Open Access
ARTICLE
Impediments of Cognitive System Engineering in Machine-Human Modeling
1 National Institute of Electronics and Information Technology (NIELIT), J&K, 191132, India
2 Department of Computer Science & Engineering, Lovely Professional University (LPU), Punjab, 144402, India
3 Department of Computer Science, COMSATS University Islamabad, Islamabad, 45550, Pakistan
4 Department of Computer Science, Govt. Degree College Sumbal Kashmir, 193501, India
5 Industrial Engineering Department, College of Engineering, King Saud University, P.O. Box 800, Riyadh, 11421, Saudi Arabia
6 Department of Information and Communication Engineering, Yeungnam University, Gyeongsan, 38541, Korea
* Corresponding Author: Muhammad Shafiq. Email:
Computers, Materials & Continua 2023, 74(3), 6689-6701. https://doi.org/10.32604/cmc.2023.032998
Received 03 June 2022; Accepted 19 August 2022; Issue published 28 December 2022
Abstract
A comprehensive understanding of human intelligence is still an ongoing process, i.e., human and information security are not yet perfectly matched. By understanding cognitive processes, designers can design humanized cognitive information systems (CIS). The need for this research is justified because today’s business decision makers are faced with questions they cannot answer in a given amount of time without the use of cognitive information systems. The researchers aim to better strengthen cognitive information systems with more pronounced cognitive thresholds by demonstrating the resilience of cognitive resonant frequencies to reveal possible responses to improve the efficiency of human-computer interaction (HCI). A practice-oriented research approach included research analysis and a review of existing articles to pursue a comparative research model; thereafter, a model development paradigm was used to observe and monitor the progression of CIS during HCI. The scope of our research provides a broader perspective on how different disciplines affect HCI and how human cognitive models can be enhanced to enrich complements. We have identified a significant gap in the current literature on mental processing resulting from a wide range of theory and practice.Keywords
In Cognitive Information Systems (CIS) and machine models, the exchange of information in the domain of human cognition and human-computer interaction leads to many problems. To develop goal-oriented strategies, independent research requires organizational and environmental analysis to understand human thinking and cognition. Starting from human cognition, our goal and proposal is to discover potential links in Human-Computer Interaction (HCI) that can improve relationship quality. To improve quality, we create further refined basic models to better understand the human mind and elucidate the shortcomings of computing institutions to improve their use in commercial applications. Thus, neurobiology can be considered an external study related to neurology, while social cognition can be considered an internal study related to neurology. Studying these fields requires an understanding of cognitive information systems (CIS) and HCI, as HCI involves different human and machine processes [1–3].
To better understand human decision-making (DM), CIS-based models can predict true DMs or replace them with innovative machines using Intelligent Automation System (IAS) technology. Robots and machines have replaced humans due to various tasks, human factors and personnel constraints. Robotic Process Automation (RPA) support can help companies save money and ensure the quality of other life parameters as robots can make informed decisions. They leverage these technologies and provide decision-making techniques to benefit from support. This HCI decision support can positively impact informed decisions that impact the business. A person with cognitive intelligence can process messages from computers at cognitive frequencies, integrate them and build a knowledge base, as shown in Fig. 1. Among them, the carbon-silicon (CS) model, database schema and cognitive information communication model all illustrate this point from the perspective of carbon composition.
Figure 1: Cognitive info-communication
Although some alternative prediction techniques currently exist, these solutions are not perfect due to the lack of awareness of better human-computer interaction and decision support; further research into human decision-making and its architecture is required. Therefore, we developed a partial support model. Applying multiple fields of science to explore human functioning is inevitable. When the machine is critical, it synchronizes the human brain and decision-making. Neurology is a branch of neuroscience that studies the brain and nervous system. According to research in this field of neuroscience, the more we learn about how the brain works, the less we seem to be able to make decisions based on free will, and the less responsible it seems to be for our choices [4,5]. Here, an inner mental and psychological examination is essential, which significantly affects the brain. Injury and micro-stimulation studies have shown that axons of microtubules (MTs) form the basis of neural decision-making mechanisms [6,7]. These units work in tandem with the scan plan to transmit relevant information, in addition to the evidence gathered during decision preparation, so that better conclusions can be drawn.
