Open Access
ARTICLE
Deep Transfer Learning Driven Automated Fall Detection for Quality of Living of Disabled Persons
1 King Salman Center for Disability Research, Riyadh, Saudi Arabia
2 Department of Special Education, College of Education, King Saud University, Riyadh, 12372, Saudi Arabia
3 Department of Information Technology, College of Computers and Information Technology, Taif University, P.O. Box 11099, Taif, 21944, Saudi Arabia
4 Department of Computer Science, College of Science & Arts, King Khaled University, Saudi Arabia
5 Department of Computer Science, College of Sciences and Humanities-Aflaj, Prince Sattam bin Abdulaziz University, Saudi Arabia
6 Department of Computer and Self Development, Preparatory Year Deanship, Prince Sattam bin Abdulaziz University, AlKharj, Saudi Arabia
* Corresponding Author: Anwer Mustafa Hilal. Email:
Computers, Materials & Continua 2023, 74(3), 6719-6736. https://doi.org/10.32604/cmc.2023.034417
Received 16 July 2022; Accepted 03 October 2022; Issue published 28 December 2022
Abstract
Mobile communication and the Internet of Things (IoT) technologies have recently been established to collect data from human beings and the environment. The data collected can be leveraged to provide intelligent services through different applications. It is an extreme challenge to monitor disabled people from remote locations. It is because day-to-day events like falls heavily result in accidents. For a person with disabilities, a fall event is an important cause of mortality and post-traumatic complications. Therefore, detecting the fall events of disabled persons in smart homes at early stages is essential to provide the necessary support and increase their survival rate. The current study introduces a Whale Optimization Algorithm Deep Transfer Learning-Driven Automated Fall Detection (WOADTL-AFD) technique to improve the Quality of Life for persons with disabilities. The primary aim of the presented WOADTL-AFD technique is to identify and classify the fall events to help disabled individuals. To attain this, the proposed WOADTL-AFD model initially uses a modified SqueezeNet feature extractor which proficiently extracts the feature vectors. In addition, the WOADTL-AFD technique classifies the fall events using an extreme Gradient Boosting (XGBoost) classifier. In the presented WOADTL-AFD technique, the WOA approach is used to fine-tune the hyperparameters involved in the modified SqueezeNet model. The proposed WOADTL-AFD technique was experimentally validated using the benchmark datasets, and the results confirmed the superior performance of the proposed WOADTL-AFD method compared to other recent approaches.Keywords
Cite This Article
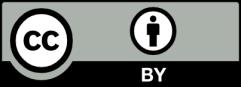
This work is licensed under a Creative Commons Attribution 4.0 International License , which permits unrestricted use, distribution, and reproduction in any medium, provided the original work is properly cited.