Open Access
ARTICLE
Numerical Investigation of Malaria Disease Dynamics in Fuzzy Environment
1 Department of Mathematics, School of Science, University of Management and Technology, Lahore, 54000, Pakistan
2 Department of Mathematics, Cankaya University, Balgat, Ankara, 06530, Turkey
3 Department of Medical Research, China Medical University, Taichung, 40402, Taiwan
4 Institute of Space Sciences, Magurele-Bucharest, 077125, Romania
5 Department of Mathematics and Statistics, University of Lahore, Lahore, 54000, Pakistan
6 Department of Automation, Biomechanics and Mechatronics, Lodz University of Technology, 1/15 Stefanowskiego St., 90-924, Lodz, Poland
7 Department of Mathematics, Faculty of Science & Technology, University of Central Punjab, Lahore, 54000, Pakistan
8 Department of Mathematics, Govt. Maulana Zafar Ali Khan Graduate College Wazirabad, Punjab Higher Education Department (PHED), Lahore, 54000, Pakistan
* Corresponding Author: Fazal Dayan. Email:
Computers, Materials & Continua 2023, 74(2), 2345-2361. https://doi.org/10.32604/cmc.2023.033261
Received 12 June 2022; Accepted 13 July 2022; Issue published 31 October 2022
Abstract
The application of fuzzy theory is vital in all scientific disciplines. The construction of mathematical models with fuzziness is little studied in the literature. With this in mind and for a better understanding of the disease, an SEIR model of malaria transmission with fuzziness is examined in this study by extending a classical model of malaria transmission. The parameters and , being function of the malaria virus load, are considered fuzzy numbers. Three steady states and the reproduction number of the model are analyzed in fuzzy senses. A numerical technique is developed in a fuzzy environment to solve the studied model, which retains essential properties such as positivity and dynamic consistency. Moreover, numerical simulations are carried out to illustrate the analytical results of the developed technique. Unlike most of the classical methods in the literature, the proposed approach converges unconditionally and can be considered a reliable tool for studying malaria disease dynamics.Keywords
Malaria is a deadly infectious disease and occupies a special place in the earliest records of human history. It affects people from the Stone Age to ancient China. People living in tropical regions of sub-Saharan Africa, Asia, and the Amazon are the most affected by malaria. Forty percent of the population in these areas is still at risk of malaria. Malaria is caused by the plasmodium parasite and transmitted between humans through bites of Anopheles mosquitoes [1]. Although malaria has been studied for hundreds of years, it remains a significant public health problem in many countries. According to WHO, 241 million malaria cases and 0.62 million malaria-induced deaths worldwide were reported in 2020. These numbers show that 14 million more cases and 69000 more deaths in 2020 were reported compared to 2019. Mathematical modeling helps to get a comprehensive overview of these outbreaks. The construction of the model and its implementation make it possible to analyze the transmission and control of the malaria spread. Scientists have been ingenious through their efforts in developing different mechanisms and tools that have helped us apply the findings to control malaria transmission appropriately. A wide range of epidemiological models has been formulated, analyzed, and mathematically used to describe the spread and control of malaria. These models are examined from different angles. Otieno et al. proposed a model for the transmission of malaria control measures in Kenya [2]. Initially, constant control parameters were considered. Reproduction number, equilibrium, stability, and sensitivity analysis were also discussed. Then the time-dependent control parameters were considered, and Numerical simulations were performed. Rahman et al. presented SEIR and SEIR-SEI models for malaria transmission [3]. Reproduction number, equilibrium, and stability were also discussed. It was concluded that reducing the contact rate between humans and mosquitoes can control the spread of malaria. The use of active antimalarials is another factor in combating malaria. Treated insecticides and bed nets may help reduce mosquito populations and malaria transmission. Mandal et al. studied some models for malaria information, and their main features have been discussed [4]. Olaniyi developed a seven-dimensional model for malaria transmission [5]. Sensitivity analysis of a malaria transmission model was proposed by Chitnis et al. [6]. Smith et al. examined an individual-based stochastic malaria model [7]. Okosun et al. studied a malaria spread model to investigate the effectiveness and cost-effectiveness of using insecticide-treated mosquito nets, spraying insecticides, and a possible treatment of infected people that blocks transmission to mosquitoes [8]. A malaria transmission model was proposed by Kim et al. and concluded that the use of mosquito reduction strategies is, in some cases, but not always, more effective than personal protection [9]. Agusto et al. presented an SEIR-SEI malaria model by applying optimal control strategies [10]. Port et al. studied the prevention of malaria during pregnancy [11]. Chitnis et al. proposed a model for the malaria spread and discussed the studied model’s reproductive number and equilibrium analysis [12]. Niger and Gumel studied different aspects of a malaria model [13].
