Open Access
ARTICLE
Reconfigurable Sensing Time in Cooperative Cognitive Network Using Machine Learning
1 Department of Electronics Engineering, Korea Polytechnic University, Gyeonggi-do, 15073, Korea
2 Department of Electronics, University of Peshawar, Peshawar, 25120, Pakistan
3 Deparment of Electrical Engineering, Mirpur University of Science of Technology, AJ&K, Mirpur, 10250, Pakistan
4 Department of Electrical Engineering, International Islamic University, Islamabad, 44000, Pakistan
* Corresponding Author: Junsu Kim. Email:
Computers, Materials & Continua 2023, 74(3), 5209-5227. https://doi.org/10.32604/cmc.2023.026945
Received 14 January 2022; Accepted 02 March 2022; Issue published 28 December 2022
Abstract
A cognitive radio network (CRN) intelligently utilizes the available spectral resources by sensing and learning from the radio environment to maximize spectrum utilization. In CRNs, the secondary users (SUs) opportunistically access the primary users (PUs) spectrum. Therefore, unambiguous detection of the PU channel occupancy is the most critical aspect of the operations of CRNs. Cooperative spectrum sensing (CSS) is rated as the best choice for making reliable sensing decisions. This paper employs machine-learning tools to sense the PU channels reliably in CSS. The sensing parameters are reconfigured to maximize the spectrum utilization while reducing sensing error and cost with improved channel throughput. The fine-k-nearest neighbor algorithm (FKNN), employed in this paper, estimates the number of samples based on the nature of the channel under-specific detection and false alarm probability demands. The simulation results reveal that the sensing cost is suppressed by reducing the sensing time and exploiting the traditional fusion rules, validating the effectiveness of the proposed scheme. Furthermore, the global decision made at the fusion center (FC) based on the modified sensing samples, results low energy consumption, higher throughput, and improved detection with low error probabilities.Keywords
Cite This Article
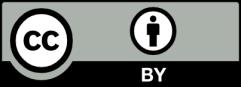
This work is licensed under a Creative Commons Attribution 4.0 International License , which permits unrestricted use, distribution, and reproduction in any medium, provided the original work is properly cited.