Open Access
REVIEW
A Review of Machine Learning Techniques in Cyberbullying Detection
1 Al-Farabi Kazakh National University, Almaty, Kazakhstan
2 International Information Technology University, Almaty, Kazakhstan
3 International University of Tourism and Hospitality, Turkistan, Kazakhstan
4 Almaty University of Power Engineering and Telecommunications, Almaty, Kazakhstan
5 Khoja Akhmet Yassawi International Kazakh-Turkish University, Turkistan, Kazakhstan
6 Korkyt Ata Kyzylorda State University, Kyzylorda, Kazakhstan
* Corresponding Author: Daniyar Sultan. Email:
Computers, Materials & Continua 2023, 74(3), 5625-5640. https://doi.org/10.32604/cmc.2023.033682
Received 24 June 2022; Accepted 23 September 2022; Issue published 28 December 2022
Abstract
Automatic identification of cyberbullying is a problem that is gaining traction, especially in the Machine Learning areas. Not only is it complicated, but it has also become a pressing necessity, considering how social media has become an integral part of adolescents’ lives and how serious the impacts of cyberbullying and online harassment can be, particularly among teenagers. This paper contains a systematic literature review of modern strategies, machine learning methods, and technical means for detecting cyberbullying and the aggressive command of an individual in the information space of the Internet. We undertake an in-depth review of 13 papers from four scientific databases. The article provides an overview of scientific literature to analyze the problem of cyberbullying detection from the point of view of machine learning and natural language processing. In this review, we consider a cyberbullying detection framework on social media platforms, which includes data collection, data processing, feature selection, feature extraction, and the application of machine learning to classify whether texts contain cyberbullying or not. This article seeks to guide future research on this topic toward a more consistent perspective with the phenomenon’s description and depiction, allowing future solutions to be more practical and effective.Keywords
The modern space of everyday communication is characterized by a new striking feature-its spread into the virtual world. If for modern adults communication skills using e-mail, instant messages and chats are an addition to the already acquired skills of live communication, then modern children and adolescents master both of these skills almost simultaneously. As for adolescents, we can say that the process of socialization is largely moving to the Internet–along with acquaintances, reference groups, the development of various social roles and norms [1]. All those communicative processes that occur in ordinary sociophysical space are “duplicated”, sometimes intensified, and sometimes compensated by virtual communication, but in any case acquire new features. Although historically virtual existence is clearly secondary in relation to the real one, one can expect a reverse impact and transfer of communicative situations and rules common on the Internet into the “real” space of communication [2].
With the development of information technologies, significant changes have taken place in the life of a modern teenager: virtual reality has appeared, in which communication and interpersonal relationships are moving to a new, unfamiliar level for them. Bullying becomes more dangerous for an individual since it can be carried out using Internet technologies [3].
For the first time, the definition of “cyberbullying” was given by Bill Belsey. In his opinion, cyberbullying is the use of information and communication technologies, for example, e-mail, mobile phone, personal Internet sites, for intentional, repeated, and hostile behavior of a person or group aimed at insulting other people [4].
Bullying on the Internet can be carried out 24 h a day, 7 days a week, leaving no chance to feel protected, messages and comments can come unexpectedly, at any time–this has a strong psychological impact on a teenager [5]. There is also anonymity on the Internet, thanks to which a teenager may not even suspect what kind of person is bullying him, which can cause him even more fear. Unlike physical violence, the consequences of emotional violence in the long term affect psychological health [6]. Therefore, our goal is to study the phenomenon of cyberbullying as a form of suppression of adolescent personality and determine the content of cyberbullying prevention using machine learning (ML) technology, which involves setting the following tasks: types of cyberbullying in online content; identify methods and tools for automatic detection of cyberbullying and hateful expressions; consider open datasets for training machine learning models for automatic detection of cyberbullying; highlight the state-of-the-art methods and analyze the future trends.
This paper is organized as follows: Section 2 explains the literature review method. Section 3 reviews cyberbullying, digital drama, hate speech problems and describes types of cyberbullying. Section 4 reviews research papers in cyberbullying detection area. In this section, we describe each stage of cyberbullying detection on social media from data collection to text classification. Section 5 discusses existing problems and research challenges. Finally, in the last section, we conclude our review.
In this literature review, both qualitative and quantitative analysis methods were integrated and applied [7,8]. Fig. 1 shows the steps of the review and the number of included and excluded articles. The collection of articles started by defining a search string. This string is composed of three search terms: “Machine Learning” and “Cyberbullying” with the logic operator “AND” in between them. Four reference databases were used: Science Direct Platform, IEEE Xplore digital library, Springer, Wiley online library. We included in our research the articles published between 2015 to 2021. In the Screening stage, we excluded ten records. In the Eligibility stage, we excluded eight records without full texts, secondary analysis, and duplicated publications. From the remaining 22 papers, we left 13 for meta-analysis.
Figure 1: Systematic literature review method
3 Classification of Cyberbullying
Digital drama is a new age phrase that refers to forms of abuse and violence among teens in the technology world [9]. The National Crime Prevention Council’s definition of cyber-bullying is “when the internet, cell phones or other devices are used to send or post text or images intended to hurt or embarrass another person” [10]. StopCyberbullying.org, an expert organization dedicated to internet safety, security, and privacy, defines cyberbullying as: “a situation when a child, tween or teen is repeatedly ‘tormented, threatened, harassed, humiliated, embarrassed or otherwise targeted’ by another child or teenager using text messaging, email, instant messaging or any other type of digital technology”.
