Open Access
ARTICLE
Smart Techniques for LULC Micro Class Classification Using Landsat8 Imagery
1 Department of Computer Science, Khwaja Fareed University of Engineering and Information Technology, Rahim Yar Khan, 64200, Pakistan
2 Department of Information and Communication Engineering, Yeungnam University, Gyeongsan-si, 38541, Korea
* Corresponding Author: Imran Ashraf. Email:
Computers, Materials & Continua 2023, 74(3), 5545-5557. https://doi.org/10.32604/cmc.2023.033449
Received 16 June 2022; Accepted 09 September 2022; Issue published 28 December 2022
Abstract
Wheat species play important role in the price of products and wheat production estimation. There are several mathematical models used for the estimation of the wheat crop but these models are implemented without considering the wheat species which is an important independent variable. The task of wheat species identification is challenging both for human experts as well as for computer vision-based solutions. With the use of satellite remote sensing, it is possible to identify and monitor wheat species on a large scale at any stage of the crop life cycle. In this work, nine popular wheat species are identified by using Landsat8 operational land imager (OLI) and thermal infrared sensor (TIRS) data. Two thousand samples of each wheat crop species are acquired every fifteen days with a temporal resolution of ten multispectral bands (band two to band eleven). This study employs random forest (RF), artificial neural network, support vector machine, Naïve Bayes, and logistic regression for nine types of wheat classification. In addition, deep neural networks are also developed. Experimental results indicate that RF shows the best performance of 91% accuracy while DNN obtains a 90.2% accuracy. Results suggest that remotely sensed data can be used in wheat type estimation and to improve the performance of the mathematical models.Keywords
Cite This Article
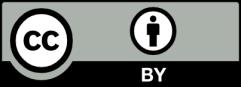
This work is licensed under a Creative Commons Attribution 4.0 International License , which permits unrestricted use, distribution, and reproduction in any medium, provided the original work is properly cited.