 93.77%. Furthermore, in the second approach, a novel algorithm has been proposed to calculate the volume of brain hemorrhage and achieved tremendous results as 1035.91 mm3 and 9.25 cm3, using the PhysioNet CT scan tomography dataset.Keywords
Cite This Article
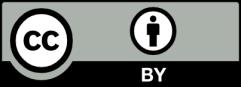
This work is licensed under a Creative Commons Attribution 4.0 International License , which permits unrestricted use, distribution, and reproduction in any medium, provided the original work is properly cited.