Open Access
ARTICLE
Bridge Crack Segmentation Method Based on Parallel Attention Mechanism and Multi-Scale Features Fusion
1 College of Civil Engineering, Changsha University of Science and Technology, Changsha, 410114, China
2 College of Computer & Communication Engineering, Changsha University of Science and Technology, Changsha, 410114, China
3 China Construction Fifth Engineering Bureau Co., Ltd., Changsha, 410004, China
* Corresponding Author: Xinli Song. Email:
Computers, Materials & Continua 2023, 74(3), 6485-6503. https://doi.org/10.32604/cmc.2023.035165
Received 09 August 2022; Accepted 24 October 2022; Issue published 28 December 2022
Abstract
Regular inspection of bridge cracks is crucial to bridge maintenance and repair. The traditional manual crack detection methods are time-consuming, dangerous and subjective. At the same time, for the existing mainstream vision-based automatic crack detection algorithms, it is challenging to detect fine cracks and balance the detection accuracy and speed. Therefore, this paper proposes a new bridge crack segmentation method based on parallel attention mechanism and multi-scale features fusion on top of the DeeplabV3+ network framework. First, the improved lightweight MobileNet-v2 network and dilated separable convolution are integrated into the original DeeplabV3+ network to improve the original backbone network Xception and atrous spatial pyramid pooling (ASPP) module, respectively, dramatically reducing the number of parameters in the network and accelerates the training and prediction speed of the model. Moreover, we introduce the parallel attention mechanism into the encoding and decoding stages. The attention to the crack regions can be enhanced from the aspects of both channel and spatial parts and significantly suppress the interference of various noises. Finally, we further improve the detection performance of the model for fine cracks by introducing a multi-scale features fusion module. Our research results are validated on the self-made dataset. The experiments show that our method is more accurate than other methods. Its intersection of union (IoU) and F1-score (F1) are increased to 77.96% and 87.57%, respectively. In addition, the number of parameters is only 4.10 M, which is much smaller than the original network; also, the frames per second (FPS) is increased to 15 frames/s. The results prove that the proposed method fits well the requirements of rapid and accurate detection of bridge cracks and is superior to other methods.Keywords
Cite This Article
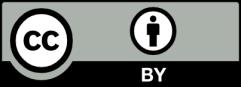
This work is licensed under a Creative Commons Attribution 4.0 International License , which permits unrestricted use, distribution, and reproduction in any medium, provided the original work is properly cited.