Open Access
ARTICLE
A Neural Study of the Fractional Heroin Epidemic Model
1 Department of Mathematics, Faculty of Science, Khon Kaen University, Khon Kaen, 40002, Thailand
2 Department of Computing and Engineering, Abasyn University Peshawar, Pakistan
3 Department of Mathematics and Statistics, Hazara University, Mansehra, 21120, Pakistan
4 Future Technology Research Center, National Yunlin University of Science and Technology, 123 University Road, Section 3, Douliou, Yunlin, 64002, Taiwan
5 Department of Mathematical Science College of Science United Arab Emirates University Al Ain, Abu Dhabi, UAE
* Corresponding Author: Thongchai Botmart. Email:
Computers, Materials & Continua 2023, 74(2), 4453-4467. https://doi.org/10.32604/cmc.2023.033232
Received 11 June 2022; Accepted 09 September 2022; Issue published 31 October 2022
Abstract
This works intends to provide numerical solutions based on the nonlinear fractional order derivatives of the classical White and Comiskey model (NFD-WCM). The fractional order derivatives have provided authentic and accurate solutions for the NDF-WCM. The solutions of the fractional NFD-WCM are provided using the stochastic computing supervised algorithm named Levenberg-Marquard Backpropagation (LMB) based on neural networks (NNs). This regression approach combines gradient descent and Gauss-Newton iterative methods, which means finding a solution through the sequences of different calculations. WCM is used to demonstrate the heroin epidemics. Heroin has been on-growth world wide, mainly in Asia, Europe, and the USA. It is the fourth foremost cause of death due to taking an overdose in the USA. The nonlinear mathematical system NFD-WCM discusses the overall circumstance of different drug users, such as suspected groups, drug users without treatment, and drug users with treatment. The numerical results of NFD-WCM via LMB-NNs have been substantiated through training, testing, and validation measures. The stability and accuracy are then checked through the statistical tool, such as mean square error (MSE), error histogram, and fitness curves. The suggested methodology’s strength is demonstrated by the high convergence between the reference solutions and the solutions generated by adding the efficacy of a constructed solver LMB-NNs, with accuracy levels ranging from 10−9 to 10−10.Keywords
Heroin is a synthetic opioid from morphine, a natural chemical extracted from the seedlings of several opium poppy plants. These plants grow in Southeast and Southwest Asia, Mexico, and Colombia. Heroin produces in the form of a white or brown powder, and a black sticky material known as black tar heroin. People use heroin by injecting, sniffing, snorting, smoking, or speed-balling by mixing heroin and crack cocaine [1]. The growth of these opium poppies was discovered as evidence in ancient times. Opium poppies were first harvested and consumed around 6,000 years ago. Opium has been a popular narcotic for millennia. During the 1800 s, opium dens were prevalent, and the first drug, morphine, was derived from the opium poppy [2]. Later, heroin became an opiate substance derived from morphine [1–3]. Illicit drug exploitation is destructive to society. Drug users are often potential health extorts to others in order to assess the risk of acquiring and ingesting the drug. These individuals are more susceptible to infection, which can transmit to others due to their damaged immune system. Heroin is an illegal narcotic with major societal consequences. The number of heroin users is increasing every day. Heroin users are affected in many ways, especially physically, culturally, and financially. According to medical science, heroin enters the body, and targets the brain, heart rate, and other cell receptors [4]. Heroin addiction is an attractive trend to study from the perspective of infectious diseases because it is highly ubiquitous. Therefore, various approaches have been adopted to study the different aspects of heroin addiction through mathematical models. In 2007 [5], White and Comiskey constructed the mathematical model for infectious diseases and found through the threshold dynamics that prophylaxis is much better than treatment. After a couple of years, this model was modified by adding the eigenvalue equation, and the Poincare-Bendixson theory was used to obtain the stability of the equilibrium point [6]. Through the delay, global stability for the heroin model was studied by Huang et al. [7]. Wang et al. [8] added the bilinear law to analyze the dynamical behavior of the heroin model. Ma et al. [9] studied the interesting question on media coverage about the spreading of drug addiction. For this purpose, they studied the dynamical behavior based on the 3D drug model using the basic reproduction number. Age structure and nonlinear incidence phenomena