The fuzzy theory proposed by Zadeh [14] is extensively used in epidemiology. Verma et al. presented a model of Influenza spread in fuzzy senses [15]. Ortega et al. proposed a fuzzy epidemic model to study the rabies dynamics [16]. Das et al. studied a SIR model and introduced interval numbers as parametric functions [17]. Many other researchers used various approaches using fuzzy theory to prevent strategies to combat deadly diseases [18–22]. Allehiany et al. studied the SIR model in fuzzy senses and proposed different numerical and mathematical schemes to solve the model [23]. Mickens et al. constructed a SEIQR model with fuzzy parameters and developed a fuzzy NSFD method, an extension of the Mickens NSFD theory [24], to solve the studied model [25]. Dayan et al. proposed a fuzzy extension of the cyber consumerism epidemic model and developed an FNSFD scheme [26]. Many researchers studied fuzzy fractional-order dynamical systems [27–30], just to mention a few. SEIR mathematic model helps to understand malaria transmission dynamics, incorporating variables in human and mosquito populations.
Due to varying degrees of susceptibility, exposure, infectivity, and recovery among individuals, the concepts of ‘susceptible,’ ‘exposed,’ ‘infected,’ and ‘recovered’ are uncertain. Uncertainties may arise due to the different degrees of these parameters among population members. Other age groups of the considered population may have different customs, habits, and resistances due to their foreign origin, etc. It is challenging to collect numerical data as a fixed value in many real-life situations, while the date’s range can be decided quickly. Models capable of dealing with the above uncertainties are needed for these different levels of individuals. The fuzzy theory was developed to account for partial truth values ranging from totally true to false to provide robust and inexpensive solutions to real-world problems. It is one of the most powerful tools for dealing with inaccuracies, uncertainties, and partial truths. In this context, mathematical models with fuzziness are more meaningful and powerful. Keeping this in mind, we have extended a classical SEIR model by introducing fuzziness to the model. The malaria transmission rate and malaria-induced death rate of humans have been considered fuzzy numbers as these parameters are functions of the malaria-spreading virus. In the case of a classical system, these parameters are not direct functions of the virus. Therefore, the fuzzy model can be considered more balanced and flexible. Using fuzzy parameters helps us explain malaria transmission in more detail. A mathematical model with fuzziness is formulated and analyzed in this paper that illustrates the transmission dynamics of malaria disease. The novelty of our work is the development, execution, and mathematical investigation of a numerical scheme in fuzzy NSFD settings for a malaria model, particularly with fuzzy parameters.
2 SEIR Model with Fuzzy Parameters
2.1 Definition 1 [31]
A number
where
2.2 Definition 2 [32]
The expected value of a TFN
2.3 Definition 3 [32]
The fuzzy basic reproductive number
We considered the SEIR mathematical model that has been talked about by Arfan et al. [29].
The fuzzy SEIR model corresponding to the above model can be written as
We suppose that the infectious rate of humans
The death rate
2.4 The Fuzzy Basic Reproductive Number
We calculated the reproductive number, denoted by
Case 1: If
Case 2: If
Case 3: If
The basic reproduction number
Now by using definitions 3 and 4 we find
The effect of variation in
Figure 1: The effect of variation in
Case 1: If
where the human population has no malaria, which is called DFE (Disease Free Equilibrium). From a biological point of view, the malaria disease is eradicated when the concentration of the malaria virus is lesser than the least required concentration in the population for carrying the disease.