Cognitive knowledge of the Carbon Actor Perspective Paradigm describes human data and information processing and understands the importance of such neural causes for human decision-making. Many brain-based neuroscience techniques or online marketing tools related to human DM may have a physiological impact on immediate decision-making due to cognitive gaze direction. Neuromarketing aims to combine visual attention with the cognitive and emotional responses of customers. The speed and accuracy of the decision-making process is controlled by the limit that stops the decision-making process. Noise is an unwelcome element of brain function that affects the way neurons present information. Chaos is not always distinguishable from signals from the brain or agents. Therefore, agents must learn to adjust their decisions to account for noise-enhanced uncertainty. Significant error rates can cause agents to change policies by slowing down the process.
Social cognition is related to neurobiology and refers to the various cognitive abilities that enable individuals to understand, communicate, perceive and use knowledge. These skills include the ability to read social cues such as facial gestures, intonation, and posture, infer what other people are thinking (a type of cognitive reasoning), make moral judgments, and control emotions and feelings [8–10]. There are three social cognitive processes: observation, evaluation, and management. There are three social cognitive processes: observation, evaluation, and management. Observation refers to obedient aspects of perception or representation by neurocognitive factors, including observation of facial and verbal information, sense of body movement, and other elements. However, evaluation is emotion recognition, affective and cognitive sensitivity and other similar processes are examples of evaluation or interpretation processes. Finally, management is a set of supervisory activities that include behavior, self-awareness, observation, cognitive control, and evaluation.
As described in Machine Learning (ML), Supervised Machine Learning (SML) extracts linguistic information in cognition. In contrast, unsupervised machine learning (UML) can be used for evaluation and reinforcement (ER) regulation to construct CIS. In addition, supervised learning (SL) retrieves linguistic information in cognition, while UML in ER supervision can be used to develop CIS [11]. Artificial Neural Networks (ANNs) are ideal for ML applications because they can emulate the human brain and nervous system and reverse their work to create a CIS. A multi-layer artificial neural network with different weights serves as the basis for decision-making. The complexity of artificial neural networks may be more important than the complexity of the brain, which has about 1011 neurons and about 1015 trillion connections. It all depends on who designed the network. CIS powered by artificial neural networks can more accurately predict and support humans, the achievable goals are limitless while replacing human decision-making and expanding cognitive capabilities. The structure of artificial neurons is similar to the function of biological neurons, as shown in Fig. 2.
Figure 2: Artificial neural network (ANN)
Dendrites such as weight w1 plus bias b1 originate from synaptic vesicles x1 and move to postnatal neurons y1 to form input to cells. The entrance to neutrons as axons and the entrance to the cell body (nucleus) as f(x) serve as core functions therein.
The basic formula shows the ANN solution with or without activation function, which can be seen as an example of the basic neural network based ANN approach applied in this case. In this case, the input and output have a linear relationship, which can be used as a multiple regression model when the activation function is nonlinear. Therefore, artificial neural networks can fit nonlinear data using nonlinear neuron functions. In humans, information requires non-linear synchronization mechanisms to flow through the cortical structure of the conscious brain. Therefore, human-centric intelligent technology algorithms can improve cognitive processing in CIS platforms [12]. However, the result for Y1 is from f (neutron).
Based on the neural network objective, many classes of ANNs are used in HCI [13] to represent it. Specific machine learning is proposed to design brain structures based on cognitive functions. For example, the cerebellum and basal ganglia (BG) undergo supervised learning. Therefore, brain-inspired technology requires real-time computing power, which is the basis for the development of CIS and neurocognitive intelligence [14].
Based on network models, neuromorphic computers can be developed. Bionic Cognitive Computing Systems (BiCoSS) can be constructed using its large-scale stimulated neural network (SNN), which can simulate many biological and cognitive tasks, such as path planning. It also facilitates the modeling of human decision-making, thereby facilitating the development of CIS. The basal ganglia, cerebellum, hippocampus, and thalamus are among the many nuclei considered by BiCoSS.
To sum up, related research fields such as neurology have had a significant impact on various fields. Therefore, in order to gain broader knowledge, human-friendly approaches to modeling trends need to be transferred to other domains. Our research approach includes an overview of how other domains affect HCI [15] and its performance, supported by a fundamental model in which we employ a multidisciplinary approach to support the detailed insights needed to uncover potential added value to Illustrating the resulting frequency of cognitive resonance.
