Open Access
ARTICLE
Topic Modelling and Sentimental Analysis of Students’ Reviews
1 Information and Computer Science Department, King Fahd University of Petroleum and Minerals, 31261, Kingdom Saudi Arabia
2 Computer Science Department, School of Computing and Digital Technology, Birmingham City University, Birmingham, B55JU, United Kingdom
* Corresponding Author: Rasheed Mohammad Nassr. Email:
Computers, Materials & Continua 2023, 74(3), 6835-6848. https://doi.org/10.32604/cmc.2023.034987
Received 02 August 2022; Accepted 26 October 2022; Issue published 28 December 2022
Abstract
Globally, educational institutions have reported a dramatic shift to online learning in an effort to contain the COVID-19 pandemic. The fundamental concern has been the continuance of education. As a result, several novel solutions have been developed to address technical and pedagogical issues. However, these were not the only difficulties that students faced. The implemented solutions involved the operation of the educational process with less regard for students’ changing circumstances, which obliged them to study from home. Students should be asked to provide a full list of their concerns. As a result, student reflections, including those from Saudi Arabia, have been analysed to identify obstacles encountered during the COVID-19 pandemic. However, most of the analyses relied on closed-ended questions, which limited student involvement. To delve into students’ responses, this study used open-ended questions, a qualitative method (content analysis), a quantitative method (topic modelling), and a sentimental analysis. This study also looked at students’ emotional states during and after the COVID-19 pandemic. In terms of determining trends in students’ input, the results showed that quantitative and qualitative methods produced similar outcomes. Students had unfavourable sentiments about studying during COVID-19 and positive sentiments about the face-to-face study. Furthermore, topic modelling has revealed that the majority of difficulties are more related to the environment (home) and social life. Students were less accepting of online learning. As a result, it is possible to conclude that face-to-face study still attracts students and provides benefits that online study cannot, such as social interaction and effective eye-to-eye communication.Keywords
On the 3rd of March, 2020, the first COVID-19 case in Saudi Arabia was reported [1]. To combat the spread of COVID-19 in Saudi Arabia, educational institutes shifted to complete online learning on the 8th of March, 2020 [2]. Many studies [2–9] have reported on Saudi Arabian universities’ experiences with full-fledged online learning. The early weeks of transformation saw technological and pedagogical challenges, which were overcome using a variety of techniques. While it is theoretically possible to deliver instruction to students online, their physical academic life is irreplaceable. As a result, students have experienced impediments with such comprehensive changes [5]. Saudi students are socialised [10] and prefer to acquire academic instruction on campus [5]. There has been criticism [11] that, while lecturers have received relevant training to become fully prepared, little attention has been paid to students; this may provide some context for student complaints.
This study uses Artificial Intelligence (AI) tools, specifically Natural Language Processing (NLP), to analyse students’ input in order to identify themes (reasons, preferences, and challenges) that better explain their satisfaction or dissatisfaction with online and face-to-face learning. Topic modelling was used in this study to assess the proportion of the probable list of concerns encountered by students. Since there was no topic modelling to precisely indicate the number of themes in the students’ reviews, this study relied on content analysis and Grid-search to determine the possible number of themes. To produce a more accurate representation, topic modelling has been combined with additional processes. Unlike many other studies concerned with students’ input during and/or after COVID-19 in the Saudi Arabian context, this study collected data at several periods in time and used mixed approaches to analyse the data, which may produce more consistent results.
The closure of educational facilities in Saudi Arabia as a result of the COVID-19 pandemic, as well as the sudden change from face-to-face learning to online learning, has created a number of issues [8]. According to one source, due to the sole usage of online learning during the lockdown, the Saudi education system encountered serious obstacles, including students’ psychological preparation and motivation [9]. There is one worldwide issue; for example, the Japanese education community prefers the current function of online learning (i.e., working alongside face-to-face) and has impeded its spread owing to the COVID-19 pandemic [12]. Similarly, multiple studies [13] suggest that educational communities prefer blended-learning models.
It has been stated that higher education institutions experienced a number of challenges during the COVID-19 pandemic. For example, students’ motivation to continue their studies online during lockdown was moderate, and the educational community played a smaller role in increasing students’ acceptance of online learning as a distinct educational channel during lockdown [12]. Furthermore, students have expressed concerns regarding the reliability of their internet connection when studying online [12,14].