Case 2: If
and
Case 3: If
where
Points
3.1 Non-Standard Finite Difference (NSFD) Scheme
NSFD scheme for the system (5)–(8) is
Case 1: If
In Fig. 2, the graphical results of the susceptible compartment of the studied model are shown for different values of the step size h for case 1. The results show the convergence and non-negative behavior, which are the main features of the disease dynamic models, as the negativity of any compartment does not make any sense. Fig. 3 depicts the results of the exposed compartment at DFE, and one can see the converging and positive results. The critical feature of these graphs is their consistency at all step sizes. Many classical methods like Euler Maruyama, stochastic Euler, and stochastic RK-4 do not preserve this at large step sizes, as discussed by Rafiq et al. [33]. Figs. 4 and 5 show the compartments of the infected and recovered populations. Again, the results are positive and converging. From this, it can be concluded that the proposed method is reliable for investigating malaria transmission in the human population, even over a long period.
Figure 2: The portion of susceptible populations for case 1 at different step sizes
Figure 3: The portion of exposed populations for case 1 at different step sizes
Figure 4: The portion of infected populations for case 1 at different step sizes
Figure 5: The portion of recovered populations for case 1 at different step sizes
Case 2: If
In Figs. 6 and 7, the results of the susceptible and exposed compartments are shown at the first EE, which shows the convergence for all values of the time step size. We concluded that our proposed method is a reliable tool for studying malaria transmission in the human population.
Figure 6: The portion of susceptible populations for case 2 at different step sizes
Figure 7: The portion of exposed populations for case 2 at different step sizes
Case 3: If
The susceptible and exposed human populations compartments at the second EE are presented in Figs. 8 and 9, respectively. At this stage, the resulting graphs of the NSFD method are not different from the previous cases. The classical standard difference schemes in the literature can cause chaos and misleading fluctuations for some passions of discretization constraints [34–49]. From all these results, we can conclude that the developed method can be considered an appropriate strategy to investigate the spread of malaria in the human population.
Figure 8: The portion of susceptible populations for case 3 at different step sizes
Figure 9: The portion of exposed populations for case 3 at different step sizes
3.2 Stability of the NSFD Scheme
Let
The Jacobian matrix corresponding to the system (34)–(37) is
Jacobian at the DFE is
Eigenvalues of the above jacobian matrix are
The core purpose of this study is to formulate a mathematical and numerical model for a better understanding of the dynamics of malaria diseases. In this work, we started from a classical SEIR malaria disease model and transformed it into a model with fuzzy parameters. The classical models fail when uncertainty in the parameters arises. More reliable models are needed when the problem of human health in the world is considered. The fuzzy theory is the best tool to solve problems containing uncertainty. The use of fuzzy theory makes this study more practical due to its ability to deal with uncertainty. The parameters
Acknowledgement: Thanks to our families and colleagues who supported us morally.
Funding Statement: The authors received no specific funding for this study.
Conflicts of Interest: The authors declare that they have no conflicts of interest to report regarding the present study.
References
- A. A. Cobremeskel and H. E. Krogstad, “Mathematical modeling of endemic transmission,” American Journal of Applied Mathematics, vol. 3, no. 2, pp. 36–46, 2015.
- G. Otieno, J. K. Koske and J. M. Mutiso, “Transmission dynamics and optimal control of malaria in Kenya,” Discrete Dynamics in Nature and Society, vol. 5, no. 1, pp. 01–27, 2016.
- M. A. Rahman, E. N. Osman and I. K. Adu, “Simple mathematical model for malaria transmission,” Journal of Advances in Mathematics and Computer Science, vol. 25, no. 6, pp. 01–24, 2017.
- S. Mandal, R. R. Sarkar and S. Sinha, “Mathematical models of malaria-a review,” Malaria Journal, vol. 10, no. 1, pp. 01–19, 2011.
- S. Olaniyi and O. S. Obabiyi, “Mathematical model for malaria transmission dynamics on human and mosquito population with nonlinear forces of infectious disease,” International Journal of Pure and Applied Mathematics, vol. 88, no. 1, pp. 125–150, 2013.
