Open Access
ARTICLE
Energy Theft Detection in Smart Grids with Genetic Algorithm-Based Feature Selection
1 Faculty of Information Technology, University of Central Punjab, Lahore, 54590, Pakistan
2 DAAI Research Group, Department of Computing and Data Science, School of Computing and Digital Technology, Birmingham City University, Birmingham B4 7XG, UK
3 Faculty of Computer and Information Systems, Fayoum University, 63514, Egypt
4 Khaybar Applied College, Taibah University, Saudi Arabia
* Corresponding Author: Muhammad Umair. Email:
Computers, Materials & Continua 2023, 74(3), 5431-5446. https://doi.org/10.32604/cmc.2023.033884
Received 30 June 2022; Accepted 03 October 2022; Issue published 28 December 2022
Abstract
As big data, its technologies, and application continue to advance, the Smart Grid (SG) has become one of the most successful pervasive and fixed computing platforms that efficiently uses a data-driven approach and employs efficient information and communication technology (ICT) and cloud computing. As a result of the complicated architecture of cloud computing, the distinctive working of advanced metering infrastructures (AMI), and the use of sensitive data, it has become challenging to make the SG secure. Faults of the SG are categorized into two main categories, Technical Losses (TLs) and Non-Technical Losses (NTLs). Hardware failure, communication issues, ohmic losses, and energy burnout during transmission and propagation of energy are TLs. NTL’s are human-induced errors for malicious purposes such as attacking sensitive data and electricity theft, along with tampering with AMI for bill reduction by fraudulent customers. This research proposes a data-driven methodology based on principles of computational intelligence as well as big data analysis to identify fraudulent customers based on their load profile. In our proposed methodology, a hybrid Genetic Algorithm and Support Vector Machine (GA-SVM) model has been used to extract the relevant subset of feature data from a large and unsupervised public smart grid project dataset in London, UK, for theft detection. A subset of 26 out of 71 features is obtained with a classification accuracy of 96.6%, compared to studies conducted on small and limited datasets.Keywords
Cite This Article
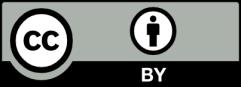
This work is licensed under a Creative Commons Attribution 4.0 International License , which permits unrestricted use, distribution, and reproduction in any medium, provided the original work is properly cited.