Students encountered challenges in terms of educational flexibility, computer usage, communication with classmates and lecturers, and a lack of human interaction in the Saudi setting of online learning during lockdown [15]. Similar findings indicated that students were uninterested in a full transition to online instruction [16,17]. In reality, significant challenges were anticipated for students in Saudi Arabia during lockdown [16] as a result of a sudden shift to online learning, which had previously been optional during face-to-face study sessions [6]. However, studies in the Saudi context (1) used specific scales that are typically used in regular situations rather than specific situations such as a lockdown; (2) used small samples of students ([4] surveyed 25; [18] surveyed 68; [15] surveyed 106; [16] surveyed 174; [8] surveyed 60); and (3) used closed-ended questions (e.g., [2,4,5,15,17–22]). These preceding criteria may limit the identification of all conceivable issues that students wish to communicate. Furthermore, employing open-ended questions and analysing data using AI algorithms may disclose more details than conventional statistical procedures or human observations.
In the post-COVID-19 period, universities increased their digital transformation of education [12,19,20,23]. Furthermore, it has been suggested that conventional online teaching materials, such as lengthy records and slides, be replaced with short and interesting videos [23]. During lockdown, however, a considerable proportion of students indicated a preference for face-to-face instructional approaches [14]. As a result, blended learning has been identified as the best post-COVID-19 learning environment [13,24–26]. Blended learning, with a higher emphasis on face-to-face interactions with students, was recommended by medical researchers [27]. On the other hand, lockdowns produced by the COVID-19 pandemic have impacted students’ perceptions of education, and noticeable modifications have been discovered [26].
According to studies, the concerns that arose during Saudi lockdowns were mostly technical and academic in nature. This does not entail that they were the only problems encountered. Due to the nature of the closed-ended questions, students’ responses were limited to the scope provided by the researchers. As a result, concerns unrelated to academic and technological aspects have been neglected. This study discovered that by allowing students to express themselves freely and employing AI approaches, all potential issues could be identified. The use of AI has led to educational shifts during lockdown by analysing students’ performance and engagement while learning online [12]. Nonetheless, insufficient studies have used AI, particularly NLP, with Saudi students’ input during and after COVID-19. This study combines NLP approaches (topic modelling and sentimental analysis) to examine Saudi students’ feedback on their experiences with online learning during lockdowns, as well as their experiences and sentimental status after returning to campus. In contrast to content analysis, which has been used in many other studies related to the Saudi context, using topic modelling and sentimental analysis reduces time in terms of discovering patterns and emotions from students’ input. In other words, when combined with text analysis and Grid-search, topic modelling can produce a more specific list of themes and their corresponding weights.
Since the input to this study has no specific assumptions, the use of open-ended questions is appropriate for allowing students to discuss any and all concerns that may arise. This study employed open-ended questionnaires [28,29] to elicit students’ perspectives on learning during lockdown and after COVID-19. Open-ended questions were chosen because their answers are more reliable and useful than those of closed-ended questions [30].
Texts were mostly analysed qualitatively as the only input for this study, which was accomplished by content analysis. However, quantitative approaches, such as those offered by AI, can analyse texts and provide statistics to back up the findings of qualitative approaches. Following that, this study used quantitative and qualitative methods to record students’ opinions of higher education during COVID-19 and after lockdowns were lifted. In the social, behavioural, and educational sciences, the operational approach entails gathering and combining quantitative and qualitative data on a regular basis [24]. Subsequently, the analysis was done in three steps to capture the themes. The first step used a qualitative method called content analysis, which is a common methodology for analysing texts. There are three approaches to content analysis: conventional, directed, and summative [30]. Because of the nature of this study, which had no specific theory as a foundation, the conventional approach was utilised to analyse the results. The results of content analysis were used to confirm the findings of topic modelling. The second step was a quantitative approach based on topic modelling, specifically Latent Dirichlet Allocation (LDA). The third step was to conduct sentimental analysis and emotion recognition to determine the sentimental status and emotions connected with themes among students.
The significance and reliability of this study’s methodology are demonstrated in the following instances: (1) In May 2020, while students were in the midst of a lockdown, data collection took place in Saudi Arabia (among 406 respondents) to capture students’ perceptions of online learning and their sentimental status; and (2) Two years later, when universities reopened for face-to-face studies/communication, specifically in April 2022, data collection took place in Saudi Arabia (among 220 respondents) asking the same questions that had been asked in 2020, as well as others (online, blended, face-to-face). This type of data collection was distinct from those utilised in research such as [26], which inquired about students’ impressions of educational modes before and after the COVID-19. Comparing the sentimental status of students during the peak of COVID-19 to the sentimental status collected throughout this method’s use in prior research reveals a possible disadvantage. In addition, a four-week gap between the questionnaires (during COVID-19), as implemented by [31], did not reveal a statistically significant difference. As a result, the outcomes of this study should be more credible, given that the students were in the midst of a lockdown and faced genuine academic obstacles. They returned to campus only after a lengthy lockdown.