- N. Chitnis, J. M. Hyman and M. D. Cushing, “Determining important parameters in the spread of malaria through the sensitivity analysis of a mathematical model,” Bulletin of Mathematical Biology, vol. 70, no. 5, pp. 1272–1296, 2008.
- T. Smith, N. Maire, A. Ross, M. Penny, N. Chitnis et al., “Towards a comprehensive simulation model of malaria epidemiology and control,” Parasitology, vol. 135, no. 13, pp. 1507–1516, 2008.
- K. O. Okosun, O. Rachid and N. Marcus, “Optimal control strategies and cost-effectiveness analysis of a malaria model,” Biosystems, vol. 111, no. 2, pp. 83–101, 2013.
- B. N. Kim, K. Nah, C. Chu, S. U. Ryu, Y. H. Kang et al., “Optimal control strategy of plasmodium vivax malaria transmission in Korea,” Osong Public Health and Research Perspectives, vol. 3, no. 3, pp. 128–136, 2012.
- F. B. Agusto, N. Marcus and K. O. Okosun, “Application of optimal control to the epidemiology of malaria,” Electronic Journal of Differential Equations, vol. 8, no. 1, pp. 01–22, 2012.
- A. L. Port, G. Cottrell, C. Dechavanne, V. Briand, A. Bouraima et al., “Prevention of malaria during pregnancy: Assessing the effect of the distribution of IPTp through the national policy in Benin,” American Journal of Tropical Medicine and Hygiene, vol. 84, no. 2, pp. 270–275, 20
- N. Chitnis, J. M. Cushing and J. M. Hyman, “Bifurcation analysis of a mathematical model for malaria transmission,” SIAM Journal on Applied Mathematics, vol. 67, no. 1, pp. 24–45, 2006.
- A. M. Niger and A. B. Gumel, “Mathematical analysis of the role of repeated exposure on malaria transmission dynamics,” Differential Equations and Dynamical Systems, vol. 16, no. 3, pp. 251–287, 2008.
- L. A. Zadeh, “Fuzzy sets,” Information Control, vol. 8, no. 1, pp. 338–353, 1965.
- R. Verma, S. P. Tiwari and R. K. Upadhyay, “Fuzzy modeling for the spread of influenza virus and its possible control,” Computational Ecology and Software, vol. 8, no. 1, pp. 32–45, 2018.
- N. R. S. Ortega, P. C. Sallum and E. Massad, “Fuzzy dynamical systems in epidemic modeling,” Kybernetes, vol. 29, no. 2, pp. 201–218, 2000.
- A. Das and M. Pal, “A mathematical study of an imprecise SIR epidemic model with treatment control,” Journal of Applied Mathematics and Computing, vol. 56, no. 1, pp. 477–500, 2018.
- R. Jafelice, L. C. Barros, R. C. Bassanezei and F. Gomide, “Fuzzy modeling in symptomatic HIV virus-infected population,” Bulletin of Mathematical Biology, vol. 66, no. 6, pp. 1597–1620, 2004.
- P. Panja, S. K. Mondal and J. Chattopadhyay, “Dynamical study in fuzzy threshold dynamics of a cholera epidemic model,” Fuzzy Information and Engineering, vol. 9, no. 3, pp. 381–401, 2017.
- A. Afzal, Z. Ansari, S. Alshahrani, A. K. Raj, M. S. Kuruniyan et al., “Clustering of COVID-19 data for knowledge discovery using c-means and fuzzy c-means,” Results in Physics, vol. 29, no. 1, pp. 01–18, 2021.
- P. K. Mondal, S. Jana, P. Haldar and T. K. Kar, “Dynamical behavior of an epidemic model in a fuzzy transmission,” International Journal of Uncertainty, Fuzziness and Knowledge-Based Systems, vol. 23, no. 5, pp. 651–665, 2015.
- R. Verma, S. P. Tiwari and R. K. Upadhyay, “Transmission dynamics of epidemic spread and outbreak of ebola in West Africa: Fuzzy modeling and simulation,” Journal of Applied Mathematics and Computing, vol. 60, no. 1, pp. 637–671, 2019.
