Open Access
ARTICLE
Proposed Framework for Detection of Breast Tumors
1 Department of Computer Science, Faculty of Computers and Informatics, Kafrelsheikh University, Kafrelsheikh, Egypt
2 Department of Computer Science, Faculty of Computers and Informatics, Zagazig University, Zagazig, Egypt
* Corresponding Author: Mostafa Elbaz. Email:
Computers, Materials & Continua 2023, 74(2), 2927-2944. https://doi.org/10.32604/cmc.2023.033111
Received 08 June 2022; Accepted 01 August 2022; Issue published 31 October 2022
Abstract
Computer vision is one of the significant trends in computer science. It plays as a vital role in many applications, especially in the medical field. Early detection and segmentation of different tumors is a big challenge in the medical world. The proposed framework uses ultrasound images from Kaggle, applying five diverse models to denoise the images, using the best possible noise-free image as input to the U-Net model for segmentation of the tumor, and then using the Convolution Neural Network (CNN) model to classify whether the tumor is benign, malignant, or normal. The main challenge faced by the framework in the segmentation is the speckle noise. It’s is a multiplicative and negative issue in breast ultrasound imaging, because of this noise, the image resolution and contrast become reduced, which affects the diagnostic value of this imaging modality. As result, speckle noise reduction is very vital for the segmentation process. The framework uses five models such as Generative Adversarial Denoising Network (DGAN-Net), Denoising U-Shaped Net (D-U-NET), Batch Renormalization U-Net (Br-U-NET), Generative Adversarial Network (GAN), and Nonlocal Neutrosophic of Wiener Filtering (NLNWF) for reducing the speckle noise from the breast ultrasound images then choose the best image according to peak signal to noise ratio (PSNR) for each level of speckle-noise. The five used methods have been compared with classical filters such as Bilateral, Frost, Kuan, and Lee and they proved their efficiency according to PSNR in different levels of noise. The five diverse models are achieved PSNR results for speckle noise at level (0.1, 0.25, 0.5, 0.75), (33.354, 29.415, 27.218, 24.115), (31.424, 28.353, 27.246, 24.244), (32.243, 28.42, 27.744, 24.893), (31.234, 28.212, 26.983, 23.234) and (33.013, 29.491, 28.556, 25.011) for DGAN, Br-U-NET, D-U-NET, GAN and NLNWF respectively. According to the value of PSNR and level of speckle noise, the best image passed for segmentation using U-Net and classification using CNN to detect tumor type. The experiments proved the quality of U-Net and CNN in segmentation and classification respectively, since they achieved 95.11 and 95.13 in segmentation and 95.55 and 95.67 in classification as dice score and accuracy respectively.Keywords
Cite This Article
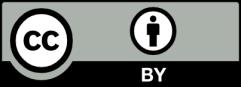
This work is licensed under a Creative Commons Attribution 4.0 International License , which permits unrestricted use, distribution, and reproduction in any medium, provided the original work is properly cited.