Open Access
ARTICLE
Ontology-Based News Linking for Semantic Temporal Queries
1 Faculty of Computing, Riphah International University, I-14 Campus, Islamabad, 44000, Pakistan
2 Department of Computer Science Zabsolutions, SZABIST, Islamabad, Pakistan
3 Department of Computer Science, COMSATS University Islamabad, Islamabad, 45550, Pakistan
4 Industrial Engineering Department, College of Engineering, King Saud University, P.O. Box 800, Riyadh, 11421, Saudi Arabia
5 Department of Information Technology, University of the Punjab Gujranwala Campus, Gujranwala, 52250, Pakistan
6 Department of Information and Communication Engineering, Yeungnam University, Gyeongsan, 38541, Korea
* Corresponding Author: Muhammad Shafiq. Email:
Computers, Materials & Continua 2023, 74(2), 3913-3929. https://doi.org/10.32604/cmc.2023.033001
Received 03 June 2022; Accepted 09 August 2022; Issue published 31 October 2022
Abstract
Daily newspapers publish a tremendous amount of information disseminated through the Internet. Freely available and easily accessible large online repositories are not indexed and are in an un-processable format. The major hindrance in developing and evaluating existing/new monolingual text in an image is that it is not linked and indexed. There is no method to reuse the online news images because of the unavailability of standardized benchmark corpora, especially for South Asian languages. The corpus is a vital resource for developing and evaluating text in an image to reuse local news systems in general and specifically for the Urdu language. Lack of indexing, primarily semantic indexing of the daily news items, makes news items impracticable for any querying. Moreover, the most straightforward search facility does not support these unindexed news resources. Our study addresses this gap by associating and marking the newspaper images with one of the widely spoken but under-resourced languages, i.e., Urdu. The present work proposed a method to build a benchmark corpus of news in image form by introducing a web crawler. The corpus is then semantically linked and annotated with daily news items. Two techniques are proposed for image annotation, free annotation and fixed cross examination annotation. The second technique got higher accuracy. Build news ontology in protégé using Ontology Web Language (OWL) language and indexed the annotations under it. The application is also built and linked with protégé so that the readers and journalists have an interface to query the news items directly. Similarly, news items linked together will provide complete coverage and bring together different opinions at a single location for readers to do the analysis themselves.Keywords
With the exponential growth of the World Wide Web (WWW) data in the form of text images have also increased significantly. The noticeable addition on the web in text images is not much envisioned, especially in the Urdu language. Urdu, which is spoken among 172 million people famous as Asian, was not given much prominence in the local language. Benchmark corpus like English or other favorite languages is critical in nominating a well-structured language. Many datasets are publicly available in the English language, like METER (MEasuring TExt Reuse) corpus, to figure out and process the text image. Moreover, in English text images Optical Character Reader (OCR) is freely available and used to query and retrieve the image in electronic form.
In Pakistan, the daily newspapers in the Urdu language publish a massive amount of data over the internet that is text in image form. Various news agencies like Aaj, Express, Nawa-e-Waqt, and Jang upload newspapers in electronic form on their websites daily [1–3]. These website repositories saving newspapers are not centralized. The same news is published in different ways in numerous repositories, which is the consequence of the redundancy of news. This Urdu news published daily and took a huge part of the web is not used efficiently due to lake of standard benchmark corpora. English newspapers are very well linked and indexed [4]. Readers can easily query and get the required result without wasting time. There is a dire need for a mechanism to link the Urdu news in a manner that the readers and journalists can query it. Before this, online Urdu news is not indexed. Notably, semantic indexing is missing in the news, which is mandatory in today’s environment.
A group of modules and tools that allow the machines to infer the semantic or sense of information on WWW is referred to as the semantic web [5–7]. The semantic web used in search inclines to improve the accuracy of the search results. A foremost technique used in the semantic web is centered on ontology.
The newspaper online is well-indexed in English, like “The news,” which can be directly queried because of well-indexed [8–11]. Urdu corpus generation is vital to improving the search and retrieving relevant results. Working on developing the Urdu corpus is a noteworthy approach to developing the Urdu language. Readers save a lot of search time in semantic searching because of directly getting the relevant result precisely and in an organized way instead of web pages. This research tackles the syntactic search by providing a user interface for temporal queries and retrieves the rank results instead of web pages [12–15]. Moreover, the Urdu news (Unews) engine also returns news images for the readers when they query the temporal data based on time.