- F. M. Allehiany, F. Dayan, F. F. Al-Harbi, N. Althobaiti, N. Ahmed et al., “Bio-inspired numerical analysis of COVID-19 with fuzzy parameters,” Computers, Materials & Continua, vol. 72, no. 2, pp. 3213–3229, 2022.
- R. E. Mickens, “A fundamental principle for constructing nonstandard finite difference schemes for differential equations,” Journal of Difference Equations and Applications, vol. 1, no. 1, pp. 645–653, 2005.
- F. Dayan, N. Ahmed, M. Rafiq, A. Akgül, A. Raza et al., “Construction and numerical analysis of a fuzzy non-standard computational method for the solution of an SEIQR model of COVID-19 dynamics,” AIMS Mathematics, vol. 7, no. 5, pp. 8449–8470, 2022.
- F. Dayan, M. Rafiq, N. Ahmed, D. Baleanu, A. Raza et al., “Design and numerical analysis of fuzzy nonstandard computational methods for the solution of rumor-base fuzzy epidemic model,” Physica A: Statistical Mechanics and Its Applications, vol. 600, no. 1, pp. 01–19, 2022.
- M. Rahman, M. Arfan, K. Shah and J. F. Gómez-Aguilar, “Investigating a nonlinear dynamical model of COVID-19 disease under fuzzy caputo, random and ABC fractional-order derivative,” Chaos, Solitons & Fractals, vol. 140, no. 20, pp. 01–20, 2020.
- K. Shah, A. R. Seadawy and M. Arfan, “Evaluation of one dimensional fuzzy fractional partial differential equations,” Alexandria Engineering Journal, vol. 59, no. 5, pp. 3347–3353, 2020.
- M. Arfan, K. Shah, T. Abdeljawad and Z. Hammouch, “An efficient tool for solving two-dimensional fuzzy fractional-ordered heat equation,” Numerical Methods for Partial Differential Equations, vol. 37, no. 2, pp. 1407–1418, 2021.
- M. Arfan, K. Shah, A. Ullah and T. Abdeljawad, “Study of fuzzy fractional order diffusion problem under the Mittag-Leffler Kernel Law,” Physica Scripta, vol. 96, no. 7, pp. 01–19, 2021.
- L. C. Barros, R. C. Bassanezi and W. A. Lodwick, “The extension principle of Zadeh and fuzzy numbers,” in A First Course in Fuzzy Logic, Fuzzy Dynamical Systems, and Biomathematics, Berlin, Germany: Springer International Publishing, pp. 23–41, 2017.
- Y. T. Mangongo, J. D. K. Bukweli and J. D. B. Kampempe, “Fuzzy global stability analysis of the dynamics of malaria with fuzzy transmission and recovery rates,” American Journal of Operations Research, vol. 11, no. 6, pp. 257–282, 2021.
- M. Rafiq, A. Ahmadian, A. Raza, D. Baleanu, M. S. Ahsan et al., “Numerical control measures of stochastic malaria epidemic model,” Computers, Materials & Continua, vol. 65, no. 1, pp. 33–51, 2020.
- M. S. Arif, A. Raza, W. Shatanawi, M. Rafiq and M. Bibi, “A stochastic numerical analysis for computer virus model with vertical transmission over the internet,” Computers, Materials Continua, vol. 61, no. 3, pp. 1025–1043, 2019.
- A. Raza, A. Ahmadian, M. Rafiq, S. Salashour, M. Naveed et al., “Modeling the effect of delay strategy on transmission dynamics of HIV/AIDS disease,” Advances in Difference Equations, vol. 663, no. 1, pp. 01–19, 2020.
- A. Raza, A. Ahmadian, M. Rafiq, S. Salahshour and I. R. Laganà, “An analysis of a nonlinear susceptible-exposed-infected-quarantine-recovered pandemic model of a novel coronavirus with delay effect,” Results in Physics, vol. 21, no. 1, pp. 01–07, 2021.
