Open Access
ARTICLE
Functional Nonparametric Predictions in Food Industry Using Near-Infrared Spectroscopy Measurement
1 Department of Mathematics, College of Science, King Khalid University, Abha, 62529, Saudi Arabia
2 Statistical Research and Studies Support Unit, King Khalid University, Abha, 62529, Saudi Arabia
3 Laboratory of Statistics and Stochastic Processes University of Djillali Liabes BP 89, Sidi Bel Abbes, 22000, Algeria
4 Medical Faculty, Djillali Liabes University BP 89, Sidi Bel Abbes, 22000, Algeria
* Corresponding Author: Ibrahim M. Almanjahie. Email:
Computers, Materials & Continua 2023, 74(3), 6307-6319. https://doi.org/10.32604/cmc.2023.033441
Received 16 June 2022; Accepted 08 September 2022; Issue published 28 December 2022
Abstract
Functional statistics is a new technique for dealing with data that can be viewed as curves or images. Parallel to this approach, the Near-Infrared Reflectance (NIR) spectroscopy methodology has been used in modern chemistry as a rapid, low-cost, and exact means of assessing an object’s chemical properties. In this research, we investigate the quality of corn and cookie dough by analyzing the spectroscopic technique using certain cutting-edge statistical models. By analyzing spectral data and applying functional models to it, we could predict the chemical components of corn and cookie dough. Kernel Functional Classical Estimation (KFCE), Kernel Functional Quantile Estimation (KFQE), Kernel Functional Expectile Estimation (KFEE), Semi-Partial Linear Functional Classical Estimation (SPLFCE), Semi-Partial Linear Functional Quantile Estimation (SPLFQE), and Semi-Partial Linear Functional Expectile Estimation (SPLFEE) are models used to accurately estimate the different quantities present in Corn and Cookie dough. The selection of these functional models is based on their ability to construct a forecast region with a high level of confidence. We demonstrate that the considered models outperform traditional models such as the partial least-squares regression and the principal component regression in terms of prediction accuracy. Furthermore, we show that the proposed models are more robust than competing models such as SPLFQE and SPLFEE in the sense that data heterogeneity has no effect on their efficiency.Keywords
Cite This Article
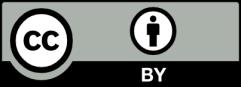