Open Access
ARTICLE
Application of Deep Learning to Production Forecasting in Intelligent Agricultural Product Supply Chain
1 Department of Logistics Management and Engineering, Nanning Normal University, Nanning, 530023, China
2 Guangxi Key Lab of Human-Machine Interaction and Intelligent Decision, Nanning Normal University, Nanning, 530023, China
3 School of Management and Marketing, Faculty of Business and Law, Taylor’s University, Kuala Lumpur, 47500, Malaysia
4 Graduate Department,Yunnan Normal University, Kunming, 650500, China
* Corresponding Author: Xiao Ya Ma. Email:
Computers, Materials & Continua 2023, 74(3), 6145-6159. https://doi.org/10.32604/cmc.2023.034833
Received 28 July 2022; Accepted 24 October 2022; Issue published 28 December 2022
Abstract
Production prediction is an important factor influencing the realization of an intelligent agricultural supply chain. In an Internet of Things (IoT) environment, accurate yield prediction is one of the prerequisites for achieving an efficient response in an intelligent agricultural supply chain. As an example, this study applied a conventional prediction method and deep learning prediction model to predict the yield of a characteristic regional fruit (the Shatian pomelo) in a comparative study. The root means square error (RMSE) values of regression analysis, exponential smoothing, grey prediction, grey neural network, support vector regression (SVR), and long short-term memory (LSTM) neural network methods were 53.715, 6.707, 18.440, 1.580, and 1.436, respectively. Among these, the mean square error (MSE) values of the grey neural network, SVR, and LSTM neural network methods were 2.4979, 31.652, and 2.0618, respectively; and their R values were 0.99905, 0.94, and 0.94501, respectively. The results demonstrated that the RMSE of the deep learning model is generally lower than that of a traditional prediction model, and the prediction results are more accurate. The prediction performance of the grey neural network was shown to be superior to that of SVR, and LSTM neural network, based on the comparison of parameters.Keywords
Cite This Article
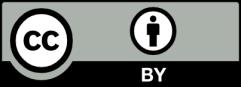
This work is licensed under a Creative Commons Attribution 4.0 International License , which permits unrestricted use, distribution, and reproduction in any medium, provided the original work is properly cited.