Open Access
ARTICLE
Natural Language Processing with Optimal Deep Learning-Enabled Intelligent Image Captioning System
1 Department of Information Systems, College of Computer and Information Sciences, Princess Nourah bint Abdulrahman University, P.O.Box 84428, Riyadh, 11671, Saudi Arabia
2 Department of Computer Sciences, College of Computer and Information Sciences, Princess Nourah bint Abdulrahman University, P.O.Box 84428, Riyadh, 11671, Saudi Arabia
3 Department of Computer Sciences, College of Computing and Information System, Umm Al-Qura University, Saudi Arabia
4 Department of Computer Science, College of Sciences and Humanities-Aflaj, Prince Sattam bin Abdulaziz University, Saudi Arabia
5 Department of Computer Science, Faculty of Computers and Information Technology, Future University in Egypt, New Cairo, 11835, Egypt
6 Department of Computer and Self Development, Preparatory Year Deanship, Prince Sattam bin Abdulaziz University, AlKharj, Saudi Arabia
* Corresponding Author: Mesfer Al Duhayyim. Email:
Computers, Materials & Continua 2023, 74(2), 4435-4451. https://doi.org/10.32604/cmc.2023.033091
Received 07 June 2022; Accepted 12 July 2022; Issue published 31 October 2022
Abstract
The recent developments in Multimedia Internet of Things (MIoT) devices, empowered with Natural Language Processing (NLP) model, seem to be a promising future of smart devices. It plays an important role in industrial models such as speech understanding, emotion detection, home automation, and so on. If an image needs to be captioned, then the objects in that image, its actions and connections, and any silent feature that remains under-projected or missing from the images should be identified. The aim of the image captioning process is to generate a caption for image. In next step, the image should be provided with one of the most significant and detailed descriptions that is syntactically as well as semantically correct. In this scenario, computer vision model is used to identify the objects and NLP approaches are followed to describe the image. The current study develops a Natural Language Processing with Optimal Deep Learning Enabled Intelligent Image Captioning System (NLPODL-IICS). The aim of the presented NLPODL-IICS model is to produce a proper description for input image. To attain this, the proposed NLPODL-IICS follows two stages such as encoding and decoding processes. Initially, at the encoding side, the proposed NLPODL-IICS model makes use of Hunger Games Search (HGS) with Neural Search Architecture Network (NASNet) model. This model represents the input data appropriately by inserting it into a predefined length vector. Besides, during decoding phase, Chimp Optimization Algorithm (COA) with deeper Long Short Term Memory (LSTM) approach is followed to concatenate the description sentences produced by the method. The application of HGS and COA algorithms helps in accomplishing proper parameter tuning for NASNet and LSTM models respectively. The proposed NLPODL-IICS model was experimentally validated with the help of two benchmark datasets. A widespread comparative analysis confirmed the superior performance of NLPODL-IICS model over other models.Keywords
Cite This Article
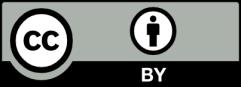
This work is licensed under a Creative Commons Attribution 4.0 International License , which permits unrestricted use, distribution, and reproduction in any medium, provided the original work is properly cited.