- A. Raza, M. S. Arif, M. Rafiq, M. Bibi, M. Naveed et al., “Numerical treatment for stochastic computer virus model,” Computer Modeling in Engineering and Sciences, vol. 120, no. 2, pp. 445–465, 2019.
- M. S. Arif, A. Raza, K. Abodayeh, M. Rafiq and A. Nazeer, “A numerical efficient technique for the solution of susceptible infected recovered epidemic model,” Computer Modeling in Engineering and Sciences, vol. 124, no. 2, pp. 477–491, 2020.
- W. Shatanawi, A. Raza, M. S. Arif, M. Rafiq, M. Bibi et al., “Essential features preserving dynamics of stochastic dengue model,” Computer Modeling in Engineering and Sciences, vol. 126, no. 1, pp. 201–215, 2021.
- M. A. Noor, A. Raza, M. S. Arif, M. Rafiq, K. S. Nisar et al., “Non-standard computational analysis of the stochastic COVID-19 pandemic model: An application of computational biology,” Alexandria Engineering Journal, vol. 61, no. 1, pp. 619–630, 2021.
- K. Abodayeh, A. Raza, M. S. Arif, M. Rafiq, M. Bibi et al., “Numerical analysis of stochastic vector-borne plant disease model,” Computers, Materials and Continua, vol. 63, no. 1, pp. 65–83, 2020.
- K. Abodayeh, A. Raza, M. S. Arif, M. Rafiq, M. Bibi et al., “Stochastic numerical analysis for impact of heavy alcohol consumption on transmission dynamics of gonorrhoea epidemic,” Computers, Materials and Continua, vol. 62, no. 3, pp. 1125–1142, 2020.
- A. Raza, J. Awrejcewicz, M. Rafiq, N. Ahmed, M. S. Ahsan et al., “Dynamical analysis and design of computational methods for nonlinear stochastic leprosy epidemic model,” Alexandria Engineering Journal, vol. 61, no. 10, pp. 1–15, 2022.
- A. Raza, M. Rafiq, J. Awrejcewicz, N. Ahmed and M. Mohsin, “Stochastic analysis of nonlinear cancer disease model through virotherapy and computational methods,” Mathematics, vol. 10, no. 3, pp. 01–18, 2022.
- A. Raza, “Mathematical modelling of rotavirus disease through efficient methods,” Computers, Materials and Continua, vol. 72, no. 3, pp. 4727–4740, 2022.
- A. Raza, M. Rafiq, J. Awrejcewicz, N. Ahmed and M. Mohsin, “Dynamical analysis of Coronavirus disease with crowding effect, and vaccination: A study of third strain,” Nonlinear Dynamics, vol. 107, no. 4, pp. 3963–3982, 2022.
- A. Raza, J. Awrejcewicz, M. Rafiq and M. Mohsin, “Breakdown of a nonlinear stochastic Nipah virus epidemic model through efficient numerical methods,” Entropy, vol. 23, no. 12, pp. 01–20, 2021.
- M. Rafiq, M. Naveed, Z. Khan, A. Raza, J. Awrejcewicz et al., “Modeling the spread of leishmaniasis disease via delayed analysis,” Alexandria Engineering Journal, vol. 61, no. 12, pp. 11197–11209, 2022.
- A. Raza, Y. M. Chu, M. Y. Bajuri, A. Ahmadian, N. Ahmed et al., “Dynamical and nonstandard computational analysis of heroin epidemic model,” Results in Physics, vol. 34, no. 1, pp. 01–10, 2022.
- H. Sun and R. Grishman, “Employing lexicalized dependency paths for active learning of relation extraction,” Intelligent Automation & Soft Computing, vol. 34, no. 3, pp. 1415–1423, 2022.
Cite This Article
F. Dayan, D. Baleanu, N. Ahmed, J. Awrejcewicz, M. Rafiq et al., "Numerical investigation of malaria disease dynamics in fuzzy environment," Computers, Materials & Continua, vol. 74, no.2, pp. 2345–2361, 2023. https://doi.org/10.32604/cmc.2023.033261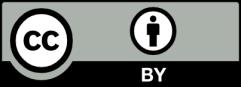