Open Access
ARTICLE
A Novel Wrapper-Based Optimization Algorithm for the Feature Selection and Classification
1 Computer and Information Sciences Department, Universiti Teknologi PETRONAS, Seri Iskandar, 32610, Perak, Malaysia
2 Computer Science Department, Community College, King Saud University, Riyadh, 145111, Saudi Arabia
* Corresponding Author: Noureen Talpur. Email:
Computers, Materials & Continua 2023, 74(3), 5799-5820. https://doi.org/10.32604/cmc.2023.034025
Received 04 July 2022; Accepted 03 October 2022; Issue published 28 December 2022
Abstract
Machine learning (ML) practices such as classification have played a very important role in classifying diseases in medical science. Since medical science is a sensitive field, the pre-processing of medical data requires careful handling to make quality clinical decisions. Generally, medical data is considered high-dimensional and complex data that contains many irrelevant and redundant features. These factors indirectly upset the disease prediction and classification accuracy of any ML model. To address this issue, various data pre-processing methods called Feature Selection (FS) techniques have been presented in the literature. However, the majority of such techniques frequently suffer from local minima issues due to large solution space. Thus, this study has proposed a novel wrapper-based Sand Cat Swarm Optimization (SCSO) technique as an FS approach to find optimum features from ten benchmark medical datasets. The SCSO algorithm replicates the hunting and searching strategies of the sand cat while having the advantage of avoiding local optima and finding the ideal solution with minimal control variables. Moreover, K-Nearest Neighbor (KNN) classifier was used to evaluate the effectiveness of the features identified by the proposed SCSO algorithm. The performance of the proposed SCSO algorithm was compared with six state-of-the-art and recent wrapper-based optimization algorithms using the validation metrics of classification accuracy, optimum feature size, and computational cost in seconds. The simulation results on the benchmark medical datasets revealed that the proposed SCSO-KNN approach has outperformed comparative algorithms with an average classification accuracy of 93.96% by selecting 14.2 features within 1.91 s. Additionally, the Wilcoxon rank test was used to perform the significance analysis between the proposed SCSO-KNN method and six other algorithms for a p-value less than 5.00E-02. The findings revealed that the proposed algorithm produces better outcomes with an average p-value of 1.82E-02. Moreover, potential future directions are also suggested as a result of the study’s promising findings.Keywords
Cite This Article
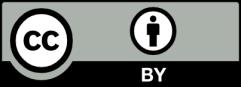
This work is licensed under a Creative Commons Attribution 4.0 International License , which permits unrestricted use, distribution, and reproduction in any medium, provided the original work is properly cited.