The content analysis approach [32] was used in this paper, and the data was structured accordingly. The conventional approach’s principles state that “coding categories are derived directly from the text data” [33]. The conventional approach has the advantage of gathering information directly from participants without the imposition of predefined categories or theories [33]. As a result, before coding, answers should be read several times to gain a sense of the overall circumstance [32]. The responses are then classified and coded, and a pattern is established [34]. The coding was designed to gather themes from students’ input based on earlier studies, the current condition following lockdown, and, eventually, the contrasts between studying during and after COVID-19. In the initial round of screening, additional codes with probable relations to the themes were established. The objective was to capture the basic features because the detailed characteristics were extensive and may have limited the capacity to generate a concise and concentrated list as in previous studies. Code categories were created based on the codes retrieved to cluster together several codes that belong together. Intercoder reliability was determined using a percentage agreement for all codes. A second author independently coded 25% of the examples, using the coding book for this purpose. For all codes, the percentage agreement was greater than 95%.
The following are the topics (themes) found in students’ inputs:
(1) Students’ inputs about studying online during lockdown (early 2020): In terms of topics in students’ inputs, the code categories were (a) limited internet access, (b) limited and unstable communication with lecturers and classmates, (c) unsuitable environment of study, (d) limited access to resources, and (e) no issues reported (Table 1).
(2) Students’ inputs about studying online during lockdown (two-year reporting ~2022): The analyses of the answers to the same questions but asked after 20 months have revealed four main topics: (a) insufficient internet access; (b) communication difficulties; (c) an unsuitable environment; and (d) a lack of human interaction. When compared to the number of responses to the same questions in 2020, the number of students who responded “no issues” in 2022 was rather low. Owing to this situation, the topic “no issues reported” found in the previous step was considered marginal and was not reported as a main topic.
(3) Students’ inputs regarding returning to the face-to-face mode of education after lockdown: Based on the content analysis, there were four main categories: (a) understanding; (b) communication (with professors and classmates); (c) socializing; and (d) discipline.
(4) Students’ inputs regarding the advantages of using online learning during COVID-19: Four topics were found: (a) time management (save time on transportation); (b) replaying recorded lectures (for more understanding); (c) more time to relax; and (d) flexibility to learn (anywhere, any time).
Manually analysing a large number of textual inputs using content analysis is a difficult endeavour. This could be overcome with reasonable accuracy by applying AI approaches such as topic modelling. The technique of “topic modelling” is used to extract hidden topics from massive publications [35]. It compiles a corpus of texts into a cohesive set of topics defined by their relationship to each document and each word within the documents [36]. Word clouds (Figs. 1–4) have been utilised with answers to provide a basic and broad overview of the themes. A word cloud’s output is simply word frequency, but it emphasises the crucial topics. In Fig. 1, for example, students commonly used terminology relating to the internet and communication to describe the difficulties encountered during lockdown. Meanwhile, Fig. 4 depicts the frequent usage of phrases associated with focus, ease of communication, and social life in face-to-face settings after COVID-19.
Figure 1: Word cloud for terms used by 406 students to describe their experience with online learning during lockdown
Figure 2: Word cloud for terms used by 220 students to describe their experience with online learning during lockdown after two years
Figure 3: Word cloud for terms used by 220 students to describe the positive aspect of studying online during lockdown
Figure 4: Word cloud for terms used by 220 students to describe their face-to-face experiences after lockdown
The prominent Latent Dirichlet Allocation (LDA) algorithm was used for more precise topic modelling [37]. LDA is widely used and has previously been used successfully to good reviews [35,38–40]. The current study concentrated on terms that characterise each issue using the β distribution value (the lower the value, the stronger the relationship). LDA, on the other hand, necessitates a lot of themes [41]. This study attempted to avoid predicting the number of topics in advance. A topic number or/and a cyclic process were assigned to half of the terms studied [42] until the model was tuned to produce the best results possible [38,43,44].
As a result, using AI approaches, this study sought the optimal number of themes. Grid-search is one of them, and it has been utilised with tuning parameters (as shown in Fig. 5) to discover the ideal LDA model with the best learning rate and number of topics. According to the findings, the optimal number of subjects for students’ contributions about their study experience during COVID-19 in early 2020 was five, which corresponded to the number set in the content analysis. The other values were Model Log Likelihood Score: −489.69, and Model Perplexity: 63.18. Table 1 contains additional information about this.
