Open Access
ARTICLE
Improving Brain Tumor Classification with Deep Learning Using Synthetic Data
1 Elmadag Vocational School, Department of Computer Technologies, Ankara University, Ankara, Turkey
2 Faculty of Technology, Department of Software Engineering, Sivas Cumhuriyet University, Sivas, 58140, Turkey
3 Faculty of Engineering, Department of Computer Engineering, Sivas Cumhuriyet University, Sivas, 58140, Turkey
* Corresponding Author: Rukiye Karakis. Email:
Computers, Materials & Continua 2023, 74(3), 5049-5067. https://doi.org/10.32604/cmc.2023.035584
Received 26 August 2022; Accepted 28 September 2022; Issue published 28 December 2022
Abstract
Deep learning (DL) techniques, which do not need complex pre-processing and feature analysis, are used in many areas of medicine and achieve promising results. On the other hand, in medical studies, a limited dataset decreases the abstraction ability of the DL model. In this context, we aimed to produce synthetic brain images including three tumor types (glioma, meningioma, and pituitary), unlike traditional data augmentation methods, and classify them with DL. This study proposes a tumor classification model consisting of a Dense Convolutional Network (DenseNet121)-based DL model to prevent forgetting problems in deep networks and delay information flow between layers. By comparing models trained on two different datasets, we demonstrated the effect of synthetic images generated by Cycle Generative Adversarial Network (CycleGAN) on the generalization of DL. One model is trained only on the original dataset, while the other is trained on the combined dataset of synthetic and original images. Synthetic data generated by CycleGAN improved the best accuracy values for glioma, meningioma, and pituitary tumor classes from 0.9633, 0.9569, and 0.9904 to 0.9968, 0.9920, and 0.9952, respectively. The developed model using synthetic data obtained a higher accuracy value than the related studies in the literature. Additionally, except for pixel-level and affine transform data augmentation, synthetic data has been generated in the figshare brain dataset for the first time.Keywords
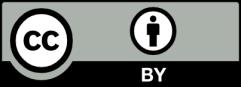