Open Access
ARTICLE
Improved Hybrid Deep Collaborative Filtering Approach for True Recommendations
1 Department of Computer Science, The Islamia University of Bahawalpur, Bahawalpur, Pakistan
2 Department of Computer Science, Bahria University Lahore Campus, Lahore, Pakistan
3 Software Engineering Department, Faculty of IT, University of Central Punjab, Lahore, Pakistan
4 Faculty of Computer Science and Information Technology, Universiti Putra Malaysia, Selangor, Malaysia
* Corresponding Author: Nadeem Sarwar. Email:
Computers, Materials & Continua 2023, 74(3), 5301-5317. https://doi.org/10.32604/cmc.2023.032856
Received 31 May 2022; Accepted 17 September 2022; Issue published 28 December 2022
Abstract
Recommendation services become an essential and hot research topic for researchers nowadays. Social data such as Reviews play an important role in the recommendation of the products. Improvement was achieved by deep learning approaches for capturing user and product information from a short text. However, such previously used approaches do not fairly and efficiently incorporate users’ preferences and product characteristics. The proposed novel Hybrid Deep Collaborative Filtering (HDCF) model combines deep learning capabilities and deep interaction modeling with high performance for True Recommendations. To overcome the cold start problem, the new overall rating is generated by aggregating the Deep Multivariate Rating DMR (Votes, Likes, Stars, and Sentiment scores of reviews) from different external data sources because different sites have different rating scores about the same product that make confusion for the user to make a decision, either product is truly popular or not. The proposed novel HDCF model consists of four major modules such as User Product Attention, Deep Collaborative Filtering, Neural Sentiment Classifier, and Deep Multivariate Rating (UPA-DCF + NSC + DMR) to solve the addressed problems. Experimental results demonstrate that our novel model is outperforming state-of-the-art IMDb, Yelp2013, and Yelp2014 datasets for the true top-n recommendation of products using HDCF to increase the accuracy, confidence, and trust of recommendation services.Keywords
Cite This Article
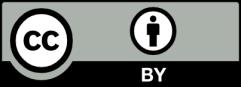