Figure 5: The optimum number of topics found in students’ inputs during the early weeks (in 2020) of COVID-19, according to Grid-search for LDA
An analysis of the LDA model results was achieved using pyLDAvis, a tool that visualises the topics predicted by LDA [45]. pyLDAvis helps in interpreting the topics and their associated terms. The topics suggested after using content analysis were compared to the number of topics proposed by LDA and visualised by pyLDAvis (Table 1). Moreover, pyLDAvis was used to assign a title (generated using content analysis) to each topic predicted by LDA, based on the top most frequent terms obtained after using different values of the λ parameter. pyLDAvis assists in giving the percentage of every topic in the documents (Table 1). For instance, the topics in students’ inputs regarding their experience with online learning in early 2020 were: Topic#1 presented 25.7% of the dominating topic based on the frequent words, with the given title “limited internet access.” Topic#2 presented 23.7% of the second dominating topic and was assigned the title “unsuitable environment of study.” The last three topics were: Topic#3 (21.6%)—“limited and unstable communication with lecturers and classmates”; Topic#4 (17.1%)—“no issues reported”; and Topic#5 (11.9%)—“limited access to resources”.
The prediction of the number of topics varied between topic modelling (LDA) and content analysis (human) (Table 1). Humans can recognise themes based on semantic meaning rather than word frequency and neighbouring words, which LDA does not allow. However, topic modelling can assist humans in assigning titles to themes based on word frequency and determining the percentage of each topic in the documents. Furthermore, LDA can help humans determine the degree of proximity or overlap between topics.
Sentiment analysis is a data mining mechanism employed to measure people’s emotional polarity (positive and negative) towards a specific topic [46,47]. The unsupervised machine learning technique (TextBlob) [35,48] was used to map the polarity scores to students’ inputs. This took place after conducting pre-processing to clean the students’ inputs and remove non-essential words. Python’s NLP toolkit was used for pre-processing data: (1) the students’ inputs were lower-cased and punctuation removed; (2) stemming and lemmatisation were performed to clean the students’ inputs; and stop words were removed. The sentiment score is generally divided into three categories: positive (Polarity_Score > 0), neutral (Polarity_Score = 0), and negative (Polarity_Score < 0) [35,48].
The sentiment analysis of 406 student inputs using TextBlob (dataset gathered in May 2020) found that positivity was reflected in 17% of the inputs, negativity in 78%, and neutrality in 5% of the inputs. Text2emotion, a Python tool, was used to discover the emotions inherent in the text (Text2emotion recognises happiness, anger, sadness, surprise, and fear) [49,50]. The findings suggest that students’ inputs during COVID-19 were dominated by negative emotions (sadness and fear) (Fig. 6). The sentiment analysis of students’ inputs describing their experience after returning to face-to-face study revealed that positivity dominated, with 68% of responses being positive.
Figure 6: Emotions recognised in students’ inputs
The positivity shown in students’ responses after returning for face-to-face study has been reflected in their choice of face-to-face learning. Among the total number of students, 66% preferred face-to-face education, while only 19% chose the online type of study, which they experienced during COVID-19 (Fig. 7).
Figure 7: Students’ learning mode preferences after returning to campuses
Open-ended questions that allow students to express themselves may provide additional insight into their preferences as well as their negative or positive perspectives. Content analysis can provide detailed descriptions of topics and subtopics. However, topic modelling can only assign topics to clusters of terms that are adjacent. LDA is a Principle Component Analysis (PCA) technique that combines as many terms as feasible into a small number of classes (factors) to minimise dimensionality and make data easier to analyse. The current study discovered that topic modelling is more practical for analysing vast volumes of data; LDA’s accuracy is lower than that of content analysis. Nonetheless, LDA was rendered less accurate in this study because students chose short phrases and few words in their input, limiting LDA’s ability to detect diversity in the data and offer an accurate number of themes. The quantitative and qualitative methodologies employed in this study resulted in an integrated solution that overcomes the shortcomings of each (content analysis and topic modelling). Content analysis cannot objectively weigh and provide a percentage for every topic in the text, but it can reliably quantify the number of themes in the text. Subject modelling, on the other hand, can empirically weigh every subject but cannot calculate the number of topics in texts (documents), particularly when they are brief and include almost identical phrases or terms.
Aside from technical aspects, the findings found that, in support of their choice for the face-to-face approach, students reported being more disciplined when they attend classes on a daily basis. Communication with instructors and peers was also noted to be easy and fruitful. In comparison to the online environment, students claimed that they needed less time to absorb lessons. The labs, with their one-on-one approach, were far more convenient and practical. This issue in particular was described negatively during online learning, as students struggled to complete their practical assignments. Finally, students reported that the face-to-face approach’s social life benefits were unrivalled. However, students recognised the benefits of online learning. This study discovered the following benefits: time management, study flexibility, and time and effort savings. These findings are consistent with those reported in [2], with the primary distinction that this study employed open-ended questions (free-style writing), whereas [2] used a long list of questions that had to be responded to using a scale of values (i.e., choices of strongly agree, agree, strongly disagree, or disagree).