were added to the heroin epidemic model using Yang et al. [10,11]. Time distributed delay and nonlinear incidence in the heroin epidemic model is discussed in [12]. The heroin epidemic model with treat age phenomena in mathematical epidemiology was introduced by Botmart et al. [13]. This mathematical model based on the relapse rate means how long time is required for the host in the treatment of heroin addiction. Other different epidemic models have been constructed and tested in various methods in order to capture the dynamics of opioid addiction [14–16]. Fractional calculus is used to model the physical and technical phenomena characterized by the fractional order system (the theory of real/complex order of integral/derivatives). The ideas of fractional calculus were proposed by well-known mathematicians, such as Leibniz, Abel, L’Hopital, Liouville, Riemann, and many others [17,18]. It is found that conventional nonlinear mathematical models, including derivatives integrations fail miserably in complicated situations. In recent years, fractional calculus has been more essential in numerous areas ranging from biomedical sciences to space sciences. Fractional differential system models play a vital role in electricity, chemistry, economics, control theory and automata, image processing, mechanics, chemistry, etc. Mostly, major topics of engineering include thermal diffusivity at semi-infinite, vibration, neutrons-point kinetic model, power-law, continuous-time random walk, die-electric polarization, colored and noise, electromagnetic waves robotics, chaos theory, and in biosciences especially in physiology can be better characterized through the fractional order system [19,20]. Recently, authors have been interested in developing the fractional differential system due to its wide use in almost all fields of engineering and biosciences. In [21–23], the kernel Hilbert space method has been adopted for first-order differential systems, BVPs with two points, integro-differential systems with two-points boundary value problems, and fractional diffusion Gorden Dirichlet time systems in the permeable source. The nonlinear homogenous time fractional gas dynamic system via fractional-order analytical technique is presented in [24]. In the biomedical field, the fractional system is used by Akinlar et al. [25] for the SIRE epidemic disease, Caputo-Fabrizio fractional Des for Rubella disease model [26], and Anthrax disease model in animals [27], for hepatitis B model [28], for the HBV infection model [29], world-known chickenpox disease [30], for infectious diseases [31], psoriasis diseases [32], for HIV/AIDS with treatment model [33] and SIR system of childhood disease [34,35].
An artificial neural network (ANN) is a major cognitive computing paradigm of artificial intelligence. ANNs are evolutionary adaptive in numerous cases based on the information through the network during the learning process, either internally or externally. The learning method entails constructing a set of links (weights) that give a representation compatible with the training set. Stochastic numerical solutions are created to model the ANNs and optimize them by combining of linear and non-linear search algorithms to solve differential problems. Sabir et al. [36] considered artificial neural networks essential for medical revolutions. Epidemic forecasting has been viewed in the light of artificial neural networks by Philemon et al. [37]. For the differentiation of infectious and non-infectious diseases, this paradigm has been used in [38].
The current study aims to solve the mathematical model by using the optimal approach to handle the drug and how a drug pandemic might take hold and spread over a population as well. First, the biological mathematical White and Comiskey model (WCM) involving the chemotaxes and nonlinear diffusion mechanism is discussed. This model is based on the fractional order differential equations, which explain the diffusion of heroin. Then the stochastics computing LMB-NNs technique was taken to solve the designed model. The purpose of introducing stochastic computing is to solve the nonlinear fractional derivative (NFD) WCM. The stochastic solvers have abundant applications to solve the diversity of applications, like food chain models [39,40], periodic differential models [41,42], thermal explosion theory [43,44], smoking differential models [45,46], corneal shape models [47,48], singular differential systems [49,50], Leptospirosis disease models [51,52] and prevention factor in the HIV systems [53,54]. In this paper, the simulations of the WCM are presented using stochastic procedures. The main procedure is given as follows:
• The WCM describes the situation of heroin and non-heroin users but is expected to use it as this group is quite close to the heroin user group.