A substantial amount of Urdu news information is published daily. These news agencies broadcast data online in text image form. The electronic versions of Urdu newspapers are un-indexed and in an un-processable format. Lack of indexing, especially semantic indexing of the daily news items, makes news items impracticable for any querying. Moreover, these un-indexed news sources do not support even the most straight-forward search facility [16–20]. There is a dire need for some technique to query these vast repositories. We aim to address the identified problem by generating a semantic search technique to query the news repositories. A medium-sized corpus is generated by collecting news items from various news agencies in Pakistan. Latterly, semantic annotation of the news item concerning Free Annotation (FA) and news ontology [20–22]. Annotations are performed by using a proposed online interface where the corpus of a news item is placed.
The contribution of this research is to the field of journalism, social journalism, and information retrieval. From a technical perspective built a benchmark corpus, web crawler, news ontology in protégé, and tool for querying. The aids of the present study also provide promoting relations between semantic web research and the digital news industry. Readers can become social journalists such as bloggers and analysts. Readers don’t need to search different newspapers from different agencies to find information of their interest. They will have a publicly available online platform where all the news is linked and shown according to user interest by semantically querying the corpus of meta-data. Readers and journalists can follow a story easily in a time-lined fashion. Above all, the present work will semantically archive the information to avoid wastage like, in syntactic search, which gives you various non-pertinent web pages as a return of query. Semantic search gives you more appropriate ranked results as output instead of web pages of newspapers.
Some of the previous studies are reviewed for information retrieval systems. Existing approaches of linking online data semantically and query to get the accurate result earlier. Specific focus on electronic Urdu news in image form, develop a benchmark corpus, the ontology of Urdu news, semantic annotations, and queries on that corpus. Mainly, concentrate on accuracy in searching for the relevant result. Many issues have been identified while conducting the literature review on existing methods presented in the research, which are mentioned here. The Image Extraction by Semantic Annotation (IESA) is one of the supreme auspicious and active study areas [23]. Researchers demonstrate much work in this domain. IESA can be obliging for users who need to search the image from a large dataset.
Majeed et al. [5] showcase a work in which they work on the qualitative semantic of some image and label it like (sky, snow, water, etc.) from one image. There proposed model works on the renovation of human graspable high-level features of image into Resource Description Framework (RDF) triple that contains information of source image. Their framework is constructed on annotation-grounded image retrieval and contented-centered image extraction through semantics. An experimental result shows 90 percent accuracy. The images they used in the paper are based on natural scenes.
Rajput [6] presents a context-based annotation framework that can mark documents printed in the Urdu language. The structure practices area precise the ontology and context annotations alternative of Natural Language Processing (NLP) approaches. Hollink et al. [2] showcase a work in which they work on online news text and image datasets. Initially, extract the images and text of most visited newspaper websites like The News York Times. Lastly, prolonging Images in Online News (ION) also covers non-English newscast publishers, mainly because TextRazor can extract subject data in numerous languages. In [11], a novel framework of information retrieval by integrating two models, deep learning word2vect and book ontology are proposed.
Mansouri et al. present a short paper on periodic queries, which are the sub-kind of temporal queries classified by exchanging seeking goals over the years. Preferably, the search would have exceptional retrieval regulations for any of the one-of-a-kind classifications, using this surplus data to supply higher solutions for their users. Knowledge of this periodic behavior might also help search engines like google provide higher reputation methods and reply with temporally appropriate effects main in the end into consumer’s achievement. Thaker [8] presents a paper in which Urdu linguistic query management structure is used. It helps the ontological scheme to catch the response user query. This method also helps us to create automatic ontology against the classes as well.
Ayaz et al. [9] present a paper in which they used a resourceful mapping among semantic exploration and novels in the Urdu discipline. The novel in Urdu set up context exploration engine is the primary of its compassionate that can show a full-size position in indorsing Urdu literature. The machine practices purview ontology to collect and save novel interrelated facts and extract the knowledge by standard query language and protocol. Ali et al. [10] established a rule-based whole stemming approach for the Urdu manuscript in this planned work. The ability of this proposed work has to generate the stalk of Urdu phrases and loan phrases that belong to plagiarized languages, consisting of Arabic, Persian, and Turkish, by doing away with suffix, infix, and prefixes from the terms. This study additionally proposed a method for spotting numerous seasonal queries using time series and content material systems. They show that the reader’s conduct in the search direction is distinguished. Random forest classifier is recycled for class and performed 88.7% F-measure. This technique is free for any language no longer a barrier.
