Open Access
ARTICLE
Visual News Ticker Surveillance Approach from Arabic Broadcast Streams
1 Department of Computer Science and Software Engineering, International Islamic University, Islamabad, 44000, Pakistan
2 Department of Computer Science, Quaid-i-Azam University, Islamabad, 44000, Pakistan
3 Department of Computer Science, Senior Member IEEE, University of Windsor, N9B 3P4, Canada
4 Department of Software Engineering, Foundation University Islamabad, Islamabad, 44000, Pakistan
5 Department of Electrical Engineering, Foundation University Islamabad, Islamabad, 44000, Pakistan
* Corresponding Author: Ayyaz Hussain. Email:
Computers, Materials & Continua 2023, 74(3), 6177-6193. https://doi.org/10.32604/cmc.2023.034669
Received 23 July 2022; Accepted 22 September 2022; Issue published 28 December 2022
Abstract
The news ticker is a common feature of many different news networks that display headlines and other information. News ticker recognition applications are highly valuable in e-business and news surveillance for media regulatory authorities. In this paper, we focus on the automatic Arabic Ticker Recognition system for the Al-Ekhbariya news channel. The primary emphasis of this research is on ticker recognition methods and storage schemes. To that end, the research is aimed at character-wise explicit segmentation using a semantic segmentation technique and words identification method. The proposed learning architecture considers the grouping of homogeneous-shaped classes. This incorporates linguistic taxonomy in a unified manner to address the imbalance in data distribution which leads to individual biases. Furthermore, experiments with a novel Arabic News Ticker (Al-ENT) dataset that provides accurate character-level and character components-level labeling to evaluate the effectiveness of the suggested approach. The proposed method attains 96.5%, outperforming the current state-of-the-art technique by 8.5%. The study reveals that our strategy improves the performance of low-representation correlated character classes.Keywords
Cite This Article
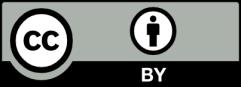
This work is licensed under a Creative Commons Attribution 4.0 International License , which permits unrestricted use, distribution, and reproduction in any medium, provided the original work is properly cited.