Open Access
ARTICLE
Hybrid Feature Selection Method for Predicting Alzheimer’s Disease Using Gene Expression Data
1 Department of Information Systems, Faculty of Computers and Informatics, Suez Canal University, Ismailia, 41522, Egypt
2 Faculty of Computer Science, Nahda University, Beni Suef, Egypt
* Corresponding Author: Aliaa El-Gawady. Email:
Computers, Materials & Continua 2023, 74(3), 5559-5572. https://doi.org/10.32604/cmc.2023.034734
Received 26 July 2022; Accepted 03 October 2022; Issue published 28 December 2022
Abstract
Gene expression (GE) classification is a research trend as it has been used to diagnose and prognosis many diseases. Employing machine learning (ML) in the prediction of many diseases based on GE data has been a flourishing research area. However, some diseases, like Alzheimer’s disease (AD), have not received considerable attention, probably owing to data scarcity obstacles. In this work, we shed light on the prediction of AD from GE data accurately using ML. Our approach consists of four phases: preprocessing, gene selection (GS), classification, and performance validation. In the preprocessing phase, gene columns are preprocessed identically. In the GS phase, a hybrid filtering method and embedded method are used. In the classification phase, three ML models are implemented using the bare minimum of the chosen genes obtained from the previous phase. The final phase is to validate the performance of these classifiers using different metrics. The crux of this article is to select the most informative genes from the hybrid method, and the best ML technique to predict AD using this minimal set of genes. Five different datasets are used to achieve our goal. We predict AD with impressive values for MultiLayer Perceptron (MLP) classifier which has the best performance metrics in four datasets, and the Support Vector Machine (SVM) achieves the highest performance values in only one dataset. We assessed the classifiers using seven metrics; and received impressive results, allowing for a credible performance rating. The metrics values we obtain in our study lie in the range [.97, .99] for the accuracy (Acc), [.97, .99] for F1-score, [.94, .98] for kappa index, [.97, .99] for area under curve (AUC), [.95, 1] for precision, [.98, .99] for sensitivity (recall), and [.98, 1] for specificity. With these results, the proposed approach outperforms recent interesting results. With these results, the proposed approach outperforms recent interesting results.Keywords
Cite This Article
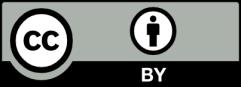