This study aims to improve the responsiveness and efficiency of human-computer interaction by demonstrating the resilience of cognitive resonant frequencies and their role in HCI information exchange. Hurwitz asserts that CIS relies on three key ideas to gain cognition: contextual insights from the framework, data interpretation (the development of a theory explaining events), and data fitting over time [16]. On the other hand, Wang and Ruhe argue that it is necessary to adopt a “pejorative” paradigm with its own set of rules, resources, and procedures, rather than traditional modulo arithmetic for cognitive decision-making [17]. Ogiela collected cases of cognitive information processing techniques applied in different fields of computer science and information technology [18]. By extending the framework based on validated publications, in addition to contextual understanding of details, hypothesis generation and continuous learning from data over time in the previously defined framework [19].
Through cognitive resonance, this combination creates a dynamic. While lexical data is collected to support the interpretation of understanding, cognitive resonance directs the process towards automated data knowledge [20]. Mental resonance is a beneficial synergy of HCI, which utilizes cognitive messaging as a function and medium. In a physiological analogy, cognitive communication ensures that information delivered as a stimulus reaches receptors that act as sensors or sense organs. It can affect the system, and the system can react accordingly. Stimuli are messages or actual data sent between parties involved in information communication activities that improve the perception of both parties throughout HCI [6]. Based on this interpretation, CIS can be viewed as part of Wang’s architecture in three ways. HCI allows us to examine the current course of cognitive processes as a basis for selection. Information Systems (IS) material includes information composed of statistics and applications as a coherent group. This is because the first cycle has no unavoidable impact on the natural world (NW), abstract world (AW), or cognitive information systems [21]. The concept and design of thought-driven management support programs are linked to cognitive approaches that mimic the psychological and neurophysiological processes of fact-finding as they occur in the brains of capable and gifted individuals [22].
HCI defines the structure for building a repository that provides information and service complements to support the human cognitive paradigm. From a neurological perspective, CI requires more research on the brain mechanisms relevant to social intelligence in social neurology on which mirror neuron systems rely. Social psychology has uncovered a relationship between this empathic social response and fundamental neurological tools based on mirror neuron systems. However, the quality of mirror neuron systems can vary within individuals over time. Mirror neuron connections control the ability to observe, imitate, and invent. This research article proposes an evolving operating system based on socio-neurological information to infer health differences over time. The authors argue for the importance of metaphors as mental models in bilateral planning processes, based on social psychological science and adaptive routing protocols.
In joint DM ownership, the state plays an important role in decision-making. The basis for acknowledging the origin of events is a person’s state, which determines the degree to which personal characteristics affect him or another reality. The above-mentioned mental processes lead to mutual understanding of carbon (C) and silicon (S) units, which will be an important part of informed collaborative decision-making [23]. A reasonable mutual decision between two persons consists of three basic elements [24]. Both people need to choose the same option, be positive about it, and both have empathy for each other’s feelings about the decision. In theory, all of the above-mentioned mental processes should lead to urgent and rational collective decision-making due to their dynamic interactions [25]. However, since all three criteria represent relatively high thresholds of their quality, there are several common judgments when one (or more) of the three factors fails in reality for one or both individuals. Therefore, the DM was modified to show the selection and interaction of carbon and silicon reagents. The term “cognitive resonance” refers to the complete process of enhancing the performance of silicon and carbon compounds. However, both parties benefit from new insights gained through cognitive resonance processes.
The correlation of this dynamic interaction changes the results of models that describe collaborative decision-making using the synergies found in cognitive resonance processes. Based on this decline,we will not discuss or consider the same point that is partly used as positive sentiment, namely the relationship between carbon and silicon reagents. Instead, we examine semantic but not empathic understanding that significantly affects the human collaborative DM paradigm [26]. The purpose of the human-human model transformation is to better understand and simulate silicon and carbon decision-making. As shown in Fig. 3, the carbon-silicon framework [27] is shown in a carbon-silicon (CS) info-communication prototype containing UML, arrow links reflect what the variables contain, and dashed lines show groups and clubs. The following parameters characterize the bidirectional vision of communication:
• Content (CT): Prospective effect (pe) and resonance (r) make up the non-static, adaptive base communication unit, which is defined by the communication directions, setting, and convergence rate.
• Stimulus (s)
• Context (CX)
• Cognitive resonance (r): the quantifiable value additions to the overall procedure require the agent’s silicon and carbon. The procedure enhances the result, whereas both sides benefit from conveyed values.
• Action (ac): Realization of a process.
