Open Access
ARTICLE
Chaotic Metaheuristics with Multi-Spiking Neural Network Based Cloud Intrusion Detection
1 Department of Management Information Systems, Faculty of Economics and Administration, King Abdulaziz University, Jeddah, 21589, Saudi Arabia
2 Department of Business Administration, Faculty of Economics and Administration, King Abdulaziz University, Jeddah, 21589, Saudi Arabia
* Corresponding Author: Mohammad Yamin. Email:
Computers, Materials & Continua 2023, 74(3), 6101-6118. https://doi.org/10.32604/cmc.2023.033677
Received 24 June 2022; Accepted 29 September 2022; Issue published 28 December 2022
Abstract
Cloud Computing (CC) provides data storage options as well as computing services to its users through the Internet. On the other hand, cloud users are concerned about security and privacy issues due to the increased number of cyberattacks. Data protection has become an important issue since the users’ information gets exposed to third parties. Computer networks are exposed to different types of attacks which have extensively grown in addition to the novel intrusion methods and hacking tools. Intrusion Detection Systems (IDSs) can be used in a network to manage suspicious activities. These IDSs monitor the activities of the CC environment and decide whether an activity is legitimate (normal) or malicious (intrusive) based on the established system’s confidentiality, availability and integrity of the data sources. In the current study, a Chaotic Metaheuristics with Optimal Multi-Spiking Neural Network-based Intrusion Detection (CMOMSNN-ID) model is proposed to secure the cloud environment. The presented CMOMSNN-ID model involves the Chaotic Artificial Bee Colony Optimization-based Feature Selection (CABC-FS) technique to reduce the curse of dimensionality. In addition, the Multi-Spiking Neural Network (MSNN) classifier is also used based on the simulation of brain functioning. It is applied to resolve pattern classification problems. In order to fine-tune the parameters relevant to the MSNN model, the Whale Optimization Algorithm (WOA) is employed to boost the classification results. To demonstrate the superiority of the proposed CMOMSNN-ID model, a useful set of simulations was performed. The simulation outcomes inferred that the proposed CMOMSNN-ID model accomplished a superior performance over other models with a maximum accuracy of 99.20%.Keywords
Cite This Article
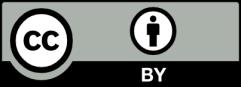
This work is licensed under a Creative Commons Attribution 4.0 International License , which permits unrestricted use, distribution, and reproduction in any medium, provided the original work is properly cited.