Cyberbullying can be as simple as continuing to send e-mail to someone who has said they want no further contact with the sender, but it may also include threats, sexual remarks, pejorative labels (i.e., hate speech), ganging up on victims by making them the subject of ridicule in forums, and posting false statements as fact aimed at humiliation.
Like traditional bullying, cyberbullying can be direct and indirect [11]. Direct cyberbullying is direct attacks on a child through letters or messages [12]. In case of indirect harassment, other people (both children and adults) are involved in the process of victim harassment, not always with their consent; the stalker can hack the victim’s account and, mimicking the host, send messages from this account to the victim’s friends, destroying the victim’s communicative field and creating doubt about his moral qualities [13]. One of the most threatening situations is when the stalker publishes information on the network that puts the victim in danger, for example, he places an ad on her behalf about finding sexual partners. Like traditional bullying, cyberbullying includes a continuum of actions, on one pole of which actions that are hardly recognized by others as harassment, and on the other—the violent behavior of the aggressor, which can even lead to the death of the victim.
Recent studies cite the following most common methods of harassment in the electronic space. Fig. 2 demonstrates types of Cyberbullying in Social Networks.
Figure 2: Various ways of Cyberbullying on social media platform
The most emotionally violent form of cyberbullying is Flaming, which begins with insults and develops into a quick emotional exchange of remarks, usually in public, less often in private correspondence [14]. It occurs between two interlocutors with initially equal positions, but sudden aggression introduces an imbalance, which is amplified by the fact that the participant does not know who his opponent can attract to his side in this battle. Forum visitors, witnesses, can join one of the parties and develop rough correspondence, not fully understanding the original meaning of the collision and often considering the situation as a game, unlike the initiators of an aggressive dialogue. You can compare this with a “wall-to-wall” fight, where the participants do not fully understand either what was the reason for the conflict, or what is the criterion for joining comrades-in-arms to each other.
Similar to flaming, but a unidirectional form of bullying is Harassment: these are usually persistent or repetitive words and actions addressed to a particular person that causes him irritation, anxiety, and stress and at the same time do not have a reasonable goal [15]. Cyber harassment is usually expressed in repeated offensive messages to the victim, from which she feels morally destroyed, to which she cannot respond due to fear or inability to identify the stalker, and sometimes she is also forced to pay for the messages received [16].
Another form of harassment is Trolling: cyber trolls publish negative, disturbing information on websites, social network pages, even on memorial pages dedicated to deceased people, provoking a strong emotional reaction. Initially, the term “trolling” is fishing and means fishing with a spinner. “Real” trolls are usually called provocateurs—these are those who use the “weak points” of other people in order to use manipulation to tease a person and get pleasure from his affective explosion. In this case, the aggressor experiences a feeling of omnipotence due to the power over the victim, over her emotional state.
Similar in meaning, but less manipulative and more directly aggressive is Cyberstalking—the use of electronic communications to pursue a victim through repeated alarming and annoying messages, threats of illegal actions or damage, the victims of which may be the recipient of messages or members of his family [17].
In addition, the so-called sexts can cause shame, anxiety or fear. Sexting is the distribution or publication of photo and video materials with naked and semi-naked people [18–20]. The older the children, the higher the probability of their involvement in sexting. According to the study, 10% of young people aged 14–24 sent or published images of themselves with sexual overtones, 15% received such messages directly from someone else [21]. Among the participants of the study of the American National Campaign for the Prevention of Teenage and unwanted Pregnancy, 71% of girls and 67% of boys sent “sexts” to their romantic partners; 21% of girls and 39% of boys sent pictures with sexual overtones to people with whom they would like to have a romantic relationship; 15% of boys and girls sent them to someone familiar only through online communication [22]. If some people send such messages as part of a harmonious relationship within a couple, then others pursue the goals of harassment and harm, for example, posting photos of a naked ex-girlfriend on the Internet as revenge for a painful breakup of relations.
Another form of harassment on the Internet is the dissemination of slander called Denigration: this is the publication and distribution of humiliating and false information about a person, his distorted images, in particular in a sexualized and/or harmful to his reputation, etc. [23]. One of the forms of slander is “online slam-books”. Slam books are notebooks in which classmates post various ratings and comments—“who is the most beautiful girl in the class”, “who dresses the worst”, etc. Accordingly, “online slambooks ''are sites created for entertainment, where classmates publish similar ratings and comments, often rude and unpleasant, for example, and «The worst couple of the class”. Entertainment sites aimed at students and schoolchildren often serve as a platform for this. Some people visit them not to gossip and leave a comment, but simply to check whether they have become another object of slander and malicious entertainment of acquaintances [24].
False information is also spread when impersonating someone else that called Impersonation. The stalker, using a stolen password, sends negative, cruel, or inadequate information to her friends from the victim’s accounts and as if on her behalf [25]. The victim experiences severe humiliation when receiving feedback and often loses friends. In addition, the stalker can use a password to change the victim’s personal profile on the website, post inappropriate, offensive information there, send threatening or humiliating e-mails from the victim’s address. In extreme cases, the stalker may post provocative offensive messages or comments on forums, signing with the victim’s name and indicating her real name, address, and phone number, thereby putting the victim at risk of real harassment and attack.
Disclosure of secrets and fraud that is Outing and Trickery involves the dissemination of personal, secret, confidential information about the victim on the network. This form is similar to the disclosure of secrets “in real life”, which is also accompanied by feelings of shame and fear of rejection on the part of the victim, and differs only in the number of possible witnesses [26].
