Open Access
ARTICLE
Drift Detection Method Using Distance Measures and Windowing Schemes for Sentiment Classification
1 School of Computing, Univerti Teknologi Malaysia, Johor, 81310, Malaysia
2 UTM Big Data Centre, Ibnu Sina Institute for Scientific and Industrial Research, Universiti Teknologi Malaysia, Johor, 81310, Malaysia
3 Ibrahim Badamasi Babangida University, Lapai, PMB 11, Niger Steate, Nigeria
4 UNITAR Graduate School, UNITAR International University, Tierra Crest, Jln SS6//3, Petaling Jaya, 47301, Selangor, Malaysia
5 Department of Information Systems-Girls Section, King Khalid University, Mahayil, 62529, Saudi Arabia
6 Department of Mathematics-Girls Section, King Khalid University, Mahayil, 62529, Saudi Arabia
* Corresponding Author: Idris Rabiu. Email:
Computers, Materials & Continua 2023, 74(3), 6001-6017. https://doi.org/10.32604/cmc.2023.035221
Received 12 August 2022; Accepted 12 November 2022; Issue published 28 December 2022
Abstract
Textual data streams have been extensively used in practical applications where consumers of online products have expressed their views regarding online products. Due to changes in data distribution, commonly referred to as concept drift, mining this data stream is a challenging problem for researchers. The majority of the existing drift detection techniques are based on classification errors, which have higher probabilities of false-positive or missed detections. To improve classification accuracy, there is a need to develop more intuitive detection techniques that can identify a great number of drifts in the data streams. This paper presents an adaptive unsupervised learning technique, an ensemble classifier based on drift detection for opinion mining and sentiment classification. To improve classification performance, this approach uses four different dissimilarity measures to determine the degree of concept drifts in the data stream. Whenever a drift is detected, the proposed method builds and adds a new classifier to the ensemble. To add a new classifier, the total number of classifiers in the ensemble is first checked if the limit is exceeded before the classifier with the least weight is removed from the ensemble. To this end, a weighting mechanism is used to calculate the weight of each classifier, which decides the contribution of each classifier in the final classification results. Several experiments were conducted on real-world datasets and the results were evaluated on the false positive rate, miss detection rate, and accuracy measures. The proposed method is also compared with the state-of-the-art methods, which include DDM, EDDM, and PageHinkley with support vector machine (SVM) and Naïve Bayes classifiers that are frequently used in concept drift detection studies. In all cases, the results show the efficiency of our proposed method.Keywords
Cite This Article
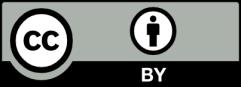
This work is licensed under a Creative Commons Attribution 4.0 International License , which permits unrestricted use, distribution, and reproduction in any medium, provided the original work is properly cited.