This study’s findings revealed that students’ preferences for face-to-face study sessions, particularly after only experiencing online study sessions, agree less with the interpretations obtained in [13] and the findings of [19] and [51]. The findings of this study, however, are consistent with the conclusions reached by [4] and [13] about the relevance of students’ social interaction in the face-to-face learning approach. According to the findings of this study, students’ enthusiasm to return to face-to-face learning (during lockdown and after COVID-19) was one of the significant topics detected in students’ input, which is consistent with [2,4,5]. This means that physical presence on campuses and socialisation are critical, because two years of solely online learning have not boosted interaction and intimacy among Saudi students.
This study’s content analysis and topic modelling with students’ input during COVID-19, as well as the sole usage of online systems, resulted in five average topics. These five items are identical to the four topics mentioned by [8] (educational impact, time management, challenges encountered, and future preferences). However, there are significant disparities in terms of sentimental status. The sentimentality tendency of large groups of participants in the present study (406 students during COVID-19 and 220 students after returning to face-to-face education) was negative, and they desired a return to face-to-face education. However, sentimentality was positive among 20 medical students (Unaizah College of Medicine and Medical Sciences, Saudi Arabia) interviewed by [8] and 221 students (Prince Sattam bin Abdulaziz University, Saudi Arabia) surveyed by [22], who decided to continue their studies online. These discrepancies are significant and may reveal distinct characteristics of the two populations studied (Unaizah College and Prince Sattam bin Abdulaziz University, Saudi Arabia). On the other hand, the lack of motivation to study from home in this study was also consistent with [21], having been considerably and negatively influenced by many other factors owing to lockdown, such as workload (number of assignments) and insufficient technological support. There were numerous similarities between the findings of this study and the findings of [18] in terms of students’ inputs (in May 2020 and April 2022) about studying online during lockdown. The similarities include technical issues, less human engagement, and an inadequate atmosphere, among other things.
Finally, in contrast to the findings of [13,24–26], which indicate a preference for a blended form of learning, the majority of participants in this study (during and after COVID-19) preferred the face-to-face approach. This could be due to the fact that, prior to the COVID-19 outbreak, the online learning system in Saudi Arabian universities was optional [6], and was mostly used to transmit educational materials and accept student submissions rather than to conduct lectures. As a result, Saudi students prefer face-to-face education [5], which allows for social interactions and discussion with peers and lecturers. This is shown by the fact that this study used content analysis and topic modelling.
The abrupt transition to exclusive online learning was difficult for Saudi students, but it appears that after two years of exclusive use of online learning methods, their preferences for face-to-face learning have not changed. Students’ primary reason for their preferences is that they value the social life on campus, which includes human interactions with peers and lecturers. Furthermore, understanding lessons in a face-to-face context, having the drive to study, and practising in labs are far more practical than in an online learning environment. This could imply that face-to-face learning will continue to play an important role in future education. Furthermore, as demonstrated by the findings of this study, a face-to-face educational setting is not only suitable for learning but also it is an avenue for students to become motivated, engage with other students and lecturers, and participate in a variety of activities. Finally, this study discovered that topic modelling is a method for rapidly categorising data and determining the primary themes described or mentioned in the data. When topic modelling is combined with content analysis, its quality in terms of assigning titles to themes discovered by topic modelling can be increased. However, content analysis is a time-consuming manual procedure. Nonetheless, it is preferable to rely solely on LDA frequencies. Finally, the findings may suggest that university campuses are a place where students practise academic life in addition to education, not just for Saudi students but also for students all over the world.
Acknowledgement: The authors would like to thank the support provided by King Fahd University of Petroleum and Minerals, KSA.
Funding Statement: The authors received no specific funding for this study.
Conflicts of Interest: The authors have no conflicts of interest to report regarding the present study.