Exclusion from the community to which a person feels that he belongs can be experienced as social death. Exclusion/ostracism from online communities can occur in any password-protected environment or through removal from the “buddy list”. The experiment showed that exclusion from the online community reduces the self-esteem of the participant and contributes to the fact that in the next community he begins to behave more conformally [27]. Often, after the exclusion, a person joins other groups (in particular, thematically dedicated to revenge on the first community), and this allows him to partially cope with his experiences; a lot of “accomplices” inspire that person and strengthens the belief in the possibility of taking revenge for ostracism—independently or with the help of members of a new group. In the absence of direct grounds, this is an analog of indirect harassment, which is expressed in the isolation and rejection of someone from the group members (“no one wants to sit with him”, “we are not friends with her”).
The importance for a person of his recognition from the community is also exploited when publishing videos of physical violence/hooligan attack (video recording of assaults/happy slapping and hopping). Happy slapping is a hooligan attack on a passerby by a group of teenagers, during which one of the hooligans films what is happening on a mobile phone video camera [28]. To increase the victim’s sense of humiliation, the persecutors post a video of the attack on the Internet, where thousands of viewers can watch and comment on it. Unfortunately, uploading a video to the Internet is much easier than deleting it.
Thus, the main leitmotifs of harassment on the Internet are the exploitation of the significance of the reference community for the victim (involving many witnesses at times increases the feelings of shame, fear, helplessness, and rejection); uncontrolled dissemination of any (false, shameful, confidential) information; provocation of hypertrophied affective feedback from the victim. The purpose of cyberbullying is to worsen the emotional state of the victim and/or destroy her social relationships.
4 Machine Learning in Cyberbullying Detection
Threats arising in the network environment naturally stimulate the development of a research apparatus for studying their factors, mechanisms, and consequences. The emotional nature of those processes that mainly determine the destructive nature of the impact of negative network phenomena on a person sets as a priority the creation of automatic analysis tools that allow assessing the severity of signs of affective states of communication participants in network communication, that is, methods of sentiment analysis in the broad sense of the word. Fig. 3 illustrates cyberbullying detection on social networks using machine-learning techniques. The figure describes cyberbullying detection process from data collection to cyberbullying text classification. The next subsections explain each stage of Fig. 3.
Figure 3: Various ways of Cyberbullying on social media platform (Adapted from [38])
Most ML-based text classification models rely on data as a key component. Data, on the other hand, is meaningless unless it is used to derive information or implications. Training and testing datasets are chosen using data gathered from social networks. Based on observed cases (marked data), supervised models strive to give computer approaches for improving classification accuracy in specified tasks [29]. A good model for a given task should not be confined to samples in a training set alone [30], and therefore should contain unlabeled actual data. The amount of data is unimportant; what matters is if the retrieved data accurately represents social media website activity [31]. Data extracted from social media using keywords, keyphrases, or hashtags (e.g., [31–33]), or data extracted from social networks using user profiles (e.g., [34–36]) are the two main data collection techniques in cyberbullying detection studies (e.g., [37]). The Data Gathering section highlights the problems with different data collection methodologies and their impact on ML technique’s performance.
The main goal of feature extraction is to turn a text from any setting into a keyword schedule that the machine learning model can easily analyze. Feature Extraction also provides information on the texts, such as the highest phrase frequency for each book. In supervised ML, selecting relevant keywords and determining the mechanism for encoding these keywords is required. These search terms and key phrases might have a significant influence on the ability of classification systems to obtain the optimal pattern [39].
A quantifiable attribute of a task that is being observed is referred to as a feature [31]. The fundamental goal of building feature vectors is to supply a collection of learning vectors to ML algorithms so that they can learn how to distinguish between various sorts of classes [32]. Most ML models’ success or failure is determined by feature engineering [33,34]. Several factors may influence the success or failure of a forecast. The characteristics utilized to train the model are the most important [35]. This work consumes the majority of the work in developing cyberbullying prediction models utilizing learning algorithms [35–38]. In this case, the input space (i.e., the characteristics and their combinations that are presented as input to the classifier) must be well-designed.
In many applications, the primary step toward building an effective classifier is proposing a collection of discriminative features that are utilized as inputs to the ML classifier. Human-engineered observations may be used to generate feature vectors, which depend on how features connect with the occurrences of classes. Recent cyberbullying research [32–34] found a link between several characteristics including gender, age, and user character, and the prevalence of cyberbullying. These data may be transformed into a useful form that allows the classifier to distinguish between cyberbullying and neutral text, allowing for the development of successful cyberbullying detection models. Proposing characteristics is a critical step to strengthening classification models’ discriminating capability [35,36]. Similarly, building successful classification models based on ML algorithms requires providing a collection of relevant characteristics of cyberbullying involvement on social media websites [37].
New characteristics have been created based on cutting-edge research to increase the accuracy of cyberbullying prediction. For instance, a lexical syntactic feature has been suggested to deal with offensive language prediction; this strategy is more precise than classic learning-based techniques [38]. Ahmed et al. used datasets from Myspace to build a gender-based methodology for cyberbullying prediction based on gender information from profile information. The gender factor was chosen to increase a classifier’s discriminating abilities. Other studies [39,40] included age and gender as variables, however, these variables are confined to the information supplied by individuals in their online profiles.