• The mathematical model of WCM in the form of differential equations is solved through the LMB-NNs techniques.
• A data set for training, testing, and validation based on the LMB-NNs for solving the NFD-WCM.
• The fitness functions are taken in the NNs process, which converts many outputs to a single response.
• Designed Backpropagation based neural networks by providing training and testing dataset LMB is adopted to faster the training.
• The significance of the LMB-NNs technique is illustrated through statistical tool, such as mean square error, error histograms, correlation, regression, and fitting graphs.
The paper is planned to distribute in four sections: Section two delves into the (NFD-WCM) problem formulation and its equilibrium and stability conditions. Section third discusses the LMB for finding the answer to the problem. Section fourth contains the numerical and graphical results. The final portion contains the conclusions.
2 The White-Comiskey Model (WCM)
The WCM consists of three groups of individuals, which are described as:
(i) Susceptible S: those who have never used a drug before.
(ii) Drug user without treatment
(iii) Drug user under-going treatment
The susceptible individual may either smarten up or die and depart or can shift to the drug addict. Drug users continue drug smarten-up or die or start treatment. Drug adductors may smarten up or return the drug. The comprehensive detail of each parameter of (WCM), used to observe the drug’ user group and their treatment process is dispatched in Table 1.
The mathematical model of (NFD-WCM) is constructed as follows,
Let
•
• Drug users can use the treatment during the period of model application.
• Drug users under the treatment process are still using drugs.
• Drug users without treatment group are infectious to adductors in susceptible and treatment.
• Drug users do not infect the susceptible group under treatment.
• Under going treatment can return to non-treatment drug users when contact with them.
• In the population, everyone has the same chance of treatment as other individuals.
The updated form of Eq. (1) with the use of Eq. (1) is given as:
where
Reducing Eq. (4) in
The fractional order differential system of Eq. (5) can be represented as:
The Caputo derivative is used in this study, and the fractional order derivative values have been taken between 0 and 1.
2.1 Free Equilibrium State of Drug
To get drug free equilibrium state, put Eq. (6) equal to zero, given as
From Eq. (7), the drug’s free equilibrium state conditions become as,
The disease free-state Jacobian matrix is provided as follows:
The eigenvalues of the matrix (10) are
For positive endemic equilibria, it is assumed that
The updated form of Eq. (7) by using the above equations becomes as follows:
The Eq. (12) becomes as follows:
where
This part presents the numerical performances of the acquired results of three situations based on the nonlinear fractional differential system of the White-Comiskey heroin model is presented.
Case I: Consider a nonlinear fraction system of heroin model for simulation by taking the suitable values of relevant parameters and corresponding initial conditions.
Let
Case II: Consider
Case III: Consider the parameters
The mathematical form in Eqs. (14)–(16) of the nonlinear fractional system of the White Chomiskey heroin model is investigated through the soft computing supervised LMB-NNs.
The emerging issue of artificial intelligence (AI) is considered a function optimization problem in which we look for the best network parameters to reduce neural network error. Levenberg-Marquardt’s approach easily implements network learning and intelligent computing. This is a method of supervised learning used to supervise the development of some prediction models, decrease notably nonlinear function problems over a few functional parameters, or precisely anticipate the outcomes by specifying a variety of distinct algorithms and patterns. LM technique is also the most prominent method for Feedforward neural networks.