• Prospective effect (ep): the content-determining influence embedded into an impulse’s shape.
Figure 3: Info-communication framework of carbon-silicon [27]
The parts of the interface that ensure the integrity of inputs and outputs are ep and pe, as the detected inputs are integrated and analyzed with information about what is contained in the carbon agent and the silicon composition in the ligands through the silicon environment. The silicone at the outlet is transferred to the carbon inlet. The CT containing resonant pe and CX is one of the given results on the silicon mean side, similar to the acquired input. Data related to carbon output is pulse based, but data related to carbon output from silicon is CT based on r. In the process of communication and information communication, each party contains an association relationship. The part of the interface that improves the precision of the endpoints is the ep and pe at both ends. As r generates a rating, so the degree of response increases during the course of the response and further subsequent communication [27]. As the number of improvement cycles increases, the cognitive distance (CD) between the carbon and silicon agents decreases. Anyone of any age, gender or personality can make up a carbon agent.
In contrast, robots with programmed environments that can interact with humans in real-time form silicon agents. As described in the Cognitive Information Exchange Paradigm from a Carbon-Agent Perspective, this assessment is effectively ancillary data that is ingested into short-term memory (STM) as a result of internal human processes and then into long-term memory (LTM) as Intelligence Quickness (IQ) expertise. Constrained mathematical theories offer an opportunity to change the disciplinary differentiation of knowledge and the cognitive resonance of knowledge and understanding.
4 Neural Resonant Frequency Tracing: A Simulation Model
Cognitive resonance is an integral part of the paradigm and is governed by the paradigm’s mathematical foundations. The stimulus that initiates the exchange of information, the iteration i that grows with frequency s, and the message that s generates within a given frame of i are the three main components of communication between carbon and silicon agents. It allows you to create CDs using the least-squares method by connecting the three main components together.
Least square is the best choice for estimation. Uncertainty is the total squared difference between each sample and the predicted value, similar to cognitive resonance. As shown in Fig. 4, the statistics differ bilaterally. Therefore, a linear model function needs to be fitted, where CT is the slope and CX is the intersection of the line and the i-axis.
The square D of the vertical distance di between two mathematical formulas f should be kept as small as possible. If there are too many resonances, the transmitted data will be corrupted by the silicon agent in the iterations; the information obtained from the coal agent may also be affected due to its limited range of interpretation. From this perspective, the goal is to set the right level of cognitive resonance for you. This formula can be expressed as Eq. (4). Additionally, perceptual resonance can be calculated as a stimulus factor and iterations to calculate the square of the ratio of all repetitions, and CT and CX expand interactively throughout the communication.
Figure 4: Depicts this as a pointer graph depiction of a cognitive resonant gap
This framework can also be applied to regression analysis when the noise term i is replaced by a random variable that converges to zero. Based on the concept of cognitive thought processing, the integration of facts related to cognitive data into the collective intelligence of humans and machines (with cognitive data distance) provides psychological facts from the perspective of carbon compounds.
5 Info-Communications and Cognitive Info-Communications
By demonstrating the identities of patterns that can facilitate cognitive resonances of carbon and silicon agents, an efficient data system will be placed between the two. In order to facilitate proper information exchange [28], a link between human aspects and prototype parts needs to be established in the computational fabric. Data communications are critical in a variety of environments, including business and industry.
From a business decision-making perspective, data needs to be analyzed, understood, and organized according to business needs [29]. Information is created at three levels, each of which is used to build the expertise that underlies business analysis (BA). BA is a set of tools based on cutting edge automation technology that can be used to gain a competitive advantage in different organizations. Examples of tools include various applications, best practices, technologies, and other resources. For example, financial and data information and market awareness are all part of the market. Business analysis has a positive meaning in different stages of DM: decisions based on short-term assessments, strategic decisions and methodologies.
The effectiveness of management decisions at three management levels can be measured using incentive-based information, including context, as described in the silicon-on-carbon (CS) information communication paradigm below. The amount of information provided directly affects the quality of decision-making and its impact [21]. Silicon agents can mimic, analyze and replace management actions that affect an organization. The basic concept of cognitive resonance can be used to develop modeling techniques that aid in the detection of carbon reagents. One possibility is to propose a framework that allows the creation of a personalized user interface and appropriate materials for each carbon agent. They need to develop custom materials using a variety of techniques to improve management understanding, strategy formulation and evaluation, and allow for a high level of customization consistent with hyper-personalization. Through adjustments, the system develops into a flexible system that adapts to multiple management levels and their requirements. The ever-increasing amount of information and the complexity of choice, customization and flexibility over time are key characteristics.