Several research [35,38,40,41] looked into cyberbullying prediction using profane phrases as a characteristic. Similarly, to signal bullying, a vocabulary of profane phrases was created, and these words were employed as features for machine learning algorithms [33,37]. The use of obscene phrases as features improves the model’s performance significantly. In a prior paper [32], the quantity of “bad” words and the density of “bad” terms were offered as features for machine learning input. According to the findings, the proportion of ‘‘bad” terms in a communication indicates cyberbullying. Another study [34] created bullying characteristics by expanding a list of pre-defined obscene terms and assigning varying weights to them. These characteristics were combined with bag-of-words and latent semantic characteristics and sent into a machine learning algorithm as a feature input.
In terms of constructing the feature vector, the context-based method outperforms the list-based method [37]. The variety and complexity of cyberbullying, on the other hand, may not necessarily support this conclusion. Several pieces of research [33,39,41] looked at how sentiment analysis may help a classifier discriminate between cyberbullying and non-cyber bullying messages. These pieces of research hypothesized that sentiment traits are a good indicator of the incidence of cyberbullying. The researchers proposed a model to identify and associate troll profiles in Twitter in a study that intended to create ways of reducing cyberbullying activities by detecting troll profiles; they assumed that troll profiles detection is an important step toward detecting cyberbullying occurrence on social networks [38]. This research proposes elements based on tweeted content, posting time, language, and location to enhance authorship identification and evaluate whether or not a profile is a troll. Reference [39] combined characteristics of SM websites’ structure (closeness, degree, betweenness, and eigenvector centralities, as well as clustering coefficient) with characteristics of users (e.g., gender, age behavior,) and content (sentiment, length). The accuracy of machine learning is improved when these characteristics are combined. A comparison of the various factors utilized in cyberbullying detection literature, shown in Table 1, demonstrates how these factors have an impact on prediction accuracy. In Table 1, we built a feature space to two parts as Content-based and Profile-based features. Content-based features included in its stage text that is generated by users and language. Profile-based features contains the information about a user. For example, gender, age, preference of the user. There we show different features as bag of words (BoW), n-gram, program feature (PF), concrete block (CB), local interpretable model-agnostic explanation (LIME), term frequency-inverse document frequency (TF-IDF), emotion bag of words (emotion BW), negation normal form (NNF). The learning process will be successful if the created characteristics include a large number of aspects that are individually associated with a class. This is why the majority of the experiments presented tried to develop a wide range of characteristics. The behavior associated with the incidence of textual cyberbullying should be reflected in the input characteristics. However, feature selection techniques should be used to assess the collection of features. To determine which characteristics are most likely relevant or irrelevant to classes, feature selection algorithms are used.
Even though several text classification classifiers exist, the complexity of the spatial domain poses a significant issue [38]. A text may include hundreds or thousands of different words that are considered features, but many of them may be chaotic, less relevant, noisy, or redundant in relation to the target class. This may cause the classifiers to be misled, lowering their current effectiveness [30,32]. As a result, feature selection should be utilized to exclude noisy, less useful, and redundant features from the feature space, reducing the feature space to a manageable size and enhancing the precision and effectiveness of the classifiers.
Feature subset creation, subset assessment, stopping criteria, and classification result confirmation are the four essential processes of a feature selection approach [39]. We employ a search approach to select a candidate feature subset in the first phase, which is then assessed using goodness criteria in the second phase. When stopping requirements are fulfilled in the third phase, subset creation and assessment come to an end, and the best feature subset from all the candidates is picked. The feature subset will be verified using a validation set in the last stage. Feature selection approaches may be classified into four groups based on how feature subsets are generated: filter model [31,33,37], wrapper model [34,40], embedding model [41], and hybrid model [36]. Because of its accuracy and performance, the large percentage of feature selection approaches for text classification are filter-based approaches. [31,34] provide a thorough examination and comparison of alternative feature selection techniques for generic data.
Feature selection algorithms were seldom taught to detect cyberbullying on online platforms and social networking sites using ML in state-of-the-art research. The bulk of the studies looked at (e.g., [30–32,34,37]) did not use feature selection to figure out which properties are important in ML training. Some studies [33] used principal component analysis and chi-square to choose a relevant feature.
Information gain. The predicted reduction in entropy created by distinguishing instances based on specified attributes is known as information gain. In information theory, entropy is a notion that defines the degree of purity of an arbitrary preparation of data [40].
Pearson correlation. When lowering feature dimensionality and assessing a feature’s discriminating strength in classification models, correlation-based feature selection is often utilized. It’s also a simple model for picking important characteristics. By calculating the Pearson correlation between a feature and a class, you can determine how important it is. The Pearson correlation coefficient [41] is a measurement of the linear relationship between two variables.
Chi-Square. Another common feature selection model is the chi-square test. This test is used in statistics, among other variables, to test the independence of two occurrences. In feature selection, chi-square is used to test whether the occurrences of a feature and class are independent.
There are many different kinds of ML techniques, however, the most well-known and extensively utilized form, supervised ML, was utilized in virtually all research on cyberbullying prediction on social media [31–36]. The accuracy with which an ML algorithm transforms different sorts of past observations or information about a task determines the model’s success. Considerable amount of machine learning’s practical application analyzes the specifics of an issue. After that, a model that allows for correct fact encoding is chosen. Nevertheless, there is no one optimum ML method for all issues [37–41]. As a result, most study chooses and evaluated a variety of supervised classifiers to find the best fit for their issue. The most widely used predictors in the area, as well as the data attributes accessible for trials, are utilized to pick classifiers. Researchers, on the other hand, may only pick which algorithms to use for building a cyberbullying detection model after conducting a full practical trial. The most often utilized machine learning techniques (support vector machines (SVM), naïve Bayes (NB), random forest (RF), decision tree (DT), k nearest neighbours (KNN), logistic regression (LR), radial based method (RB)) for creating cyberbullying prediction models are shown in Table 2.