Fig. 1 displays the standard procedure’s structure. First, the prepared LMBs-NNs algorithm is embedded for three cases of NFD-WCM, as illustrated in Table 1. By using 0.001 as the step size for each variable, the total data for LMBs-NNs is 1001 input points found between 0 and 1. Next, the data are randomly distributed for a set of validations, trained, and tested in various ratios to establish the percentage that provides a better grasp of stability and convergence analysis. Finally, the NFD-WCM model is interpreted for several cases using a data set for coupled non-linear higher order fractional differential equations. For LMBs-NNs, the “nftool” is sat, taking the data set for training, testing, and validation as along 7 neurons as, Data set obtained for training is 72%, Data set obtained for testing is 14%, Data set obtained for validation is 14%.
Figure 1: Stepwise frameworks of the nonlinear fractional system of WC heroin model via LMBs-NNs technique. a) mathematical system of heroin model, b) procedure of LMBs-NNs, c) simulation of the proposed model via LMBs-NNs
4 Numerical Results and Discussion
This part presents the numerical results of the proposed NFD-WC-based heroin model through the LMB-NNs algorithm. The fractional order derivatives have been used in many applications [55–61]. The Significant performance of LMBs-NNs has been checked through statistical tools. Comparing of the proposed technique proves the excellent agreement and precision of proposed technique with generated dataset. The structure to compute NFD-WCM is provided in Table 2.
Figs. 2–4 are drawn to show the analysis report of the designed LMBs-NNs technique for the NFD-WC heroin model. The mean square error is measured in Fig. 2 for training, testing, and authentication. The significant performance is found at the points
Figure 2: High Performance of LMBs-NNs for the nonlinear fractional system of WC heroin model using the mean square error tool at suitable epochs for three different cases
Figure 3: State transition for three cases using the nonlinear fractional system of WC heroin model by LMBs-NNs to compute the proposed model
Figure 4: Comparison of outputs using the LMBs-NNs for the nonlinear fractional system of WC heroin model
Figure 5: Results comparability of the function
Figure 6: Results comparability of the function
This study aims to construct the artificial neural networks in conjunction with Levenberg Marquardt backpropagation, i.e., LMBs-NNs, to solve the nonlinear fractional system of the White-Comiskey model of heroin (NFD-WCM). This model depends upon three compartments, susceptible, drug user without treatment, and drug’ user undergoing the treatment and cure. The significance of supervised LMBs-NNs can be described in the following steps of statistical tools:
• The LMBs-NNs are implemented using authentication, testing, and training data samples.
• For training, validation, and testing, the ratios for solving three cases of the NFD-WCM are chosen with samples of 72%, 14%, and 14%, respectively.
• The nonlinear fractional order system of the heroin model is solved by observing the brilliance, quality, precision, and stability of the LMBs-NNs and matching/overlapping the findings.
• The M.S.E convergence graphs are used to check the system’s testing, authentication, and security. M.S.E in the range
• The correlation results are reliable enough to verify the regression processes. The gradient values for each case of the nonlinear fractional model of heroin are obtained using the step size.
Funding Statement: This project is funded by National Research Council of Thailand (NRCT) and Khon Kaen University: N42A650291.
Conflicts of Interest: The authors declare that they have no conflicts of interest to report regarding the present study.
