Open Access
ARTICLE
Automated Deep Learning Driven Crop Classification on Hyperspectral Remote Sensing Images
1 Department of Computer Science, College of Sciences and Humanities-Aflaj, Prince Sattam bin Abdulaziz University, Saudi Arabia
2 Department of Information Systems, College of Computer and Information Sciences, Princess Nourah bint Abdulrahman University, P. O. Box 84428, Riyadh, 11671, Saudi Arabia
3 Department of Computer Science, College of Science & Art at Mahayil, King Khalid University, Saudi Arabia
4 Department of Industrial Engineering, College of Engineering at Alqunfudah, Umm Al-Qura University, Saudi Arabia
5 Department of Electrical Engineering, Faculty of Engineering & Technology, Future University in Egypt, New Cairo, 11845, Egypt
6 Department of Computer and Self Development, Preparatory Year Deanship, Prince Sattam bin Abdulaziz University, AlKharj, Saudi Arabia
* Corresponding Author: Mesfer Al Duhayyim. Email:
Computers, Materials & Continua 2023, 74(2), 3167-3181. https://doi.org/10.32604/cmc.2023.033054
Received 06 June 2022; Accepted 12 July 2022; Issue published 31 October 2022
Abstract
Hyperspectral remote sensing/imaging spectroscopy is a novel approach to reaching a spectrum from all the places of a huge array of spatial places so that several spectral wavelengths are utilized for making coherent images. Hyperspectral remote sensing contains acquisition of digital images from several narrow, contiguous spectral bands throughout the visible, Thermal Infrared (TIR), Near Infrared (NIR), and Mid-Infrared (MIR) regions of the electromagnetic spectrum. In order to the application of agricultural regions, remote sensing approaches are studied and executed to their benefit of continuous and quantitative monitoring. Particularly, hyperspectral images (HSI) are considered the precise for agriculture as they can offer chemical and physical data on vegetation. With this motivation, this article presents a novel Hurricane Optimization Algorithm with Deep Transfer Learning Driven Crop Classification (HOADTL-CC) model on Hyperspectral Remote Sensing Images. The presented HOADTL-CC model focuses on the identification and categorization of crops on hyperspectral remote sensing images. To accomplish this, the presented HOADTL-CC model involves the design of HOA with capsule network (CapsNet) model for generating a set of useful feature vectors. Besides, Elman neural network (ENN) model is applied to allot proper class labels into the input HSI. Finally, glowworm swarm optimization (GSO) algorithm is exploited to fine tune the ENN parameters involved in this article. The experimental result scrutiny of the HOADTL-CC method can be tested with the help of benchmark dataset and the results are assessed under distinct aspects. Extensive comparative studies stated the enhanced performance of the HOADTL-CC model over recent approaches with maximum accuracy of 99.51%.Keywords
Cite This Article
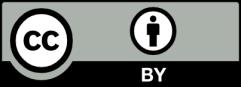