Cyberbullying, such as cruel emails or instant messages, films and pictures with explicit sexual material, or threats, are all too prevalent in today’s media. As a result, utilizing information and communication technologies (ICT) to humiliate and insult others poses a significant danger [30]. Some pieces of research try to solve this problem using supervised machine learning [30,31].
The authors of the work [32] contrast the approach based on machine learning in its various variants with the lexical one. Having sufficiently high indicators, the lexical approach is limited by the fact that it is aimed at identifying only verbally expressed emotions. The methods of identifying hidden sentiment that allow overcoming this limitation imply the use of knowledge bases containing concepts associated by native speakers with certain emotions. The authors also described three approaches to solving the problem of automatic recognition of cyberbullying in the text: 1) a rules-based approach that identifies keywords and their combinations with words that have an explicit meaning of cyberbullying; at the same time, lexical resources containing vocabulary with prohibited words are used. If a sentence contains a word from a dictionary of banned words, the sentence is classified as malicious; 2) supervised machine learning, which implies the analysis of n-grams, punctuation, emoticons, hashtags, means of negation, etc. with the subsequent use of classifiers based on decision trees, logistic regression and SVM; 3) deep machine learning based on neural networks-convolutional neural networks, gated recurrent unit (GRU), Light Gradient Boosting Machine (GBM); 4) Hierarchical models like hierarchical attention model (HAN) and pseudo-sentence HAN.
Thun and The [33] explore a content-based approach in cyberbullying detection on social networks. They used four ML models to classify the texts to bullying and non-bullying. Four ML models as SVM, NB, DT, KNN were trained to apply different features as bad words, negative emotion, positive emotion, link, proper nouns, and pronouns. In one more similar work [31], the authors presented the cyberbullying early detection issue and suggested two feature sets, text similarities, and time features, that are specially tailored for this topic. In addition, they modified two particular machine learning models, threshold and dual, and tested their performance. In the result, authors prove that the dual model consistently provides the best performance for the early detection of cyberbullying, based on the use of all features for the identification of positive cases along with low thresholds to produce early detections, and simpler features (i.e., profile owner characteristics) for the negative model.
Lopez-Vizcaino et al. [34] examined eleven features including content-based and profile-based features, and current smartphone apps for detecting cyberbullying on social media. The findings indicate that the research identified a collection of relevant characteristics for detecting cyberbullying and developed a model based on those features by assessing the features. The findings also revealed that the suggested mobile application can apply the machine learning model and offer a user interface that allows parents to utilize it to identify cyberbullying in their children. In conclusion, the authors suggested a mobile application that incorporates a machine learning model to help parents in detecting cyberbullying among their children. As a previous study, [40] explores profile-based features in machine learning. In this study, the authors use psychological features in cyberbullying detection on Twitter.
Currently, research is also being conducted aimed at expanding the methods of determining the tonality of texts at the learning stage; for example, an alternative to traditional expert markup is proposed in the form of automatic classification based on markers included in the utterance that have proven to differentiate power (for example, pre-typologized emoticons [34,36,42–44]). Another direction of improving the tools is to expand the list of identifiable emotions; for example, in [37], a classification method based on a partial coincidence prediction algorithm is proposed, which allows us to detect the expression of six basic emotions in texts with a sufficient degree of accuracy: anger, disgust, fear, joy, sadness, and surprise.
The definition of the emotional component of the text is used to study various forms and manifestations of online aggressiveness: trolling [38,45], verbal hostility [39,46–48], cyberbullying through mobile applications [34,41,49], various types of manipulation [50–52], inciting discord [53], etc. The network component of such a form of offline aggression as mass protest is the subject of a complex method of cybermetry, or cybermetric analysis [54–56]. Cybermetry is used for segmenting information flows based on search queries and marker dictionaries; in total, the study includes dictionaries of markers of 14 types of social media documents according to the degree of the radicalism of protest attitudes and message objects expressed in them. Comparing the shares of active-nihilistic and passive-nihilistic message arrays allows us to assess the dynamics of real mass protests, as well as to search for factors and triggers that provoke a mass protest. In addition, cybermetry includes the analysis of tag clouds, geolocation analysis of social media messages, analysis of the demographic characteristics of the authors of messages, analysis of the publication activity of public opinion leaders in social media, and identification of the structure of network social connections.
In cyberbullying detection research uses Accuracy, Precision, Recall, F-measure, and area under the curve receiver operating characteristics (AUC-ROC) curve as evaluation parameters.
The goal of this research was to see whether cyberbullying could be automatically identified using the parameters that make up its identity and characteristics. As a result, we utilized a systematic literature review method to provide an in-depth overview of studies on automated cyberbullying detection. In accordance with the results of the given review, we offer recommendations for future study and suggest enhancements to existing machine learning models and classifiers in automated cyberbullying detection in this section.