References
1. S. A. Brown, “Stigma towards marijuana users and heroin users,” Journal of Psychoactive Drugs, vol. 47, no. 3, pp. 213–220, 2015. [Google Scholar]
2. M. Rafiq, A. Raza, M. U. Iqbal, Z. Butt, H. A. Naseem et al., “Numerical treatment of stochastic heroin epidemic model,” Advances in Difference Equations, vol. 2019, no. 1, pp. 1–17, 2019. [Google Scholar]
3. B. Fang, X. Z. Li, M. Martcheva and L. M. Cai, “Global asymptotic properties of a heroin epidemic model with treat-age,” Applied Mathematics and Computation, vol. 263, pp. 315–331, 2015. [Google Scholar]
4. A. Din and Y. Li, “Controlling heroin addiction via age-structured modeling,” Advances in Difference Equations, vol. 2020, no. 1, pp. 1–17. 2020. [Google Scholar]
5. E. White and C. Comiskey, “Heroin epidemics, treatment and ODE modelling,” Mathematical Biosciences, vol. 208, no. 1, pp. 312–324, 2007. [Google Scholar]
6. G. Mulone and B. Straughan, “A note on heroin epidemics,” Mathematical Biosciences, vol. 218, no. 2, pp. 138–141, 2009. [Google Scholar]
7. G. Huang and A. Liu, “A note on global stability for a heroin epidemic model with distributed delay,” Applied Mathematics Letters, vol. 26, no. 7, pp. 687–691, 2013. [Google Scholar]
8. X. Wang, J. Yang and X. Li, “Dynamics of a heroin epidemic model with very population,” Applied Mathematics, vol. 2, no. 6, pp. 732–738, 2011. [Google Scholar]
9. M. Ma, S. Liu and J. Li, “Does media coverage influence the spread of drug addiction,” Communications in Nonlinear Science and Numerical Simulation, vol. 50, pp. 169–179, 2017. [Google Scholar]
10. J. Yang, X. Li and F. Zhang, “Global dynamics of a heroin epidemic model with age structure and nonlinear incidence,” International Journal of Biomathematics, vol. 9, no. 3, pp. 1650033, 2016. [Google Scholar]
11. A. Chekroun, M. N. Frioui, T. Kuniya and T. M. Touaoula, “Mathematical analysis of an age structured heroin-cocaine epidemic model,” Discrete & Continuous Dynamical Systems-B, vol. 25, no. 11, pp. 4449, 2020. [Google Scholar]
12. S. Djilali, S. Bentout, T. M. Touaoula, A. Tridane and S. Kumar, “Global behavior of heroin epidemic model with time distributed delay and nonlinear incidence function,” Results in Physics, vol. 31, pp. 104953, 2021. [Google Scholar]
13. T. Botmart, Z. Sabir, M. A. Z. Raja, W. Weera, R. Sadat et al., “A numerical study of the fractional order dynamical nonlinear susceptible infected and quarantine differential model using the stochastic numerical approach,” Fractal and Fractional, vol. 6, no. 3, pp. 1–13, 2022. [Google Scholar]
14. X. Liu and J. Wang, “Epidemic dynamics on a delayed multi-group heroin epidemic model with nonlinear incidence rate,” Journal of Nonlinear Sciences and Applications, vol. 9, no. 5, pp. 2149–2160, 2016. [Google Scholar]
15. L. Zhang and Y. Xing, “Stability analysis of a reaction-diffusion heroin epidemic model,” Complexity, vol. 2020, pp. 1–16, 2020. [Google Scholar]
16. J. Malon, P. Shah, W. Y. Koh, G. Cattabriga, E. Li et al., “Characterizing the demographics of chronic pain patients in the state of Maine using the Maine all payer claims database,” BMC Public Health, vol. 18, no. 1, pp. 1–12, 2018. [Google Scholar]
17. M. I. Troparevsky, S. A. Seminara and M. A. Fabio, “A review on fractional differential equations and a numerical method to solve some boundary value problems,” in Nonlinear Systems-Theoretical Aspects and Recent Applications, 1st ed., vol. 2. London, UK: IntechOpen, pp. 3–22, 2019. [Google Scholar]
