Open Access
ARTICLE
Using Informative Score for Instance Selection Strategy in Semi-Supervised Sentiment Classification
School of Computer Sciences, Universiti Sains Malaysia, Pulau Pinang, 11800, Malaysia
* Corresponding Author: Gan Keng Hoon. Email:
Computers, Materials & Continua 2023, 74(3), 4801-4818. https://doi.org/10.32604/cmc.2023.033752
Received 27 June 2022; Accepted 22 September 2022; Issue published 28 December 2022
Abstract
Sentiment classification is a useful tool to classify reviews about sentiments and attitudes towards a product or service. Existing studies heavily rely on sentiment classification methods that require fully annotated inputs. However, there is limited labelled text available, making the acquirement process of the fully annotated input costly and labour-intensive. Lately, semi-supervised methods emerge as they require only partially labelled input but perform comparably to supervised methods. Nevertheless, some works reported that the performance of the semi-supervised model degraded after adding unlabelled instances into training. Literature also shows that not all unlabelled instances are equally useful; thus identifying the informative unlabelled instances is beneficial in training a semi-supervised model. To achieve this, an informative score is proposed and incorporated into semi-supervised sentiment classification. The evaluation is performed on a semi-supervised method without an informative score and with an informative score. By using the informative score in the instance selection strategy to identify informative unlabelled instances, semi-supervised models perform better compared to models that do not incorporate informative scores into their training. Although the performance of semi-supervised models incorporated with an informative score is not able to surpass the supervised models, the results are still found promising as the differences in performance are subtle with a small difference of 2% to 5%, but the number of labelled instances used is greatly reduced from 100% to 40%. The best finding of the proposed instance selection strategy is achieved when incorporating an informative score with a baseline confidence score at a 0.5:0.5 ratio using only 40% labelled data.Keywords
Cite This Article
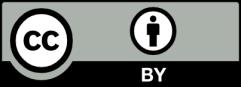
This work is licensed under a Creative Commons Attribution 4.0 International License , which permits unrestricted use, distribution, and reproduction in any medium, provided the original work is properly cited.