References
1. M. Hassounah, H. Raheel and M. Alhefzi, “Digital response during the COVID-19 pandemic in Saudi Arabia,” Journal of Medical Internet Research, vol. 22, no. 9, pp. e19338, 2020. https://dx.doi.org/10.2196/19338. [Google Scholar]
2. E. Al Zahrani, Y. Alnaam, S. Alrabeeah, D. Aldossary, L. Al-Jamea et al., “E- learning experience of the medical profession’s college students during COVID-19 pandemic in Saudi Arabia,” BMC Medical Education, vol. 21, no. 443, pp. 482, 2021. https://doi.org/10.1186/s12909-021-02860-z. [Google Scholar]
3. H. Abdulrahim and F. Mabrouk, “COVID-19 and the digital transformation of saudi higher education,” Asian Journal of Distance Education, vol. 15, no. 1, pp. 291–306, 2020. [Google Scholar]
4. H. Al-Nofaie, “Saudi university students’ perceptions towards virtual education during COVID-19 pandemic: A case study of language learning via Blackboard,” Arab World English Journal (AWEJ), vol. 11, no. 3, pp. 4–20, 2020. https://dx.doi.org/10.24093/awej/vol11no3.1. [Google Scholar]
5. A. Al Shlowiy, “Tracking Saudi EFL students’ reflections of online learning during coronavirus: Different rounds,” Frontiers in Education, no. 6, pp. 770786, 2021. https://dx.doi.org/10.3389/feduc.2021.770786. [Google Scholar]
6. Z. Amri and N. Alasmari, “Self-efficacy of Saudi English majors after the emergent transition to online learning and online assessment during the COVID-19 pandemic,” International Journal of Higher Education, vol. 10, no. 3, pp. 127, 2021. https://dx.doi.org/10.5430/ijhe.v10n3p127. [Google Scholar]
7. C. Barhoumi, A. Alsaysi and S. Essid, “Diagnosis of the effectiveness of the e-learning solutions adopted in Saudi higher education during the COVID-19 pandemic,” Online Information Review, vol. 46, no. 6, pp. 1132–1151, 2022. https://doi.org/10.1108/OIR-02-2021-0078. [Google Scholar]
8. R. Khalil, A. Mansour, W. Fadda, M. Aldamegh, A. Al-Nafeesah et al., “The sudden transition to synchronized online learning during the COVID-19 pandemic in Saudi Arabia: A qualitative study exploring medical students’ perspectives,” BMC Medical Education, vol. 20, no. 285, pp. 14, 2020. https://doi.org/10.1186/s12909-020-02208-z. [Google Scholar]
9. A. Mann, M. Schwabe, P. Fraser, G. Fülöp and G. Ansah, “How the COVID-19 pandemic is changing education: A perspective from Saudi Arabia,” Organisation for Economic Co-operation and Development, 2020. [Google Scholar]
10. N. Razek and S. Coyner, “Cultural impacts on Saudi Students at a Mid-western American University. Counselor Education and Human Services Faculty Publications,” 2013. [Online]. Available: https://ecommons.udayton.edu/edc_fac_pub/4. [Google Scholar]
11. L. Neuwirth, S. Jović and B. Runi, “Reimagining higher education during and post-COVID-19: Challenges and opportunities,” Journal of Adult and Continuing Education, vol. 27, no. 2, 2020. https://doi.org/10.1177/1477971420947738. [Google Scholar]
12. B. Kang, “How the COVID-19 pandemic is reshaping the education service,” In: J. Lee and S. Han, H (Eds.The Future of Service Post-COVID-19 Pandemic, Volume 1, Rapid Adoption of Digital Service Technology, vol. 1, Springer, Chapter 2, pp. 15–32, 2021. [Google Scholar]
13. D. Turnbull, R. Chugh and J. Luck, “Transitioning to e‐learning during the COVID‐19 pandemic: How have higher education institutions responded to the challenge?,” Education and Information Technologies, vol. 26, no. 26, pp. 6401–6419, 2021. https://doi.org/10.1007/s10639-021-10633-w. [Google Scholar]
14. R. Nassr, A. Aborujilah, D. Aldossary and A. Aldossary, “Understanding education difficulty during COVID-19 lockdown: Reports on Malaysian university students’ experience,” IEEE Access, vol. 8, pp. 186939–186950, 2020. https://doi.org/10.1109/ACCESS.2020.3029967. [Google Scholar]
15. I. Ebaid, “Accounting students’ perceptions on e-learning during the COVID--19 pandemic: Preliminary evidence from Saudi Arabia,” Journal of Management and Business Education, vol. 3, no. 3, pp. 236–249, 2020. [Google Scholar]
16. R. Al-Jarf, “Distance learning and undergraduate Saudi students’ agency during the COVID-19 pandemic,” Bulletin of the Transylvania University of Braşov, Series IV: Philology & Cultural Studies, vol. 13, no. 62, 2020. https://doi.org/10.31926/but.pcs.2020.62.13.2.4. [Google Scholar]
