Open Access
ARTICLE
Log Anomaly Detection Based on Hierarchical Graph Neural Network and Label Contrastive Coding
School of Cyber Science and Engineering, Sichuan University, Chengdu, 610000, China
* Corresponding Author: Yijia Xu. Email:
Computers, Materials & Continua 2023, 74(2), 4099-4118. https://doi.org/10.32604/cmc.2023.033124
Received 08 June 2022; Accepted 02 September 2022; Issue published 31 October 2022
Abstract
System logs are essential for detecting anomalies, querying faults, and tracing attacks. Because of the time-consuming and labor-intensive nature of manual system troubleshooting and anomaly detection, it cannot meet the actual needs. The implementation of automated log anomaly detection is a topic that demands urgent research. However, the prior work on processing log data is mainly one-dimensional and cannot profoundly learn the complex associations in log data. Meanwhile, there is a lack of attention to the utilization of log labels and usually relies on a large number of labels for detection. This paper proposes a novel and practical detection model named LCC-HGLog, the core of which is the conversion of log anomaly detection into a graph classification problem. Semantic temporal graphs (STG) are constructed by extracting the raw logs’ execution sequences and template semantics. Then a unique graph classifier is used to better comprehend each STG’s semantic, sequential, and structural features. The classification model is trained jointly by graph classification loss and label contrastive loss. While achieving discriminability at the class-level, it increases the fine-grained identification at the instance-level, thus achieving detection performance even with a small amount of labeled data. We have conducted numerous experiments on real log datasets, showing that the proposed model outperforms the baseline methods and obtains the best all-around performance. Moreover, the detection performance degrades to less than 1% when only 10% of the labeled data is used. With 200 labeled samples, we can achieve the same or better detection results than the baseline methods.Keywords
Cite This Article
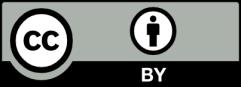
This work is licensed under a Creative Commons Attribution 4.0 International License , which permits unrestricted use, distribution, and reproduction in any medium, provided the original work is properly cited.