For decision preparation and decision making, data science, machine learning and artificial intelligence are used to generate data, visualizations and administrator platforms. Therefore, the aim is to promote interpretability, openness, interpretability, and understandability of algorithms. As shown in Fig. 5, the cognitive information communication mechanism consisting of the internal information, explanations and variables in Fig. 6 in the carbon-silicon information communication framework [30] is a proof of mathematical theory integrated into the model. According to Wang’s theory, data processing can be related to social reasoning research in multidisciplinary fields, which describes the loading of data obtained from silicon agents (SAs) into carbon agents (CAs).
Figure 5: Info-communication and cognitive Info-communication
Figure 6: Activity diagram depicting verbal & non-verbal carbon-silicon agent framework
6 Theme Discussions and Outcomes
Context-specific observations, hypothesis testing, and infinite learning through information in the framework are the ideal cognitive information exchange systems we propose and develop, regardless of specific characteristics. The non-static properties of neural networks for continuous adaptive systems support carbon-agent learning. It improves the utilization of the synergy of carbon and silicon reagents and allows the value of cognitive resonance to continue to be included in the exchange of information. It conveys complex semantic information and is able to understand it correctly after encountering automatic data processing. Based on modeled variables from human-computer interaction and carbon-silicon information exchange, cognitive resonance frequencies are represented as stimulus and repetition factors in the carbon-agent perspective framework of cognitive information exchange. On this basis, a mathematical model is used to represent cognitive distances and hypergraphs are used to simulate the relationship between topics and ideas.
Mathematical methods, such as research exercises and actual regression measures, can be used for cognitive responses reported in models based on knowledge transfer between carbon and silicon agents. Before applying Cognitive Rich to Silicon and Carbon agents to generate edges, a dataset based on provided parameters must be created to create an ANN. By using this machine learning technique, a cognitive information framework can be constructed to analyze, optimize, augment and replace human cognition with the underlying biological basis for the use of silicon agents with intelligent process automation [31], including computer vision, Artificial Intelligence and Brain Intelligence-based Robotic Process Automation (RPA) [32]. During the investigation, different frameworks provided different intelligence and DM information. We believe this is a focal point that can be used to generate new insights and further research based on them, to ensure that each framework includes not only subfields, but entire processes. Individual frameworks can be further improved; however, they are currently considered the base model.
Over time, the main prototype can be extended to provide deeper insights into specific domains. Strategic management decisions or tactical decisions can be submitted to the DM process. Various variables can be incorporated into the design through quantitative formulations to enhance and improve the accuracy of the underlying model. With tailored materials, context, and reasoning, silicone reagents can aid in decision-making. A thorough internal and external environmental and hazard analysis is required before a decision can be made. Machine intelligence and business intelligence systems have examined data to make practical judgments. Many big data-based forecasts and scenarios are validated, calculated, and developed to help companies make outcome-based decisions about their financial health. These techniques are among the most recently studied cognitive information management tools.
In a research process, different models provide different levels of human reasoning and decision-making details to gain new insights and conduct additional research based on those insights to ensure that all designs include not only sub-domains of the process, but the entire process. The specific designs can be further refined. However, they are currently considered the base model. In the long run, the base model can be extended to gain deeper insights into certain subdomains. Comprehensive macro- and micro-environmental and risk assessments are critical to decision-making. Strategic decisions require knowledge previously validated through artificial intelligence systems. Many big data-based forecasts and scenarios are researched, calculated and developed to help companies make the best decisions based on their specific financial situation. These techniques are one of the latest research solutions for cognitive information systems. A number of partially validated models have been constructed to explain the concept and evolution of physiological and human-based perception systems using the multidisciplinary framework required by this field of research. The construction of these frameworks is highly dependent on human cognitive resonance. A component of the machine touches and performs emotion recognition. In this process, it is crucial that psychological knowledge plays an important role in spreading cognitive resonance. The design provides a better understanding of human intelligence and its activities, while laying a foundation and pathway to guide future research. The limitation of this work is that it builds on the insights of the base model to ensure that all designs include the entire process, not just sub-domains of the process. However, the base model can be extended in future to gain deeper insights into certain subfields including the comprehensive macro- and micro-environmental and risk assessments, which are of interest for decision-making in the long run.