17. M. Tanveer, A. Bhaumik, S. Hassan and I. Ul Haq, “COVID-19 pandemic, outbreak educational sector and students online learning in Saudi Arabia,” Journal of Entrepreneurship Education, vol. 23, no. 3, 2020. [Google Scholar]
18. A. Al-Rasheed, “The challenges faced by undergraduate women during the COVID-19 pandemic in Saudi Arabia,” Hindawi Education Research International, vol. 2021, no. 4, pp. 1–10, 2021. https://doi.org/10.1155/2021/8841997. [Google Scholar]
19. A. Alammary, M. Alshaikh and A. Alhogail, “The impact of the COVID-19 pandemic on the adoption of e-learning among academics in Saudi Arabia,” Behaviour & Information Technology, 2021. https://doi.org/10.1080/0144929X.2021.1973106. [Google Scholar]
20. M. Alashwal, “Assessing online teaching in higher education amid the COVID-19 pandemic: A case study from Saudi Arabia,” in Proc. ConScienS Conf., pp. 68–72, 2020. https://doi.org/10.5281/zenodo.4058780. [Google Scholar]
21. S. Hassan, F. Algahtani, R. Zrieq, B. Aldhmadi, A. Atta et al., “Academic self-perception and course satisfaction among university students taking virtual classes during the COVID-19 pandemic in the Kingdom of Saudi-Arabia (KSA),” Education Sciences, vol. 11, no. 134, pp. 134, 2021. https://doi.org/10.3390/educsci11030134. [Google Scholar]
22. M. Karim, Rezaul and M. Hasan, “Virtual classes during covid 19 pandemic in tertiary level in Saudi Arabia: Challenges and prospects from the students’ perspective,” Asian EFL Journal Research Articles, vol. 27, no. 52020. [Google Scholar]
23. L. Ewing, “Rethinking higher education post COVID-19,” in The Future of Service Post-COVID-19 Pandemic, Volume 1, Rapid Adoption of Digital Service Technology, J. Lee, S. Han, Springer, pp. 37–53, 2021. [Google Scholar]
24. Á. Benito, K. Yenisey, K. Khanna, M. Masis, R. Monge et al., “Changes that should remain in higher education post COVID-19: A mixed-methods analysis of the experiences at three universities,” Higher Learning Research Communications, vol. 11, pp. 51–75, 2021. https://doi.org/10.18870/hlrc.v11i0.1195. [Google Scholar]
25. H. Pham and T. Ho, “Toward a ‘new normal’ with e-learning in Vietnamese higher education during the post COVID-19 pandemic,” Higher Education Research & Development, vol. 39, no. 7, pp. 1327–1331, 2020. https://doi.org/10.1080/07294360.2020.1823945. [Google Scholar]
26. A. Sharma and I. Alvi, “Evaluating pre and post covid 19 learning: An empirical study of learners’ perception in higher education,” Education and Information Technologies, vol. 26, no. 26, pp. 7015–7032, 2021. https://doi.org/10.1007/s10639-021-10521-3. [Google Scholar]
27. A. Bashir, S. Bashir, K. Rana, P. Lambert and A. Vernallis, “Post-COVID-19 adaptations; the shifts towards online learning, hybrid course delivery and the implications for biosciences courses in the higher education setting,” Frontiers in Education, vol. 6, no. 711619, pp. 362, 2021. https://doi.org/10.3389/feduc.2021.711619. [Google Scholar]
28. E. Gomez, J. Azadi and D. Magid, “Innovation born in isolation: rapid transformation of an in-person medical student radiology elective to a remote learning experience during the COVID-19 pandemic,” Academic Radiology, vol. 27, no. 9, pp. 1285–1290, 2020. https://doi.org/10.1016/j.acra.2020.06.001. [Google Scholar]
29. V. Rajhans, U. Memon, V. Patil and A. Goyal, “Impact of COVID-19 on academic activities and way forward in Indian optometry,” Journal of Optometry, vol. 13, no. 4, pp. 216–226, 2020. https://doi.org/10.1016/j.optom.2020.06.002. [Google Scholar]
30. R. Popping, “Analyzing open-ended questions by means of text analysis procedures,” in Proc. the Optimal Coding of Open-Ended Survey Data, Michigan, 2008. [Google Scholar]
31. K. Almendingen, M. Morseth, E. Gjølstad, A. Brevik and C. Tørris, “Student’s experiences with online teaching following COVID-19 lockdown: A mixed methods explorative study,” PLoS One, vol. 16, no. 8, pp. e0250378, 2021. https://doi.org/10.1371/journal.pone.0250378. [Google Scholar]
32. J. Lofland and L. Lofland, Analyzing social settings: A guide to qualitative observation and analysis. Belmont, CA, USA: Wadsworth, 1995. [Google Scholar]
33. H. Hsieh and S. E. Shannon, “Three approaches to qualitative content analysis,” Qualitative Health Research, vol. 15, no. 9, pp. 1277–1289, 2005. https://doi.org/10.1177/1049732305276687. [Google Scholar]