To summarize, and per our second goal, we propose that future research take into consideration and completely disclose a set of essential information in order to enhance the quality of future datasets, models, and the performance of classifiers. It is critical to give annotators clear instructions based on the characteristics that define cyberbullying (i.e., intentionality, repetition, aggression, and peer conduct), as well as to guarantee that the annotators are specialists in the area of cyberbullying [51–54]. Furthermore, data extraction from users should be acquired through peers, and users’ privacy should be prioritized during this process [55]. In addition, methods should be created to try to capture the context and nature of the players’ relationships in a cyberbullying incident, since this is a critical component in identifying deliberate damage and repeated aggressions among peers [56].
There are a few flaws in this research that need to be addressed. We contemplated doing a meta-analysis, however, the papers we looked at didn’t offer the required values for this kind of analysis. Furthermore, we were unable to conduct a more in-depth study of user characteristics owing to the fact that the research we examined did not offer this information. Despite these drawbacks, we believe that the current study adds to future attempts to enhance existing approaches and classifiers for automated detection of cyberbullying and online harassment.
Social media is a relatively new human communication medium that has grown in popularity in recent years. Machine learning is utilized in a variety of applications, including social network analysis. This review provides a thorough overview of different applications that use machine learning techniques to analyze social media to detect cyberbullying and online harassment. Our paper explores each step to cyberbullying detection on social media, such as data collection, data preprocessing, data preparation, feature selection and extraction, feature engineering, applying machine learning techniques, and text classification. Various academics have proposed various methods to address the problems of generic metadata architecture, threshold settings, and fragmentation in cyberbullying detection on social networks data streams. To address problems with cyberbullying categorization in social network data, the review also proposed a general metadata architecture for cyberbullying classification on social media. When compared to comparable techniques, the proposed architecture performed better across all evaluation criteria for cyberbullying and online harassment detection.
In further, a more durable automated cyberbullying detection system can be developed by considering the problems as class imbalance data, binary and multi-classification, scalability, class imbalance data, multilingualism, threshold settings, and fragmentation.
Funding Statement: The authors received no specific funding for this study.
Conflicts of Interest: The authors declare that they have no conflicts of interest to report regarding the present study.
References
1. A. Orben, “Teenagers, screens and social media: A narrative review of reviews and key studies,” Social Psychiatry and Psychiatric Epidemiology, vol. 55, no. 4, pp. 407–414, 2020. [Google Scholar]
2. D. Al-Sabti, A. Singh and S. Jha, “Impact of social media on society in a large and specific to teenagers,” in 6th Int. Conf. on Reliability, Infocom Technologies and Optimization (Trends and Future Directions) (ICRITO), Noida, India, pp. 663–667, 2017. [Google Scholar]
3. A. Kumar and N. Sachdeva, “A Bi-GRU with attention and CapsNet hybrid model for cyberbullying detection on social media,” World Wide Web, vol. 25, no. 4, pp. 1537–1550, 2022. [Google Scholar]
4. B. Belsey, “Cyberbullying: An Emerging Threat to the «Always on» Generation,” 2019. [Online]. Available: https://billbelsey.com/?p=1827. [Google Scholar]
5. M. Boniel-Nissim and H. Sasson, “Bullying victimization and poor relationships with parents as risk factors of problematic internet use in adolescence,” Computers in Human Behavior, vol. 88, pp. 176–183, 2018. [Google Scholar]
6. P. K. Bender, C. Plante and D. A. Gentile, “The effects of violent media content on aggression,” Current Opinion in Psychology, vol. 19, no. 1, pp. 104–108, 2018. [Google Scholar]
7. Z. Munn, M. D. Peters, C. Stern, C. Tufanaru, A. McArthur et al., “Systematic review or scoping review? guidance for authors when choosing between a systematic or scoping review approach,” BMC Medical Research Methodology, vol. 18, no. 1, pp. 1–7, 2018. [Google Scholar]
8. M. J. Grant and A. Booth, “A typology of reviews: An analysis of 14 review types and associated methodologies,” Health Information and Libraries Journal, vol. 26, no. 2, pp. 91–108, 2009. [Google Scholar]
9. M. Brandau, T. Dilley, C. Schaumleffel and L. Himawan, “Digital citizenship among appalachian middle schoolers: The common sense digital citizenship curriculum,” Health Education Journal, vol. 81, no. 2, pp. 157–169, 2022. [Google Scholar]
10. S. Day, K. Bussey, N. Trompeter and D. Mitchison, “The impact of teasing and bullying victimization on disordered eating and body image disturbance among adolescents: A systematic review,” Trauma, Violence and Abuse, vol. 23, no. 3, pp. 985–1006, 2022. [Google Scholar]