Acknowledgement: The authors extend their appreciation to King Saud University for funding this work through Researchers Supporting Project Number (RSP2022R426), King Saud University, Riyadh, Saudi Arabia.
Funding Statement: This work was supported by King Saud University through Researchers Supporting Project Number (RSP2022R426), King Saud University, Riyadh, Saudi Arabia.
Conflicts of Interest: The authors declare that they have no conflicts of interest to report regarding the present study.
References
1. C. I. Patel, S. Garg, T. Zaveri, A. Banerjee and R. Patel, “Human action recognition using fusion of features for unconstrained video sequences,” Computers & Electrical Engineering, vol. 70, pp. 284–301, 2018. [Google Scholar]
2. I. Lopatovska and I. Arapakis, “Theories, methods and current research on emotions in library and information science, information retrieval and human-computer interaction,” Information Processing & Management, vol. 47, no. 4, pp. 575–592, 2011. [Google Scholar]
3. A. A. Yatoo and A. Sharma, “A novel model for automatic crop disease detection,” in Proc. Int. Conf. on Image Processing, Lecce, Italy, pp. 310–313, 2021. [Google Scholar]
4. P. W. Glimcher, “Indeterminacy in brain and behavior,” Annual Review of Psychology, vol. 56, pp. 25–56, 2005. [Google Scholar]
5. J. S. Lerner, Y. Li, P. Valdesolo and K. S. Kassam, “Emotion and decision making,” Annual Review of Psychology, vol. 66, pp. 799–823, 2015. [Google Scholar]
6. J. I. Gold and M. N. Shadlen, “The neural basis of decision making,” Annual Review of Neuroscience, vol. 30, pp. 535–574, 2007. [Google Scholar]
7. M. N. Shadlen and A. L. Roskies, “The neurobiology of decision-making and responsibility: Reconciling mechanism and mindedness,” Frontiers in Neuroscience, vol. 6, no. 4, pp. 1–12, 2012. [Google Scholar]
8. A. T. Peggy, “Mechanisms linking social ties and support to physical and mental health,” Journal of Health & Social Behavior, vol. 52, no. 2, pp. 145–161, 2011. [Google Scholar]
9. F. Van Overwalle, “Social cognition and the brain: A meta-analysis,” Journal of Health & Social Behavior, vol. 30, no. 3, pp. 829–858, 2009. [Google Scholar]
10. M. Ietswaart, M. Milders, J. R. Crawford, D. Currie and C. L. Scott, “Longitudinal aspects of emotion recognition in patients with traumatic brain injury,” Neuropsychologia, vol. 46, no. 1, pp. 148–159, 2008. [Google Scholar]
11. P. Wolfert, N. Robinson and T. Belpaeme, “A review of evaluation practices of gesture generation in embodied conversational agents,” IEEE Transactions on Human-Machine Systems, vol. 1, no. 3, pp. 1–11, 2022. [Google Scholar]
12. J. J. Wright and D. T. J. Liley, “Dynamics of the brain at global and microscopic scales: Neural networks and the EEG,” Behavioral & Brain Sciences, vol. 19, no. 2, pp. 285–320, 1996. [Google Scholar]
13. E. Daglarli, “Computational modeling of prefrontal cortex for meta-cognition of a humanoid robot,” IEEE Access, vol. 8, pp. 98491–98507, 2020. [Google Scholar]
14. S. Yang, J. Wang, X. Hao, H. Li, X. Wei et al., “BiCoSS: Toward large-scale cognition brain with multigranular neuromorphic architecture,” IEEE Transactions on Neural Networks & Learning Systems, vol. 33, no. 7, pp. 1–15, 2021. [Google Scholar]
15. S. Wetcho and J. Na-Songkhla, “An investigation of pre-service teachers using mobile and wearable devices for emotion recognition and social sharing of emotion to support emotion regulation in mCSCL environments,” Contemporary Educational Technology, vol. 14, no. 2, pp. ep359, 2022. [Google Scholar]
16. B. Molnar and D. Mattyasovszky-Philipp, “An architectural approach to cognitive information system,” in Proc. Int. Conf. on Cognitive Infocommunications, Naples, Italy, pp. 459–462, 2019. [Google Scholar]
17. Y. Wang and G. Ruhe, “The cognitive process of decision making,” International Journal of Cognitive Informatics & Natural Intelligence, vol. 1, no. 2, pp. 73–85, 2007. [Google Scholar]