Peterson [11] say that the organization of text papers has become a necessity in today’s world due to the upsurge in the accessibility of automated data over the internet. Two novel algorithms, Ontology-Based Classification, and Hybrid Approaches, the amalgamation of Naïve Bayes and Ontology-Based Classification, are anticipated for Punjabi Text Classification. The tentative results accomplish that Ontology-Based Classification (85%) and Hybrid Approach (85%) deliver healthier consequences in contrast to standard classification procedures, Naïve Bayes Classification (64%), and Centroid Based Classification (71%). Peterson [11], this study is a fragment of a more significant project. I pursue to contest the simple scientific and economic determinist clarifications for public and traditional variation not by controverting them but by highlighting the significance of native conventional environments. Abbas [12] this work particularizes the semi-semantic part of speech mark guidelines for the annotated corpus. A ranked annotation pattern was designed to tag the part of speech and then the pragmatic corpus. The guiding principle presented will be helpful for the language public to annotate sentences not only for the nationwide linguistic Urdu native language other than Urdu like Sindhi, Pashto, Punjab, etc.
Muhammad et al. [13] published this research study based on textual content. It reprocesses the pirating textual content from current documents to provide unique texts. The naturally available online resources aren’t the best to recycle text more public in society but also stiffer to be aware. The Corpus of Urdu News (i.e., COUNTER) incorporates 1200 files with real examples of textual content reprocessing from the broadcasting field.
Now, a significant stage is to work on analyzing roman Urdu text. Nargis et al. [14] proposed a work in which they tell roman Urdu text is utmost public used linguistic on societal networking media in Pakistan and India. It is mutual that in roman Urdu text, many expressions and terms are used to signify emotions. In this research, the researcher also offers a tactic to parse classical Urdu by emerging emotion ontology. In this research [24] the author presented two context-aware recommended systems using machine learning, RDF, Web Ontology Language (OWL), Java, and mapping rules. The article [25] predicts the trends of opinion for decision-making. The natural language processing and machine learning are employed to get the required results. The proposed algorithm [26] is to reduce the stop words that have no semantic implication. This article [27] presents a corpus of 15000 web pages and applies a novel algorithm. The corpus is made by using a web crawler. This article [28,29] present the machine learning technique to minimize the number of annotations.
There is no proper dataset of Urdu news images with metadata to pursue the research, especially in the Urdu language. We cannot query the news image in electronic form because of Urdu language text in an image and the unavailability of an OCR in Urdu and the lack of indexing. This study develops ontology for Urdu news initially using Urdu News job in an online newspaper. Construct a corpus, semi annotates the corpus, query the corpus, and as a result, retrieve news image in electronic form that subsidizes our overall system performance semantically.
The proposed work, called Urdu information linking for sematic temporal queries (UN-LISTQ), demonstrates a novel and unified approach for developing and querying the annotated corpus of Urdu news items. For this purpose, several modules were designed to generate corpus from different news agencies and perform the social annotations by using news ontology.
The use of wordnet is essential in natural language processing. A vast database of semantic wordnet is publicly available for use [12]. So, when we add annotations to images, they can be indexed under the ontology. In our case, we have connected the accessible database with protégé, open-source software supported by the OWL and RDF languages. The expected results are achieved after applying test queries to the resulting annotated Urdu corpus. The main objective of the proposed methodology is to provide a searching facility, querying and getting the optimum results as much as possible. Fig. 1 shows the framework of this research.
Figure 1: Proposed Framework of Urdu information linking for sematic temporal queries
The five essential phases of this work are to generate a news Job corpus (JBCorpus). A job corpus is initially a set of news text images, and these news images are crawled from the web by applying numerous filters and rectifiers. Therefore, generate a job corpus refined (JBcorpusRefined) from the job corpus raw news items directory (JBCorpusRaw). Filter and rectifiers help remove images that are not needed in a semi-automated way by using Urdu News Image (UNI) module, which has Urdu news text in image form. Additionally, the thread concept used in crawling the image plays a vital role in making a corpus of 500 images because we programmed it to reduce obstruction.
