Open Access
ARTICLE
Xception-Fractalnet: Hybrid Deep Learning Based Multi-Class Classification of Alzheimer’s Disease
School of Computer Science and Engineering, VIT-AP University, Andhra Pradesh, 522237, India
* Corresponding Author: Battula Srinivasa Rao. Email:
Computers, Materials & Continua 2023, 74(3), 6909-6932. https://doi.org/10.32604/cmc.2023.034796
Received 27 July 2022; Accepted 26 October 2022; Issue published 28 December 2022
Abstract
Neurological disorders such as Alzheimer’s disease (AD) are very challenging to treat due to their sensitivity, technical challenges during surgery, and high expenses. The complexity of the brain structures makes it difficult to distinguish between the various brain tissues and categorize AD using conventional classification methods. Furthermore, conventional approaches take a lot of time and might not always be precise. Hence, a suitable classification framework with brain imaging may produce more accurate findings for early diagnosis of AD. Therefore in this paper, an effective hybrid Xception and Fractalnet-based deep learning framework are implemented to classify the stages of AD into five classes. Initially, a network based on Unet++ is built to segment the tissues of the brain. Then, using the segmented tissue components as input, the Xception-based deep learning technique is employed to extract high-level features. Finally, the optimized Fractalnet framework is used to categorize the disease condition using the acquired characteristics. The proposed strategy is tested on the Alzheimer’s Disease Neuroimaging Initiative (ADNI) dataset that accurately segments brain tissues with a 98.45% of dice similarity coefficient (DSC). Additionally, for the multiclass classification of AD, the suggested technique obtains an accuracy of 99.06%. Moreover, ANOVA statistical analysis is also used to evaluate if the groups are significant or not. The findings show that the suggested model outperforms various state-of-the-art methods in terms of several performance metrics.Keywords
Cite This Article
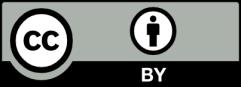