18. H. Liu, W. Dong, Y. Li, F. Li, J. Geng et al., “An epidermal sEMG tattoo-like patch as a new human-machine interface for patients with loss of voice,” Microsystems & Nanoengineering, vol. 6, no. 1, pp. 1–13, 2020. [Google Scholar]
19. M. Muzammal, R. Talat, A. H. Sodhro and S. Pirbhulal, “A Multi-sensor data fusion enabled ensemble approach for medical data from body sensor networks,” Information Fusion, vol. 53, no. 6, pp. 155–164, 2020. [Google Scholar]
20. M. Zhao, Z. Ji, J. Zhang, Z. Zhu, C. Ye et al., “Assessing distinct cognitive workload levels associated with unambiguous and ambiguous pronoun resolutions in human-machine interactions,” Brain Sciences, vol. 12, no. 3, pp. 1–23, 2022. [Google Scholar]
21. M. Shafiq, M. Ahmad and J. -G. Choi, “Public system usability analysis for the valuation of cognitive burden and interface standardization: A case study of cross-ATM design,” Journal of Organizational Computing & Electronic Commerce, vol. 27, no. 2, pp. 162–196, 2017. [Google Scholar]
22. R. Tadeusiewicz, L. Ogiela and M. R. Ogiela, “Cognitive analysis techniques in business,” in Proc. Int. Conf. on Artificial Intelligence & Soft Computing, Zapopan, Poland, pp. 1027–1039, 2006. [Google Scholar]
23. A. Said, L. C. Félix-Herrán, Y. A. Davizón, C. Hernandez-Santos, R. Soto et al., “An active learning didactic proposal with human-computer interaction in engineering education: A direct current motor case study,” Electronics, vol. 11, no. 7, pp. 1059, 2022. [Google Scholar]
24. G. Cosoli, A. Poli, L. Scalise and S. Spinsante, “Heart rate variability analysis with wearable devices: Influence of artifact correction method on classification accuracy for emotion recognition,” in Proc. Int. Instrumentation & Measurement Technology Conf., Ottawa, Canada, pp. 1–6, 2021. [Google Scholar]
25. R. Duell and J. Treur, “A computational analysis of joint decision making processes,” in Proc. Int. Conf. on Social Informatics, Venice, Italy, pp. 292–308, 2012. [Google Scholar]
26. F. A. Fayaz, A. Malik and A. A. Yatoo, “Cognitive internet of things (CIoT) a success for data collection,” in Proc. Int. Conf. on Image Information Processing, Suzhou, China, vol. 6, pp. 284–287, 2021. [Google Scholar]
27. D. Mattyasovszky-Philipp, A. M. Putnoki and B. Molnár, “The unrepeatable human mind—Challenges in the development of cognitive information systems—What makes a machine human?,” Electronics, vol. 11, no. 3, pp. 1–14, 2022. [Google Scholar]
28. J. Katona, “A review of human–computer interaction and virtual reality research fields in cognitive infocommunications,” Applied Sciences, vol. 11, no. 6, pp. 2646, 2021. [Google Scholar]
29. Y. C. Ko and H. Fujita, “Knowledge-based system acquisition for competitiveness predictions in decision making,” in Proc. Int. Conf. on Intelligent Software Methodologies, Tools & Techniques, Naples, Italy, pp. 265–270, 2013. [Google Scholar]
30. Y. Zhu, X. Chen, K. Chu, X. Wang, Z. Hu et al., “Carbon black/PDMS based flexible capacitive tactile sensor for multi-directional force sensing,” Sensors, vol. 22, no. 2, pp. 1–14, 2022. [Google Scholar]
31. I. H. Sarker, “AI-based modeling: Techniques, applications and research issues towards automation, intelligent and smart systems,” SN Computer Science, vol. 3, no. 2, pp. 1–20, 2022. [Google Scholar]
32. N. R. Mos, “Machine learning and robotic process automation take higher education one step further,” Science & Technology, vol. 25, no. 1, pp. 92–99, 2022. [Google Scholar]
Cite This Article
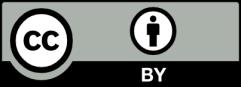
This work is licensed under a Creative Commons Attribution 4.0 International License , which permits unrestricted use, distribution, and reproduction in any medium, provided the original work is properly cited.