This can be happened by using a system of getting a similar idea at least from ten threads. Fig. 2 illustrates the working of the overall system. This fast mechanism reduces overtime for making news image corpus used for further filtration and alteration.
Figure 2: Proposed algorithm from corpus eneration to indexing for UN-LISTQ system
In step S2; developing News ontology by using protégé interface given the concepts, properties, and classes in return News-Owl file is generated, in Step S3 Perform Social Annotation (PS0). Annotate the image under OWL file concepts while End-Of-Corpus (EOC) items. The resultant achievement is annotated job corpus (JBCORPUSAnnotated). After annotation, evaluate the tags by executing a method of Corpus Annotated Evaluation (CAE) in which annotated corpus is analyzed. In step, S4 Indexed Corpus Image (ICI) by integrating the OWL files and evaluated JBCorpus. Therefore job corpus is indexed (JBCorpusindexed), and the query method from readers is persisted. In step S5, return corpus indexed is used using the delimiter function to delimit the query keywords and make a set of query terms. Execute the query, match the individual keywords of query Ti (t1, t2, t3, tN) with the indexed JBCorpus annotations, and count the variable “add count” in the algorithm to rank the image against the query and display it on the news interface.
Additionally, use the relevancy matrix and Term Frequency-Inverse Document Frequency (TF-IDF) to check the similarity and occurrences of the keyword. If the occurrence of a keyword is higher than the other results generated through the query, this can be ranked accordingly. Fig. 2 illustrates the algorithm of the proposed UN-LISTQ system in which the input is raw new images corpus and the output is ranked news retrieval against the semantic query.
Corpus is a vital source in developing an information retrieval system, especially for Urdu news items. There is no such benchmark corpus available for the information retrieval system to query and extract the result of Urdu news in electronic form. In the proposed system, create a corpus of 500 new online images available from numerous news agency websites and crawl from their Uniform Resource Locators (URLs) by giving them the time and title of the job as a concept.
A diverse system of crawlers (web robots) is used to make a corpus. These corpora are used for information retrieval and extraction. One example is that ‘Common crawler’ is used for making the search engine of ‘Hamkinar’. Fig. 3 shows the news image crawler work to build a corpus.
Figure 3: Steps involved in making a corpus
3.3 Preprocessing and Multi-Threaded Environment
Initially, the corpus ‘JBCorpus’ is constructed from the modified image crawler to crawl the news image from the web. The links of some agencies that publish news daily are given to the image crawler to download them for corpus generation. The semi-automated way further preprocesses raw images because the JBCorpus is used as an input for the next module. Fig. 3 illustrates the multi-threaded image crawler that extracts news images from various news agencies by applying multiple filter controls.
The annotations were performed voluntarily by the graduate students in their final semester who were native and Urdu speakers. Everyone is qualified for in-text annotation by demonstrating a tutorial before the experiment. The time duration for annotation to an individual item is 15 min. Annotators tag news images by reading the news images without any pressure, and there is no restriction on how many tags they must provide for any specific image. These annotations are then stored in a corpus that now has images and annotations. After annotations on the news corpus, the analysis of annotations session is conducted through an assistant professor of the university, an expert in this research. The annotation procedure is divided into two significant ways: indexing under the Urdu News Ontology and latterly used for semantic, temporal queries, and reporting. The two ways of annotation are Free Annotation and fixed cross-examination annotate.
Annotators annotate news images freely. Annotators tagged the Urdu News Image (UNI) freely by reading the news and tagged by inferred keywords. In this technique, after annotation, conflict resolving sessions of experts are also held to investigate whether this tag is relevant or irrelevant. Almost the average number of tags per image in free annotations is 5.7, which is suitable for double-check of experts. The average number of tags is reduced to a fixed number of question tags.
3.4.2 Fixed Cross-Examination Annotation
While reading the UNI in a scenario, the annotators must answer the fixed numbers of keywords like when, where, how, and why. The annotations performed under the fixed number of concepts are taken from the annotations. These tags are stored with images for reuse. In a fixed number of annotations, the average number of tags per image is reduced to 3.83.