18. H. Porpattama, M. Arusamy, G. Anumanthappa, R. Grienggrai, G. Vediyappan et al., “A study on fractional differential equations using the fractional Fourier transform,” Advances in Difference Equations, vol. 2020, no. 1, pp. 1–22, 2020. [Google Scholar]
19. Z. U. A. Zafar, K. Rehan and M. Mushtaq, “Fractional-order scheme for bovine babesiosis disease and tick populations,” Advances in Difference Equations, vol. 2017, no. 1, pp. 1–19, 2017. [Google Scholar]
20. Z. U. A. Zafar, M. Mushtaq and K. Rehan, “A Non-integer order dengue internal transmission model,” Advances in Difference Equations, vol. 2018, no. 1, pp. 1–23, 2018. [Google Scholar]
21. O. A. Arqub and H. Rashaideh, “The RKHS method for numerical treatment for integrodifferential algebraic systems of temporal two-point BVPs,” Neural Computing and Applications, vol. 30, no. 8, pp. 2595–2606, 2018. [Google Scholar]
22. O. A. Arqub, “Numerical solutions of systems of first-order, two-point BVPs based on the reproducing kernel algorithm,” Calcolo, vol. 55, no. 3, pp. 1–28, 2018. [Google Scholar]
23. O. A. Arqub and N. Shawagfeh, “Application of reproducing kernel algorithm for solving dirichlet time-fractional diffusion-gordon types equations in porous media,” Journal of Porous Media, vol. 22, no. 4, pp. 411–434, 2019. [Google Scholar]
24. S. Kumar and M. M. Rashidi, “New analytical method for gas dynamics equation arising in shock fronts,” Computer Physics Communications, vol. 185, no. 7, pp. 1947–1954, 2014. [Google Scholar]
25. M. A. Akinlar, M. Inc, J. F. Gómez-Aguilar and B. Boutarfa, “Solutions of a disease model with fractional white noise,” Chaos, Solitons & Fractals, vol. 137, pp. 109840, 2020. [Google Scholar]
26. D. Baleanu, H. Mohammadi and S. Rezapour, “A mathematical theoretical study of a particular system of Caputo–Fabrizio fractional differential equations for the Rubella disease model,” Advances in Difference Equations, vol. 2020, no. 1, pp. 1–19, 2020. [Google Scholar]
27. S. Rezapour, S. Etemad and H. Mohammadi, “A mathematical analysis of a system of Caputo–Fabrizio fractional differential equations for the anthrax disease model in animals,” Advances in Difference Equations, vol. 2020, no. 1, pp. 1–30, 2020. [Google Scholar]
28. L. C. Cardoso, R. F. Camargo, F. L. P. dos Santos and J. P. C. Dos Santos, “Global stability analysis of a fractional differential system in hepatitis B,” Chaos, Solitons & Fractals, vol. 143, pp. 110619, 2021. [Google Scholar]
29. J. Danane, K. Allali and Z. Hammouch, “Mathematical analysis of a fractional differential model of HBV infection with antibody immune response,” Chaos, Solitons & Fractals, vol. 136, pp. 109787, 2020. [Google Scholar]
30. S. Qureshi and A. Yusuf, “Modeling chickenpox disease with fractional derivatives: From Caputo to Atangana-Baleanu,” Chaos, Solitons & Fractals, vol. 122, pp. 111–118, 2019. [Google Scholar]
31. B. Ghanbari, “Chaotic behaviors of the prevalence of an infectious disease in a prey and predator system using fractional derivatives,” Mathematical Methods in the Applied Sciences, vol. 44, no. 13, pp. 9998–10013, 2021. [Google Scholar]
32. X. Cao, A. Datta, F. Al Basir and P. K. Roy, “Fractional-order model of the disease psoriasis: A control based mathematical approach,” Journal of Systems Science and Complexity, vol. 29, no. 6, pp. 1565–1584, 2016. [Google Scholar]
33. E. J. Moore, S. Sirisubtawee and S. Koonprasert, “A Caputo–Fabrizio fractional differential equation model for HIV/AIDS with treatment compartment,” Advances in Difference Equations, vol. 2019, no. 1, pp. 1–20, 2019. [Google Scholar]
