Open Access
ARTICLE
Residual Attention Deep SVDD for COVID-19 Diagnosis Using CT Scans
1 Information Systems Department, Faculty of Computers and Information, Mansoura University, Mansoura, 35511, Egypt
2 Computer Science Department, Ibb University, Ibb, Yemen
3 SE Department, Faculty of Computer Sciences and Informatics, Amman Arab University, Amman, 11953, Jordan
* Corresponding Author: Akram Ali Alhadad. Email:
Computers, Materials & Continua 2023, 74(2), 3333-3350. https://doi.org/10.32604/cmc.2023.033413
Received 16 June 2022; Accepted 26 July 2022; Issue published 31 October 2022
Abstract
COVID-19 is the common name of the disease caused by the novel coronavirus (2019-nCoV) that appeared in Wuhan, China in 2019. Discovering the infected people is the most important factor in the fight against the disease. The gold-standard test to diagnose COVID-19 is polymerase chain reaction (PCR), but it takes 5–6 h and, in the early stages of infection, may produce false-negative results. Examining Computed Tomography (CT) images to diagnose patients infected with COVID-19 has become an urgent necessity. In this study, we propose a residual attention deep support vector data description SVDD (RADSVDD) approach to diagnose COVID-19. It is a novel approach combining residual attention with deep support vector data description (DSVDD) to classify the CT images. To the best of our knowledge, we are the first to combine residual attention with DSVDD in general, and specifically in the diagnosis of COVID-19. Combining attention with DSVDD naively may cause model collapse. Attention in the proposed RADSVDD guides the network during training and enables quick learning, residual connectivity prevents vanishing gradients. Our approach consists of three models, each model is devoted to recognizing one certain disease and classifying other diseases as anomalies. These models learn in an end-to-end fashion. The proposed approach attained high performance in classifying CT images into intact, COVID-19, and non-COVID-19 pneumonia. To evaluate the proposed approach, we created a dataset from published datasets and had it assessed by an experienced radiologist. The proposed approach achieved high performance, with the normal model attained sensitivity (0.96–0.98), specificity (0.97–0.99), F1-score (0.97–0.98), and area under the receiver operator curve (AUC) 0.99; the COVID-19 model attained sensitivity (0.97–0.98), specificity (0.97–0.99), F1-score (0.97–0.99), and AUC 0.99; and the non-COVID pneumonia model attained sensitivity (0.97–1), specificity (0.98–0.99), F1-score (0.97–0.99), and AUC 0.99.Keywords
Cite This Article
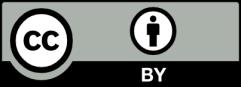