Open Access
ARTICLE
Identification of Anomaly Scenes in Videos Using Graph Neural Networks
1 Department of Software Engineering, Lahore Garrison University, Lahore, 54000, Pakistan
2 School of Business, Skyline University College, Sharjah, UAE
3 Al-Madinah International University–Faculty of Computer and Information Technology, Kuala Lumpur, Malaysia
4 College of Computer and Information Systems, Islamic University of Madinah, Madinah, Kingdom of Saudi Arabia
5 School of IT, Skyline University College, Sharjah, UAE
6 Faculty of Engineering, Computing and Science (FECS)-Swinburne University of Technology, Sarawak
7 Department of General Education, Skyline University College, University City of Sharjah, UAE
8 Department of Computer Science, Lahore Garrison University, Lahore, 54000, Pakistan
* Corresponding Author: Waqas Nawaz. Email:
Computers, Materials & Continua 2023, 74(3), 5417-5430. https://doi.org/10.32604/cmc.2023.033590
Received 21 June 2022; Accepted 22 September 2022; Issue published 28 December 2022
Abstract
Generally, conventional methods for anomaly detection rely on clustering, proximity, or classification. With the massive growth in surveillance videos, outliers or anomalies find ingenious ways to obscure themselves in the network and make conventional techniques inefficient. This research explores the structure of Graph neural networks (GNNs) that generalize deep learning frameworks to graph-structured data. Every node in the graph structure is labeled and anomalies, represented by unlabeled nodes, are predicted by performing random walks on the node-based graph structures. Due to their strong learning abilities, GNNs gained popularity in various domains such as natural language processing, social network analytics and healthcare. Anomaly detection is a challenging task in computer vision but the proposed algorithm using GNNs efficiently performs the identification of anomalies. The Graph-based deep learning networks are designed to predict unknown objects and outliers. In our case, they detect unusual objects in the form of malicious nodes. The edges between nodes represent a relationship of nodes among each other. In case of anomaly, such as the bike rider in Pedestrians data, the rider node has a negative value for the edge and it is identified as an anomaly. The encoding and decoding layers are crucial for determining how statistical measurements affect anomaly identification and for correcting the graph path to the best possible outcome. Results show that the proposed framework is a step ahead of the traditional approaches in detecting unusual activities, which shows a huge potential in automatically monitoring surveillance videos. Performing autonomous monitoring of CCTV, crime control and damage or destruction by a group of people or crowd can be identified and alarms may be triggered in unusual activities in streets or public places. The suggested GNN model improves accuracy by 4% for the Pedestrian 2 dataset and 12% for the Pedestrian 1 dataset compared to a few state-of-the-art techniques.Keywords
Cite This Article
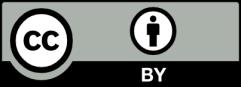
This work is licensed under a Creative Commons Attribution 4.0 International License , which permits unrestricted use, distribution, and reproduction in any medium, provided the original work is properly cited.