11. J. S. Hong, D. H. Kim, R. Thornberg, J. H. Kang and J. T. Morgan, “Correlates of direct and indirect forms of cyberbullying victimization involving south Korean adolescents: An ecological perspective,” Computers in Human Behavior, vol. 87, pp. 327–336, 2018. [Google Scholar]
12. G. Sarna and M. P. S. Bhatia, “Content based approach to find the credibility of user in social networks: An application of cyberbullying,” International Journal of Machine Learning and Cybernetics, vol. 8, no. 2, pp. 677–689, 2015. [Google Scholar]
13. S. Buelga, J. Postigo, B. Martínez-Ferrer, M. J. Cava and J. Ortega-Barón, “Cyberbullying among adolescents: Psychometric properties of the CYB-AGS cyber-aggressor scale,” International Journal of Environmental Research and Public Health, vol. 17, no. 9, pp. 3090, 2020. [Google Scholar]
14. W. N. H. W. Ali, M. Mohd and F. Fauzi, “Cyberbullying detection: An overview,” in 2018 Cyber Resilience Conf. (CRC), Putrajaya, Malaysia, IEEE, pp. 1–3, 2018. [Google Scholar]
15. M. Bugueño and M. Mendoza, “Learning to detect online harassment on twitter with the transformer,” in Joint European Conf. on Machine Learning and Knowledge Discovery in Databases, Cham, Springer, pp. 298–306, 2019. [Google Scholar]
16. K. Van Royen, K. Poels, H. Vandebosch and P. Adam, “Thinking before posting?” reducing cyber harassment on social networking sites through a reflective message,” Computers in Human Behavior, vol. 66, pp. 345–352, 2017. [Google Scholar]
17. N. Sest and E. March, “Constructing the cyber-troll: Psychopathy, sadism, and empathy,” Personality and Individual Differences, vol. 119, pp. 69–72, 2017. [Google Scholar]
18. M. Smoker and E. March, “Predicting perpetration of intimate partner cyberstalking: Gender and the dark tetrad,” Computers in Human Behavior, vol. 72, pp. 390–396, 2017. [Google Scholar]
19. Y. Barrense-Dias, A. Berchtold, J. C. Surís and C. Akre, “Sexting and the definition issue,” Journal of Adolescent Health, vol. 61, no. 5, pp. 544–554, 2017. [Google Scholar]
20. J. L. J. Medrano, F. Lopez Rosales and M. Gámez-Guadix, “Assessing the links of sexting, cybervictimization, depression, and suicidal ideation among university students,” Archives of Suicide Research, vol. 22, no. 1, pp. 153–164, 2018. [Google Scholar]
21. R. M. Kowalski, S. P. Limber and A. McCord, “A developmental approach to cyberbullying: Prevalence and protective factors,” Aggression and Violent Behavior, vol. 45, pp. 20–32, 2019. [Google Scholar]
22. G. Variyan and J. Wilkinson, “The erasure of sexual harassment in elite private boys’ schools,” Gender and Education, vol. 34, no. 2, pp. 183–198, 2022. [Google Scholar]
23. G. Allsopp, J. Rosenthal, J. Blythe and J. S. Taggar, “Defining and measuring denigration of general practice in medical education,” Education for Primary Care, vol. 31, no. 4, pp. 205–209, 2020. [Google Scholar]
24. A. Baybarin, M. V. Afonin, E. I. Maksimenko, V. V. Goncharov and D. A. Singilevich, “Information security of internet users: Technological and legal opportunities for personal protection,” Eurasian Journal of Biosciences, vol. 14, no. 2, pp. 6805–6811, 2020. [Google Scholar]
25. E. Villar-Rodriguez, J. D. Ser, S. Gil-Lopez, M. N. Bilbao and S. Salcedo-Sanz, “A Meta-heuristic learning approach for the non-intrusive detection of impersonation attacks in social networks,” International Journal of Bio-Inspired Computation, vol. 10, no. 2, pp. 109–118, 2017. [Google Scholar]
26. A. Cassiman, “Spiders on the world wide web: Cyber trickery and gender fraud among youth in an Accra zongo,” Social Anthropology, vol. 27, no. 3, pp. 486–500, 2019. [Google Scholar]
27. K. Williams, C. Cheung and W. Choi, “Cyberostracism: Effects of Being Ignored over the Internet,” Journal of Personality and Social Psychology, vol. 79, no. 5, pp. 748–762, 2000. [Google Scholar]
28. D. Álvarez-García, J. C. Núñez, A. Barreiro-Collazo and T. García, “Validation of the cybervictimization questionnaire (CYVIC) for adolescents,” Computers in Human Behavior, vol. 70, pp. 270–281, 2017. [Google Scholar]
29. A. Sanchez-Medina, I. Galvan-Sanchez and M. Fernandez-Monro, “Applying artificial intelligence to explore sexual cyberbullying behaviour,” Heliyon, vol. 6, no. 1, pp. 1–9, 2020. [Google Scholar]
30. A. Perera and P. Fernandol, “Accurate cyberbullying detection and prevention on social media,” Procedia Computer Science, vol. 181, pp. 605–611, 2021. [Google Scholar]
31. E. Zinoviyeva, W. Karl Hardle and S. Lessmann, “Antisocial online behavior detection using deep learning. Decision Support Systems, vol. 138, no. 1, pp. 1–9, 2020. [Google Scholar]
32. R. Zhao and K. Mao, “Cyberbullying detection based on semantic-enhanced marginalized denoising auto-encoder,” IEEE Transactions on Affective Computing, vol. 8, no. 3, pp. 328–329, 2016. [Google Scholar]
33. L. Thun, P. The and C. Cheng, “CyberAid: Are your children safe from cyberbullying?,” Journal of King Saud University-Computer and Information Sciences, vol. 34, no. 7, pp. 4099–4108, 2022. [Google Scholar]