34. H. M. Srivastava and H. Günerhan, “Analytical and approximate solutions of fractional-order susceptible-infected-recovered epidemic model of childhood disease,” Mathematical Methods in the Applied Sciences, vol. 42, no. 3, pp. 935–941, 2019. [Google Scholar]
35. F. Haq, M. Shahzad, S. Muhammad and H. A. Wahab, “Numerical analysis of fractional order epidemic model of childhood diseases,” Discrete Dynamics in Nature and Society, vol. 2017, pp. 1–7, 2017. [Google Scholar]
36. Z. Sabir, T. Botmart, M. A. Z. Raja, R. Sadat, M. R. Ali et al., “Artificial neural network scheme to solve the nonlinear influenza disease model,” Biomedical Signal Processing and Control, vol. 75, no. 103594, pp. 1–13, 2022. [Google Scholar]
37. M. D. Philemon, Z. Ismail and J. Dare, “A review of epidemic forecasting using artificial neural networks,” International Journal of Epidemiologic Research, vol. 6, no. 3, pp. 132–143, 2019. [Google Scholar]
38. P. H. Dakappa, K. Prasad, S. B. Rao, G. Bolumbu, G. K. Bhat et al., “Classification of infectious and noninfectious diseases using artificial neural networks from 24-h continuous tympanic temperature data of patients with undifferentiated fever,” Critical Reviews in Biomedical Engineering, vol. 46, no. 2, pp. 173–183, 2018. [Google Scholar]
39. Z. Sabir, “Stochastic numerical investigations for nonlinear three-species food chain system,” International Journal of Biomathematics, vol. 15, no. 4, pp. 2250005, 2022. [Google Scholar]
40. Z. Sabir, M. R. Ali and R. Sadat, “Gudermannian neural networks using the optimization procedures of genetic algorithm and active set approach for the three-species food chain nonlinear model,” Journal of Ambient Intelligence and Humanized Computing, vol. 13, pp. 1–10, 2022. [Google Scholar]
41. Z. Sabir, D. Baleanu, M. R. Ali and R. Sadat, “A novel computing stochastic algorithm to solve the nonlinear singular periodic boundary value problems,” International Journal of Computer Mathematics, vol. 99,. pp. 1–14, 2022. [Google Scholar]
42. Z. Sabir, C. M. Khalique, M. A. Z. Raja and D. Baleanu, “Evolutionary computing for nonlinear singular boundary value problems using neural network, genetic algorithm and active-set algorithm,” The European Physical Journal Plus, vol. 136, no. 2, pp. 1–19, 2021. [Google Scholar]
43. Z. Sabir, “Neuron analysis through the swarming procedures for the singular two-point boundary value problems arising in the theory of thermal explosion,” The European Physical Journal Plus, vol. 137, no. 5, pp. 638, 2022. [Google Scholar]
44. Z. Sabir, H. A. Wahab, M. R. Ali and R. Sadat, “Neuron analysis of the two-point singular boundary value problems arising in the thermal explosion’s theory,” Neural Processing Letters, vol. 54,. pp. 1–28, 2022. [Google Scholar]
45. T. Saeed, Z. Sabir, M. S. Alhodaly, H. H. Alsulami and Y. G. Sánchez, “An advanced heuristic approach for a nonlinear mathematical based medical smoking model,” Results in Physics, vol. 32, pp. 105137, 2022. [Google Scholar]
46. Z. Sabir, M. A. Z. Raja, A. S. Alnahdi, M. B. Jeelani and M. A. Abdelkawy, “Numerical investigations of the nonlinear smoke model using the Gudermannian neural networks,” Mathematical Biosciences and Engineering, vol. 19, no. 1, pp. 351–370, 2022. [Google Scholar]
47. B. Wang, J. F. Gomez-Aguilar, Z. Sabir, M. A. Z. Raja, W. F. Xia et al., “Numerical computing to solve the nonlinear corneal system of eye surgery using the capability of Morlet wavelet artificial neural networks,” Fractals, vol. 30, no. 5, pp. 1–19, 2022. [Google Scholar]