The primary research component is developing Urdu News ontology for indexing semantic annotations. All the annotation previously done on electronic news images is indexed under the ontology’s concepts. Ontology by meaning is the nature of being. A set of entities, the concept in the actual world also shows properties and relation of the concept between them. The ontology is based on electronic news annotation, including the classes, subclasses, object properties, data, and annotation. There are various Integrated Development Environments (IDE) that freely available to develop ontology. The protégé is used in this scenario. It supports different file extensions like OWL, and RDF. The file is quickly loaded into various online tools to visualize the taxonomy.
In protégé, the general ontology is built for Urdu news available on the web. Specifically for Urdu news job, there is a concept of classes and subclasses, properties like an object, functional and their relationships. There is a scenario in which a concept of teacher, professor, faculty positions, educators, tutors, instructors, and the object properties are same as, is a, belongs to are included. So every professor is a teacher. In owl class, “professor” and the subclass is “teacher.” The property between these classes is “is a”. Similarly, teaching staff, faculty positions, educators’ jobs, and assistant professors have the property same. There are classes and subclasses and their properties.
3.5.2 Ontology Architecture (OA) and Visualizer (OV)
A tremendous ontology visualizer tool adds plugins in protégé to visualize the Urdu news ontology and represent the ontology in a better way to understand and add more features in news ontology that belong to that tool. Various tools have developed the desired ontology for knowledge representation pictorials, like Web-Visual Notation for OWL ontologies, and Online Ontology Visualization (i.e., OWLGrEd). The concept of Urdu news ontology of connected classes is represented in Tab. 1.
Fig. 4 conveys a detailed view of class’s properties object and data range and domain in a systematized manner in protégé, OWLViz shows class’s sub-entities and linkage with sub-class and hierarchy of the things. Both the Pakistan Atomic Energy Commission (PEAC) and PEAC idea are replacements as those two are the same jobs of a newspaper.
Figure 4: Ontology viusalizer, parent-child relationship of news agency and categories of Urdu, linkage concept, annotation data properties
3.5.3 Urdu News Searching Technique
The present work addresses the temporal news item that is job advertisement published daily on the web in electronic form. Individually users are permitted to use the engine by a suitable interface. A person who reads job hunters may query related to the job and do searching operations. Daily news of jobs published from various agencies is crawled and stored in the corpus for further processing.
A lexical analyzer algorithm is worked alongside the query that can parse the query and delimit the reader’s query into a series of tokens. A matcher and pattern are sub-modules of algorithms that can match the query tokens to the corresponding base ontology annotations patterns. The search engine algorithm works quite well, and results are correlated and relevant to the search query. Similarly, metrics of numerous tactics are estimated and authenticate the machine’s entire performance. It can be believed that the search engine and the reader’s question contained two segments explicitly semantic temporal query against ontology indicating Urdu news corpus and information retrieval. Each of these two layers is reflected distinctly in the following subsections.
The user query string is divided into query vectors to match the concerning concepts of news item annotations. A term frequently occurs with news items retrieved and ranked on top. The query vectors retrieved the news items vector annotated previously in Sections 3.3.1 and 3.3.2 when executing the user temporal query. This can be done by checking the similarity and properties lies between these concepts of annotations. Temporal queries apply because the news items collected are time-dependent.
This level mainly works for the extraction module of the search engine. Handle the query to tokenize words and semantically extract data from created base ontology. A substantial amount of queries are made to obtain the data by the application program interface. The prototype application was developed in the .net platform and owl management system in this scenario. Various linked queries have been considered in detail in Tab. 2. It also declared that readers could request jobs for educators in 2018. This retrieval request is verbalized into a delimiter to tokenize into keywords. This query catches the class individual’s jobs department relating distinct educators over an entity property belong to. Subsequently, the performance of query the recent educator’s job in 2018 is obtained.
The query1 “recent educator’s job in 2018” results are in Tab. 3. The occurrences of recent find in 3 images match the concept educator with image one, but image two also has a subclass concept faculty position. The property between the educators and faculty positions is “same as” in ontology, which also retrieved image 2. Tab. 3 clearly shows that the educator’s tags are matched and retrieved in the results.
