Open Access
ARTICLE
Transfer Learning-Based Semi-Supervised Generative Adversarial Network for Malaria Classification
1 Institute of Computing, Kohat University of Science and Technology, Kohat, 26000, K.P.K, Pakistan
2 College of Science and Engineering, Hamad bin Khalifa University, Doha, Qatar
3 Centre of Research in Data Science, Computer and Information Sciences Department, Universiti Teknologi PETRONAS, Seri Iskandar, 32610, Perak, Malaysia
* Corresponding Author: Samir Brahim Belhaouari. Email:
Computers, Materials & Continua 2023, 74(3), 6335-6349. https://doi.org/10.32604/cmc.2023.033860
Received 29 June 2022; Accepted 02 September 2022; Issue published 28 December 2022
Abstract
Malaria is a lethal disease responsible for thousands of deaths worldwide every year. Manual methods of malaria diagnosis are time-consuming that require a great deal of human expertise and efforts. Computer-based automated diagnosis of diseases is progressively becoming popular. Although deep learning models show high performance in the medical field, it demands a large volume of data for training which is hard to acquire for medical problems. Similarly, labeling of medical images can be done with the help of medical experts only. Several recent studies have utilized deep learning models to develop efficient malaria diagnostic system, which showed promising results. However, the most common problem with these models is that they need a large amount of data for training. This paper presents a computer-aided malaria diagnosis system that combines a semi-supervised generative adversarial network and transfer learning. The proposed model is trained in a semi-supervised manner and requires less training data than conventional deep learning models. Performance of the proposed model is evaluated on a publicly available dataset of blood smear images (with malaria-infected and normal class) and achieved a classification accuracy of 96.6%.Keywords
Cite This Article
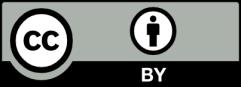
This work is licensed under a Creative Commons Attribution 4.0 International License , which permits unrestricted use, distribution, and reproduction in any medium, provided the original work is properly cited.