34. L. Grenier, “How to analyze open-ended questions in 5 steps,” Available: http://www.hotjar.com/blog/analyze-open-ended-questions. [Google Scholar]
35. M. Mujahid, E. Lee, F. Rustam, P. Washington, S. Ullah et al., “Sentiment analysis and topic modeling on tweets about online education during COVID-19,” Applied Sciences, vol. 11, no. 8438, 2021. https://doi.org/10.3390/app11188438. [Google Scholar]
36. S. Moro, R. Lopes, J. Esmerado and M. Botelho, “Service quality in airport hotel chains through the lens of online reviewers,” Journal of Retailing and Consumer Services, vol. 56, no. 2, pp. 102193, 2020. https://doi.org/10.1016/j.jretconser.2020.102193. [Google Scholar]
37. D. Blei, A. Ng and M. Jordan, “Latent dirichlet allocation,” Journal of Machine Learning Research, no. 3, pp. 993–1022, 2003. [Google Scholar]
38. A. Amado, P. Cortez, P. Rita and S. Moro, “Research trends on big data in marketing: A text mining and topic modeling based literature analysis,” European Research on Management and Business Economics, vol. 24, no. 1, pp. 1–7, 2018. https://doi.org/10.1016/j.iedeen.2017.06.002. [Google Scholar]
39. A. Calheiros, S. Moro and P. Rita, “Sentiment classification of consumer-generated online reviews using topic modeling,” Journal of Hospitality Marketing & Management, vol. 26, no. 7, pp. 675–693, 2017. https://doi.org/10.1080/19368623.2017.1310075. [Google Scholar]
40. S. Kiliç and T. Çadirci, “An evaluation of airport service experience: An identification of service improvement opportunities based on topic modeling and sentiment analysis,” Research in Transportation Business & Management, 2021. https://doi.org/10.1016/j.rtbm.2021.100744. [Google Scholar]
41. X. Yi and J. Allan, “A comparative study of utilizing topic models for information retrieval,” in Proc. the European Conf. on Information Retrieval, 2009. [Google Scholar]
42. S. Moro, P. Cortez and P. Rita, “Business intelligence in banking: A literature analysis from 2002 to 2013 using text mining and latent Dirichlet allocation,” Expert Systems with Applications, vol. 42, no. 3, pp. 1314–1324, 2015. https://doi.org/10.1016/j.eswa.2014.09.024. [Google Scholar]
43. J. Canito, P. Ramos, S. Moro and P. Rita, “Unfolding the relations between companies and technologies under the Big Data umbrella,” Computers in Industry, vol. 99, no. 1, pp. 1–8, 2018. https://doi.org/10.1016/j.compind.2018.03.018. [Google Scholar]
44. R. Sharda, D. Delen and E. Turban, Business intelligence, analytics and data science: A managerial perspective, 4th ed., London, UK: Pearson, 2017. [Google Scholar]
45. K. M. Ridhwan and C. A. Hargreaves, “Leveraging Twitter data to understand public sentiment for the COVID-19 outbreak in Singapore,” International Journal of Information Management Data Insights, no. 1, pp. 100021, 2021. https://doi.org/10.1016/j.jjimei.2021.100021. [Google Scholar]
46. A. Athar, W. H. Butt, M. W. Anwar, M. Latif and F. Azam, “Exploring the Ensemble of Classifiers for sentimental analysis - A systematic literature review,” in Int. Conf. on Machine Learning and Computing’17, Singapore, Singapore, 2017. [Google Scholar]
47. L. Martin-Domingo, J. C. Martín and G. Mandsberg, “Social media as a resource for sentiment analysis of Airport Service Quality (ASQ),” Journal of Air Transport Management, vol. 78, no. 1, pp. 106–115, 2019. https://doi.org/10.1016/j.jairtraman.2019.01.004. [Google Scholar]
48. D. Effrosynidis, A. I. Karasakalidis, G. Sylaios and A. Arampatzis, “The climate change Twitter dataset,” Expert Systems with Applications, vol. 204, no. 3, pp. 117541, 2022. https://doi.org/10.1016/j.eswa.2022.117541. [Google Scholar]
49. T. Diana, “Using sentiment analysis to reinforce learning: The case of airport community engagement,” Journal of Air Transport Management, vol. 102, no. 2, pp. 102228, 2022. https://doi.org/10.1016/j.jairtraman.2022.102228. [Google Scholar]
50. S. Paul, “Analyzing the attitude of Indian citizens during the second wave of COVID-19: A text analytics study,” International Journal of Disaster Risk Reduction, vol. 79, no. 1, pp. 103161, 2022. [Google Scholar]
51. A. Alanazi and Z. Alshaalan, “Views of faculty members on the use of e-learning in Saudi medical and health colleges during COVID-19 pandemic,” Journal of Nature and Science of Medicine, vol. 3, no. 4, 2020. https://doi.org/10.4103/JNSM.JNSM_82_20. [Google Scholar]
Cite This Article
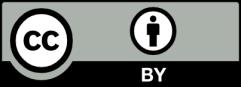