These include the consequences of various educators’ jobs broadcasts that precisely fit the annotations retrieved. Likewise, the company call of activity _news educators is a query and retrieves results. Query catches the concepts mainly Agency Name via concerning them with individual educators via an object belonging written by. This query extracts the broadcast of news specific to who is the publisher of the Urdu news express. Additionally, the person can manage the name of the city where news jobs are to be held. As shown in Tab. 3, a question is created that catches the name of all of the capital from the Availability class to link individuals of the Availability class with the individual specific the use of property available. Similarly, the remaining test query set of queries is, “2: find out PAEC job for teaching faculty”, “3: Show me the 15 august 2018 jobs in atomic”, “4: Find out all recent Lahore-based educator job”. Tab. 4 illustrates the test query sets to evaluate the annotated corpus.
Besides the properly prepared retrieval of facts from the domain ontology, the search engine additionally authorizes the essential additions of the latest information. The additional necessity usually arises simultaneously as brand-new news is to be had or a new corporation is announced.
Correspondingly, the updating is compulsory to update the data of ontology. The responsibility to feature the information, writer, and reader statistics lies with the writer. Separately from inserting the new data inside the ontology, there may be a necessity to make deleting movements in the area of ontology. These moves are performed with the aid of the person admin simply.
Furthermore, the admin is capable of performing additional and powerful features. The expansion, updating, and elimination structures inside the area ontology are added by using the third and remaining section of the engine. This stage lets in changing the present site ontology by inserting, eliminating, and updating various constraints. In this stage, the most straightforward part of the editor and admin is well-described. Further, in Urdu information linking for sematic temporal queries (i.e., UN-LISTQ), this module is applied for the usage of OWL Application Programming Interface (API).
3.5.5 Evaluations Parameters for UN-LISTQ System
The valuation of this system is estimated by the following parameters like Precision, recall, and F measure. The reader’s query annotated corpus. The query vector is then matched with the concepts of the annotated ontology. The rank results are generated in information retrieval systems by applying the query discussed previously. These results are evaluated by the performance check parameters including precision, recall and F measure, which can be defined as follows,
Precision is the number of relevant images distributed by a total number of images,
The recall is slightly altered from precision. It is the summation of related images alienated by a whole number of images existing in the corpus,
We need to mention that we calculate the harmonic mean of two different parameter including the precision and recall in the F measure. The best score is observed as 1 of the F measure and found worst when the F measure is 0.
In the proposed research, the user interface is developed for natural language queries. The platform used to create the interface for querying the annotated corpus is .net framework 4.0. C sharp language is used for development. There is a 3-tier application architecture including user interface, business logic, and data storage layers.
There is a generation of a corpus, development of ontology, perform social annotations from the annotators, and build an interface for querying in natural language. Apply test queries and find out the occurrences of matching concepts. Furthermore, evaluation parameters are over-performance check estimators that are used in the results and discussion chapter.
Applications of UN-LISTQ are developed by using the integrated development environment visual studio of version 13.0, which is set in with the image control strategies and the quantifiable gadgets. A platform of Intel core i5 with 8 GB memory is used throughout the implementation. Initially, 500 images are practiced in our system.
This system is evaluated from two perspectives that were discussed in Section 3.4. One annotation strategy is freely annotated, and the remaining is fixed cross-examination annotation or ontological annotations. The corpus is queried through the online interface. Readers put the temporal query on temporal news job items. The question is then divided into query vectors and matches the annotated job news item. If the query vector matches the relevant annotations concept, the retrieved results are ranked accordingly. Furthermore, these outcomes are evaluated by various performance measurements like calculating the Precision, recall, and f measure to assess the relevancy.
4.1 Evaluation of Free Annotation
In free annotation, the readers annotate the Urdu news job items. The average number of annotations in this scenario is 5.7. The average number of annotations increased because the annotators freely annotate what they feel is mandatory to tag the new items. In Section 3.5, the test query set used in prototyping was four to estimate the Precision, recall, and F measure. These performance parameters help us figure out the UN-LISTQ system’s accuracy. The table illustrates the query vectors and the resultant annotated results and shows the Precision and recall of the query outcomes.
