Open Access
ARTICLE
CLGA Net: Cross Layer Gated Attention Network for Image Dehazing
1 Hunan Normal University, Changsha, 410006, China
2 Z-emotion Pty Ltd., Victoria, 3178, Australia
* Corresponding Author: Jingui Huang. Email:
Computers, Materials & Continua 2023, 74(3), 4667-4684. https://doi.org/10.32604/cmc.2023.031444
Received 18 April 2022; Accepted 12 June 2022; Issue published 28 December 2022
Abstract
In this paper, we propose an end-to-end cross-layer gated attention network (CLGA-Net) to directly restore fog-free images. Compared with the previous dehazing network, the dehazing model presented in this paper uses the smooth cavity convolution and local residual module as the feature extractor, combined with the channel attention mechanism, to better extract the restored features. A large amount of experimental data proves that the defogging model proposed in this paper is superior to previous defogging technologies in terms of structure similarity index (SSIM), peak signal to noise ratio (PSNR) and subjective visual quality. In order to improve the efficiency of decoding and encoding, we also describe a fusion residual module and conduct ablation experiments, which prove that the fusion residual is suitable for the dehazing problem. Therefore, we use fusion residual as a fixed module for encoding and decoding. In addition, we found that the traditional defogging model based on the U-net network may cause some information losses in space. We have achieved effective maintenance of low-level feature information through the cross-layer gating structure that better takes into account global and subtle features. We also present the application of our CLGA-Net in challenging scenarios where the best results in both quantity and quality can be obtained. Experimental results indicate that the present cross-layer gating module can be widely used in the same type of network.Keywords
Cite This Article
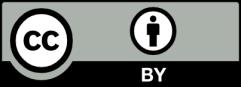