Open Access
ARTICLE
Data Augmentation and Random Multi-Model Deep Learning for Data Classification
1 Computer Science Department, Future Academy-Higher Future Institute for Specialized Technological Studies, Egypt
2 Department of Computer Systems Engineering, Faculty of Electrical and Computer Engineering, University of
Engineering and Technology, Peshawar, 25120, Pakistan
3 Department of Information Technology, College of Computer, Qassim University, Buraydah, Saudi Arabia
4 Department of Computer Sciences, Faculty of Sciences of Gafsa, University of Gafsa, Gafsa, Tunisia
* Corresponding Author: Suliman Aladhadh. Email:
Computers, Materials & Continua 2023, 74(3), 5191-5207. https://doi.org/10.32604/cmc.2022.029420
Received 03 March 2022; Accepted 06 June 2022; Issue published 28 December 2022
Abstract
In the machine learning (ML) paradigm, data augmentation serves as a regularization approach for creating ML models. The increase in the diversification of training samples increases the generalization capabilities, which enhances the prediction performance of classifiers when tested on unseen examples. Deep learning (DL) models have a lot of parameters, and they frequently overfit. Effectively, to avoid overfitting, data plays a major role to augment the latest improvements in DL. Nevertheless, reliable data collection is a major limiting factor. Frequently, this problem is undertaken by combining augmentation of data, transfer learning, dropout, and methods of normalization in batches. In this paper, we introduce the application of data augmentation in the field of image classification using Random Multi-model Deep Learning (RMDL) which uses the association approaches of multiDL to yield random models for classification. We present a methodology for using Generative Adversarial Networks (GANs) to generate images for data augmenting. Through experiments, we discover that samples generated by GANs when fed into RMDL improve both accuracy and model efficiency. Experimenting across both MNIST and CIAFAR-10 datasets show that, error rate with proposed approach has been decreased with different random models.Keywords
Cite This Article
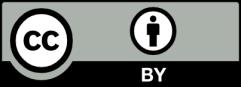
This work is licensed under a Creative Commons Attribution 4.0 International License , which permits unrestricted use, distribution, and reproduction in any medium, provided the original work is properly cited.