It is clearly shown in Tab. 4 that queries vector occurrences and possible outcomes of annotation as a result of executing the query. These four test queries are evaluated by the performance measurement discussed in Section 3.5. When query1 is performed, following annotations like recent, job, Punjab, educator, etc., are retrieved with news images. So Precision can be found by checking the relevancy and the retrieved annotations. Precision is a fraction of the total number of query vectors and the relevant retrieved annotations. The Precision of query1 is 0.6 when converted into a percentage of 60% of Precision achieved. Similarly, recall is 0.214, and f measure is 0.316. The query2, query3, and query4 performances are clearly shown in Tab. 5. This table is converted into a graph to visualize the query set evaluation and implementation.
4.2 Evaluation of Fixed Ontological Annotation of Test Queries
The annotator tags news images against a fixed number of concepts like when, where, how, etc. So the average number of annotations in scenario 3 is reduced by half of the free annotations. From earlier, the test query set used in prototyping was four to estimate the Precision, recall, and F measure. These performance parameters help us figure out the UN-LISTQ system’s accuracy. The same queries are executed on the ontological concept annotations and retrieved results far better than the free annotation tag-based systems. This system reduced recall and balanced the Precision, memory, and f measure. Tab. 5 clearly shows the query performance in ontological concept annotations.
It is visible in Tab. 5 that the query set is executed on the ontological annotations. The acquired results are far better than the free annotations. Query1 Precision is 100% in ontological annotations while applying the same query vector on free annotations gives 60% precision. Similarly, recall and f measure are also balanced with the Precision in this concept annotation technique. The ontological annotations provide more accurate results because the property lies between the ontology and can classify the annotation under the concepts. So redundancy can easily remove, and recall is balanced because the average amount of annotations is reduced in ontological annotations.
4.3 Comparison of FA and OA by F-Measure
Both tactics discussed previously can be evaluated by finding the Precision, recall, and f measure. Now, by comparing free annotation and ontological annotations, the following findings are clearly shown in Fig. 5. When query1 is executed, it can be divided into five query vectors. The outcome of this query concerning fixed annotations is fourteen, and in ontological annotations, these result is significantly decreased by seven. So when calculating the Precision, recall, and f measure, results are 0.6 P, 0.2 R, and 0.3 F, respectively. F measure is decreased here because the memory is reduced, and precision increases. We need balance results. Suppose the relevant results are achieved against the query and precision increases.
Figure 5: Evaluation of free annotation of four queries
Similarly, recall decreases in query1 because the free annotations are 14 in the corpus retrieved by executing query1. When completed, the result retrieved concerning the query is reduced to seven in the ontological annotation. When calculating the precision, recall and f measure achieved 1 P, 0.7 R, and 0.8 F. Because the average annotations are reduced, and relevancy is increased in ontological annotations. Fig. 6 visualizes the comparison of free annotations and ontological annotations. When query2 is executed on the free annotations interface, the precision-recall and f-measure are 0.7, 0.5, and 0.6. Respectively, when the same query is performed on the ontological annotations interface. The same result is retrieved because the annotations matched with query2 are nine. Similarly, query3 and query4 conducted on interface-free and fixed annotations discussed in Tabs. 4 and 5 have scored 0.8 f measure and 0.3 F measure.
Figure 6: Query set evaluation for ontological annotations
The present work aimed to study and link daily news items semantically so that they can be queried by readers, job hunters, and journalists. Develop concept, annotations, and ontology which need to update as the new department enrolls; edition can quickly be done from the user interface. Similarly, news items linked together provide a complete story coverage and bring together different opinions at a single location for readers to do the analysis themselves. The performance can be evaluated by calculating precision, recall, and f measure of both tactics, the free annotations, and ontological annotations. The overall performance of the ontological annotation system is significantly increased compared to the modern annotations system. In this study, only using a corpus of size 500 images and 13,000 annotations, this structure can progress by considering an enormous corpus of size like 10,000 images. It increases to check the correctness of UN-LISTQ using annotations of fixed ontological concepts by balancing the recall issue and generating more accurate and precise results. The breakdown of the query into query vectors, searching the annotations semantically, and matching with relevant annotations is a challenging task that can be overcome by using advanced machine learning algorithms. Optimizing in annotating the images technique, a thesaurus of similar words which remove ambiguity from the current scenario in ontology to match the most accurate synonym and infer it would be the work that needs to be done.
