Open Access
ARTICLE
Research on Federated Learning Data Sharing Scheme Based on Differential Privacy
Department of Information and Communications Engineering, Nanjing Institute of Technology, Nanjing, 211167, China
* Corresponding Author: Lihong Guo. Email:
Computers, Materials & Continua 2023, 74(3), 5069-5085. https://doi.org/10.32604/cmc.2023.034571
Received 20 July 2022; Accepted 03 October 2022; Issue published 28 December 2022
Abstract
To realize data sharing, and to fully use the data value, breaking the data island between institutions to realize data collaboration has become a new sharing mode. This paper proposed a distributed data security sharing scheme based on C/S communication mode, and constructed a federated learning architecture that uses differential privacy technology to protect training parameters. Clients do not need to share local data, and they only need to upload the trained model parameters to achieve data sharing. In the process of training, a distributed parameter update mechanism is introduced. The server is mainly responsible for issuing training commands and parameters, and aggregating the local model parameters uploaded by the clients. The client mainly uses the stochastic gradient descent algorithm for gradient trimming, updates, and transmits the trained model parameters back to the server after differential processing. To test the performance of the scheme, in the application scenario where many medical institutions jointly train the disease detection system, the model is tested from multiple perspectives by taking medical data as an example. From the testing results, we can know that for this specific test dataset, when the parameters are properly configured, the lowest prediction accuracy rate is 90.261% and the highest accuracy rate is up to 94.352. It shows that the performance of the model is good. The results also show that this scheme realizes data sharing while protecting data privacy, completes accurate prediction of diseases, and has a good effect.Keywords
Cite This Article
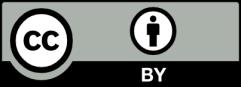
This work is licensed under a Creative Commons Attribution 4.0 International License , which permits unrestricted use, distribution, and reproduction in any medium, provided the original work is properly cited.