Open Access
ARTICLE
Hybrid Dipper Throated and Grey Wolf Optimization for Feature Selection Applied to Life Benchmark Datasets
1 Department of Computer Sciences, College of Computer and Information Sciences, Princess Nourah bint Abdulrahman University, P.O. Box 84428, Riyadh, 11671, Saudi Arabia
2 Department of Communications and Electronics, Delta Higher Institute of Engineering and Technology, Mansoura, 35111, Egypt
3 Faculty of Artificial Intelligence, Delta University for Science and Technology, Mansoura, 35712, Egypt
4 Department of System Programming, South Ural State University, Chelyabinsk, 454080, Russia
5 Computer Engineering and Control Systems Department, Faculty of Engineering, Mansoura University, Mansoura, 35516, Egypt
6 Department of Computer Science, Faculty of Computer and Information Sciences, Ain Shams University, Cairo, 11566, Egypt
7 Department of Computer Science, College of Computing and Information Technology, Shaqra University, 11961, Saudi Arabia
8 Department of Computer Science, Faculty of Specific Education, Mansoura University, Egypt
* Corresponding Author: Faten Khalid Karim. Email:
Computers, Materials & Continua 2023, 74(2), 4531-4545. https://doi.org/10.32604/cmc.2023.033042
Received 05 June 2022; Accepted 12 July 2022; Issue published 31 October 2022
Abstract
Selecting the most relevant subset of features from a dataset is a vital step in data mining and machine learning. Each feature in a dataset has 2n possible subsets, making it challenging to select the optimum collection of features using typical methods. As a result, a new metaheuristics-based feature selection method based on the dipper-throated and grey-wolf optimization (DTO-GW) algorithms has been developed in this research. Instability can result when the selection of features is subject to metaheuristics, which can lead to a wide range of results. Thus, we adopted hybrid optimization in our method of optimizing, which allowed us to better balance exploration and harvesting chores more equitably. We propose utilizing the binary DTO-GW search approach we previously devised for selecting the optimal subset of attributes. In the proposed method, the number of features selected is minimized, while classification accuracy is increased. To test the proposed method’s performance against eleven other state-of-the-art approaches, eight datasets from the UCI repository were used, such as binary grey wolf search (bGWO), binary hybrid grey wolf, and particle swarm optimization (bGWO-PSO), bPSO, binary stochastic fractal search (bSFS), binary whale optimization algorithm (bWOA), binary modified grey wolf optimization (bMGWO), binary multiverse optimization (bMVO), binary bowerbird optimization (bSBO), binary hysteresis optimization (bHy), and binary hysteresis optimization (bHWO). The suggested method is superior and successful in handling the problem of feature selection, according to the results of the experiments.Keywords
Cite This Article
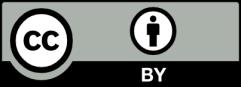
This work is licensed under a Creative Commons Attribution 4.0 International License , which permits unrestricted use, distribution, and reproduction in any medium, provided the original work is properly cited.