34. M. Lopez-Vizcaino, F. Novoa, V. Carneiro and F. Cacheida, “Early detection of cyberbullying on social media networks,” Future Genereation Computer Systems,” vol. 118, pp. 219–229, 2021. [Google Scholar]
35. M. Al-garadi, K. Varatham and S. Ravana, “Cybercrime detection in online communications: The experimantal case of cyberbullying detection in the twitter network,” Computers in Human Behavior, vol. 63, pp. 433–443, 2016. [Google Scholar]
36. O. Gencoglu, “Cyberbullying detection with fairness constraints,” IEEE Internet Computing, vol. 25, no. 1, pp. 20–29, 2020. [Google Scholar]
37. Z. Meng, S. Tian and L. Yu, “Regional bullying text recognition based on two-branch parallel neural networks,” Automatic Control and Computer Sciences, vol. 54, no. 4, pp. 323–334, 2020. [Google Scholar]
38. J. Hani, M. Nashaat, M. Ahmed, Z. Emad, E. Amer et al., “Social media cyberbullying detection using machine learning,” International Journal of Advanced Computer Science and Applications, vol. 10, no. 5, pp. 703–707, 2019. [Google Scholar]
39. T. Ahmed, S. Ivan, M. Kabir, H. Mahmud and K. Hasan, “Performance analysis of transformer-based architectures and their ensembles to detect trait-based cyberbullying,” Social Network Analysis and Mining, vol. 12, no. 1, pp. 1–17, 2022. [Google Scholar]
40. V. Balakrishnan, Sh. Khan and H. Arabnia, “Improving Cyberbullying Detection using Twitter Users’ Psychological Features and Machine Learning,” Computers and Security, vol. 90, no. 1, pp. 1–11, 2019. [Google Scholar]
41. Y. Silva, D. Hall and C. Rich, “BullyBlocker: Toward an interdisciplinary approach to identify cyberbullying,” Social Network Analysis and Mining, vol. 8, no. 1, 1–15, 2018. [Google Scholar]
42. M. Raj and S. Singh, K. Solanki and R. Selvanambi, “An application to detect cyberbullying using machine learning and deep learning techniques,” SN Computer Science, vol. 3, no. 5, pp. 1–13, 2022. [Google Scholar]
43. B. Haidar, M. Chamoun and A. Serhrouchni, “A multilingual system for cyberbullying detection: Arabic content detection using machine learning,” Advances in Science, Technology and Engineering Systems Journal, vol. 2, no. 6, pp. 275–284, 2017. [Google Scholar]
44. E. Raisi and B. Huang, “Cyberbullying detection with weakly supervised machine learning, “ in Proc. of the 2017 IEEE/ACM Int. Conf. on Advances in Social Networks Analysis and Mining, Sydney, Australia, pp. 409–416, 2017. [Google Scholar]
45. B. Omarov, A. Altayeva, A. Turganbayeva, G. Abdulkarimova, F. Gusmanova et al., “Agent based modeling of smart grids in smart cities,” in Int. Conf. on Electronic Governance and Open Society: Challenges in Eurasia, St. Petersburg, Russia, pp. 3–13, 2018. [Google Scholar]
46. O. Oriola and Kotzé, E., “Evaluating machine learning techniques for detecting offensive and hate speech in South African tweets,” IEEE Access, vol. 8, pp. 21496–21509, 2020. [Google Scholar]
47. B. Omarov, N. Saparkhojayev, S. Shekerbekova, O. Akhmetova, M. Sakypbekova et al., “Artificial intelligence in medicine: Real time electronic stethoscope for heart diseases detection,” Computers, Materials & Continua, vol. 70, no. 2, pp. 2815–2833, 2022. [Google Scholar]
48. I. Aljarah, M. Habib, N. Hijazi, H. Faris, R. Qaddoura et al., “Intelligent detection of hate speech in arabic social network: A machine learning approach,” Journal of Information Science, vol. 47, no. 4, pp. 483–501, 2020. [Google Scholar]
49. M. M. Singh, P. J. Ng, K. M. Ya, M. H. Husin and N. H. A. H. Malim, “Cyberbullying and a mobile game app? an initial perspective on an alternative solution,” Journal of Information Processing Systems, vol. 13, no. 3, pp. 559–572, 2017. [Google Scholar]
50. F. Kazerooni, S. H. Taylor, N. N. Bazarova and J. Whitlock, “Cyberbullying bystander intervention: The number of offenders and retweeting predict likelihood of helping a cyberbullying victim,” Journal of Computer-Mediated Communication, vol. 23, no. 3, pp. 146–162, 2018. [Google Scholar]
51. A. A. Nuaimi, “Effectiveness of cyberbullying prevention strategies in the UAE in ICT analysis and applications,” in ICT Analysis and Applications, vol. 2, pp. 731–739, 2021. [Google Scholar]
52. N. A. Palomares and V. S. Wingate, “Victims’ goal understanding, uncertainty reduction, and perceptions in cyberbullying: Theoretical evidence from three experiments,” Journal of Computer-Mediated Communication, vol. 25, no. 4, pp. 253–273, 2020. [Google Scholar]
53. N. Thompson and D. Woodger, ““I hope the river floods”: Online hate speech towards gypsy, roma and traveller communities,” British Journal of Community Justice, vol. 16, no. 1, pp. 41–63, 2020. [Google Scholar]
54. A. W. Hanley and E. L. Garland, “The mindful personality: A meta-analysis from a cybernetic perspective,” Mindfulness, vol. 8, no. 6, pp. 1456–1470, 2017. [Google Scholar]
55. K. Kasianiuk, “A System-cybernetic approach to the study of political power. Introductory remarks,” Kybernetes, vol. 47, no. 6, pp. 1262–1276, 2018. [Google Scholar]
56. S. Li, Y. Xue, G. Feng and B. Xu, “Simulation analysis of intermittent arc grounding fault applying with improved cybernetic arc model,” The Journal of Engineering, vol. 2019, no. 16, pp. 3196–3201, 2019. [Google Scholar]
Cite This Article
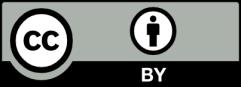