Open Access
ARTICLE
Novel Framework of Segmentation 3D MRI of Brain Tumors
1 Department of Computer Science, Faculty of Computers and Informatics, Zagazig University, Zagazig, Egypt
2 Department of Computer Science, Faculty of Computers and Informatics, Kafrelsheikh University, Kafrelsheikh, Egypt
3 Department of Embedded Network Sys. and Tec., Faculty of Artificial Intelligence, Kafrelsheikh University, Kafrelsheikh, Egypt
4 Computers Engineering and Control Systems Department, Faculty of Engineering, Mansoura University, Mansoura, Egypt
* Corresponding Author: Zainab H. Ali. Email:
Computers, Materials & Continua 2023, 74(2), 3489-3502. https://doi.org/10.32604/cmc.2023.033356
Received 15 June 2022; Accepted 19 August 2022; Issue published 31 October 2022
Abstract
Medical image segmentation is a crucial process for computer-aided diagnosis and surgery. Medical image segmentation refers to portioning the images into small, disjointed parts for simplifying the processes of analysis and examination. Rician and speckle noise are different types of noise in magnetic resonance imaging (MRI) that affect the accuracy of the segmentation process negatively. Therefore, image enhancement has a significant role in MRI segmentation. This paper proposes a novel framework that uses 3D MRI images from Kaggle and applies different diverse models to remove Rician and speckle noise using the best possible noise-free image. The proposed techniques consider the values of Peak Signal to Noise Ratio (PSNR) and the level of noise as inputs to the attention-U-Net model for segmentation of the tumor. The framework has been divided into three stages: removing speckle and Rician noise, the segmentation stage, and the feature extraction stage. The framework presents solutions for each problem at a different stage of the segmentation. In the first stage, the framework uses Vibrational Mode Decomposition (VMD) along with Block-matching and 3D filtering (Bm3D) algorithms to remove the Rician. Afterwards, the most significant Rician noise-free images are passed to the three different methods: Deep Residual Network (DeRNet), Dilated Convolution Auto-encoder Denoising Network (Di-Conv-AE-Net), and Denoising Generative Adversarial Network (DGAN-Net) for removing the speckle noise. VMD and Bm3D have achieved PSNR values for levels of noise (0, 0.25, 0.5, 0.75) for reducing the Rician noise by (35.243, 32.135, 28.214, 24.124) and (36.11, 31.212, 26.215, 24.123) respectively. The framework also achieved PSNR values for removing the speckle noise process for each level as follows: (34.146, 30.313, 28.125, 24.001), (33.112, 29.103, 27.110, 24.194), and (32.113, 28.017, 26.193, 23.121) for DeRNet, Di-Conv-AE-Net, and DGAN-Net, respectively. The experiments that have been conducted have proved the efficiency of the proposed framework against classical filters such as Bilateral, Frost, Kuan, and Lee according to different levels of noise. The attention gate U-Net achieved 94.66 and 95.03 in the segmentation of free noise images in dice and accuracy, respectively.Keywords
Cite This Article
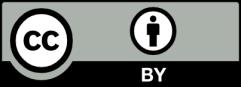
This work is licensed under a Creative Commons Attribution 4.0 International License , which permits unrestricted use, distribution, and reproduction in any medium, provided the original work is properly cited.