Open Access
ARTICLE
A Credit Card Fraud Model Prediction Method Based on Penalty Factor Optimization AWTadaboost
1 College of Computer and Communication, Hunan Institute of Engineering, Xiangtan, 411104, China
2 College of Computational Science and Electronics, Hunan Institute of Engineering, Xiangtan, 411104, China
* Corresponding Authors: Wang Ning. Email: ; Siliang Chen. Email:
Computers, Materials & Continua 2023, 74(3), 5951-5965. https://doi.org/10.32604/cmc.2023.035558
Received 25 August 2022; Accepted 26 October 2022; Issue published 28 December 2022
Abstract
With the popularity of online payment, how to perform credit card fraud detection more accurately has also become a hot issue. And with the emergence of the adaptive boosting algorithm (Adaboost), credit card fraud detection has started to use this method in large numbers, but the traditional Adaboost is prone to overfitting in the presence of noisy samples. Therefore, in order to alleviate this phenomenon, this paper proposes a new idea: using the number of consecutive sample misclassifications to determine the noisy samples, while constructing a penalty factor to reconstruct the sample weight assignment. Firstly, the theoretical analysis shows that the traditional Adaboost method is overfitting in a noisy training set, which leads to the degradation of classification accuracy. To this end, the penalty factor constructed by the number of consecutive misclassifications of samples is used to reconstruct the sample weight assignment to prevent the classifier from over-focusing on noisy samples, and its reasonableness is demonstrated. Then, by comparing the penalty strength of the three different penalty factors proposed in this paper, a more reasonable penalty factor is selected. Meanwhile, in order to make the constructed model more in line with the actual requirements on training time consumption, the Adaboost algorithm with adaptive weight trimming (AWTAdaboost) is used in this paper, so the penalty factor-based AWTAdaboost (PF_AWTAdaboost) is finally obtained. Finally, PF_AWTAdaboost is experimentally validated against other traditional machine learning algorithms on credit card fraud datasets and other datasets. The results show that the PF_AWTAdaboost method has better performance, including detection accuracy, model recall and robustness, than other methods on the credit card fraud dataset. And the PF_AWTAdaboost method also shows excellent generalization performance on other datasets. From the experimental results, it is shown that the PF_AWTAdaboost algorithm has better classification performance.Keywords
Cite This Article
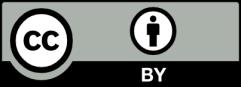
This work is licensed under a Creative Commons Attribution 4.0 International License , which permits unrestricted use, distribution, and reproduction in any medium, provided the original work is properly cited.