48. M. Umar, F. Amin, H. A. Wahab and D. Baleanu, “Unsupervised constrained neural network modeling of boundary value corneal model for eye surgery,” Applied Soft Computing, vol. 85, pp. 105826, 2019. [Google Scholar]
49. Z. Sabir, H. A. Wahab, S. Javeed and H. M. Baskonus, “An efficient stochastic numerical computing framework for the nonlinear higher order singular models,” Fractal and Fractional, vol. 5, no. 4, pp. 1–14, 2021. [Google Scholar]
50. Z. Sabir and H. A. Wahab, “Evolutionary heuristic with Gudermannian neural networks for the nonlinear singular models of third kind,” Physica Scripta, vol. 96, no. 12, pp. 125261, 2021. [Google Scholar]
51. K. Mukdasai, Z. Sabir, M. A. Z. Raja, R. Sadat, M. R. Ali et al., “A numerical simulation of the fractional order Leptospirosis model using the supervise neural network,” Alexandria Engineering Journal, vol. 61, no. 12, pp. 12431–12441, 2022. [Google Scholar]
52. T. Botmart, Z. Sabir, M. A. Z. Raja, M. R. Ali, R. Sadat et al., “A hybrid swarming computing approach to solve the biological nonlinear Leptospirosis system,” Biomedical Signal Processing and Control, vol. 77, pp. 103789, 2022. [Google Scholar]
53. M. Umar, Z. Sabir, M. A. Z. Raja, H. M. Baskonus, S. W. Yao et al., “A novel study of Morlet neural networks to solve the nonlinear HIV infection system of latently infected cells,” Results in Physics, vol. 25, pp. 104235, 2021. [Google Scholar]
54. Z. Sabir, M. Umar, M. A. Z. Raja, H. M. Baskonus and W. Gao, “Designing of Morlet wavelet as a neural network for a novel prevention category in the HIV system,” International Journal of Biomathematics, vol. 15, no. 4, pp. 2250012, 2022. [Google Scholar]
55. M. Izadi, Ş Yüzbaşı and W. Adel, “Two novel bessel matrix techniques to solve the squeezing flow problem between infinite parallel plates,” Computational Mathematics and Mathematical Physics, vol. 61, no. 12, pp. 2034–2053, 2021. [Google Scholar]
56. P. Veeresha, N. S. Malagi, D. G. Prakasha and H. M. Baskonus, “An efficient technique to analyze the fractional model of vector-borne diseases,” Physica Scripta, vol. 97, pp. 1–19, 2022. [Google Scholar]
57. R. Jan and Ş Yüzbaşı, “Dynamical behaviour of hiv infection with the influence of variable source term through galerkin method,” Chaos, Solitons & Fractals, vol. 152, pp. 111429, 2021. [Google Scholar]
58. A. Kumar, A. Prakash and H. M. Baskonus, “The epidemic COVID-19 model via Caputo-Fabrizio fractional operator,” Waves in Random and Complex Media, vol. 32,. pp. 1–15, 2022. [Google Scholar]
59. A. M. R. Elsonbaty, Z. Sabir, R. Ramaswamy and W. Adel, “Dynamical analysis of a novel discrete fractional SITRS model for COVID-19,” Fractals, vol. 29, no. 8, pp. 2140035, 2021. [Google Scholar]
60. S. Boulaaras, R. Jan, A. Khan and M. Ahsan, “Dynamical analysis of the transmission of dengue fever via caputo-fabrizio fractional derivative,” Chaos, Solitons & Fractals: X, vol. 8, pp. 1–13, 2022. [Google Scholar]
61. A. Ciancio, G. Yel, A. Kumar, H. M. Baskonus and E. Ilhan, “On the complex mixed dark-bright wave distributions to some conformable nonlinear integrable models,” Fractals, vol. 30, no. 1, pp. 1–14, 2022. [Google Scholar]
Cite This Article
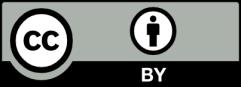
This work is licensed under a Creative Commons Attribution 4.0 International License , which permits unrestricted use, distribution, and reproduction in any medium, provided the original work is properly cited.