Acknowledgement: The authors extend their appreciation to King Saud University for funding this work through Researchers Supporting Project number (RSP-2021/387), King Saud University, Riyadh, Saudi Arabia.
Funding Statement: This work was supported by King Saud University through Researchers Supporting Project number (RSP-2021/387), King Saud University, Riyadh, Saudi Arabia.
Conflicts of Interest: The authors declare that they have no conflicts of interest to report regarding the present study.
References
1. H. Mohammadzadeh, D. -Ulm, T. Gottron, D. -Koblenz, F. Schweiggert et al., “TitleFinder: Extracting the headline of news web pages,” in Proc. Int. Workshop on Web Information & Data Management, Melbourne, Australia, pp. 65–72, 2015. [Google Scholar]
2. L. Hollink, A. Bedjeti, M. V. Harmelen and D. Elliott, “A corpus of images and text in online news,” in Proc. Int. Conf. on Language Resources and Evaluation, Portorož, Slovenia, pp. 1377–1382, 2016. [Google Scholar]
3. N. Fernández, D. Fuentes, L. Sánchez and J. A. Fisteus, “The news ontology: Design and applications,” Expert Systems with Applications, vol. 37, no. 12, pp. 8694–8704, 2010. [Google Scholar]
4. M. Mehfooza and V. Pattabiraman, “An assessment on domain ontology-based information extraction techniques,” International Journal of Services Technology & Management, vol. 23, no. 4, pp. 299–312, 2013. [Google Scholar]
5. S. Majeed, Z. U. Qayyum and S. Sarwar, “SIREA: Image retrieval using ontology of qualitative semantic image descriptions,” in Proc. Int. Conf. on Information Science & Applications, Poland, pp. 1–6, 2013. [Google Scholar]
6. Q. Rajput, “Ontology based semantic annotation of Urdu language web documents,” Procedia Computer Science, vol. 35, no. 1, pp. 662–670, 2014. [Google Scholar]
7. T. T. S. Nguyen, “A deep learning framework for book search,” in Proc. Int. Conf. on Information Integration & Web-based Applications & Services, Singapore, pp. 81–85, 2016. [Google Scholar]
8. R. Thaker, “Domain specific ontology based query processing system for Urdu language,” International Journal of Computer Applications, vol. 121, no. 13, pp. 20–23, 2015. [Google Scholar]
9. B. Ayaz, W. Altaf, F. Sadiq, H. Ahmed and M. A. Ismail, “Novel Mania: A semantic search engine for Urdu,” in Proc. Int. Conf. on Open Source Systems & Technologies, Gothenburg, Sweden, pp. 42–47, 2016. [Google Scholar]
10. M. Ali, S. Khalid and M. H. Aslam, “Pattern based comprehensive Urdu stemmer and short text classification,” IEEE Access, vol. 6, pp. 7374–7389, 2018. [Google Scholar]
11. M. A. Peterson, “Katibs and computers: Innovation and ideology in the Urdu newspaper revival,” Contemporary South Asia, vol. 22, no. 2, pp. 130–142, 2014. [Google Scholar]
12. Q. Abbas, “Semi-semantic annotation: A guideline for the Urdu. KON-TB treebank POS annotation,” Acta Linguistica Asiatica, vol. 6, no. 2, pp. 97–134, 2016. [Google Scholar]
13. S. Muhammad, R. M. A. Nawab and R. Paul, “COUNTER: Corpus of Urdu news text reuse,” Language Resources & Evaluation, vol. 51, pp. 777–803, 2016. [Google Scholar]
14. G. Z. Nargis and N. Jamil, “Generating an emotion ontology for Roman Urdu text,” International Journal of Computational Linguistics Research, vol. 7, no. 3, pp. 83–91, 2016. [Google Scholar]
15. I. M. El-Henawy and K. Ahmed, “Content-based image retrieval using multiresolution analysis of shape-based classified images,” Global Journal of Computers & Technology, vol. 1, no. 1, pp. 1–8, 2014. [Google Scholar]
16. V. Gupta, “Domain based classification of Punjabi text documents using ontology and hybrid based approach,” in Proc. Workshop on South & Southeast Asian Natural Language Processing, Mumbai, India, pp. 109–122, 2010. [Google Scholar]
17. J. Nandigam, V. N. Gudivada and M. Kalavala, “Semantic web services,” