Open Access
ARTICLE
DQN-Based Proactive Trajectory Planning of UAVs in Multi-Access Edge Computing
1 School of Electronic Engineering, Beijing University of Posts and Telecommunications, Beijing, 100876, China
2 State Key Laboratory of Networking and Switching Technology, Beijing University of Posts and Telecommunications, Beijing, 100876, China
3 Department of Automation, Chongqing University of Posts and Telecommunications, Chongqing, 400065, China
* Corresponding Author: Adil Khan. Email:
Computers, Materials & Continua 2023, 74(3), 4685-4702. https://doi.org/10.32604/cmc.2023.034892
Received 31 July 2022; Accepted 22 September 2022; Issue published 28 December 2022
Abstract
The main aim of future mobile networks is to provide secure, reliable, intelligent, and seamless connectivity. It also enables mobile network operators to ensure their customer’s a better quality of service (QoS). Nowadays, Unmanned Aerial Vehicles (UAVs) are a significant part of the mobile network due to their continuously growing use in various applications. For better coverage, cost-effective, and seamless service connectivity and provisioning, UAVs have emerged as the best choice for telco operators. UAVs can be used as flying base stations, edge servers, and relay nodes in mobile networks. On the other side, Multi-access Edge Computing (MEC) technology also emerged in the 5G network to provide a better quality of experience (QoE) to users with different QoS requirements. However, UAVs in a mobile network for coverage enhancement and better QoS face several challenges such as trajectory designing, path planning, optimization, QoS assurance, mobility management, etc. The efficient and proactive path planning and optimization in a highly dynamic environment containing buildings and obstacles are challenging. So, an automated Artificial Intelligence (AI) enabled QoS-aware solution is needed for trajectory planning and optimization. Therefore, this work introduces a well-designed AI and MEC-enabled architecture for a UAVs-assisted future network. It has an efficient Deep Reinforcement Learning (DRL) algorithm for real-time and proactive trajectory planning and optimization. It also fulfills QoS-aware service provisioning. A greedy-policy approach is used to maximize the long-term reward for serving more users with QoS. Simulation results reveal the superiority of the proposed DRL mechanism for energy-efficient and QoS-aware trajectory planning over the existing models.Keywords
Cite This Article
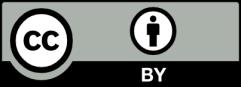
This work is licensed under a Creative Commons Attribution 4.0 International License , which permits unrestricted use, distribution, and reproduction in any medium, provided the original work is properly cited.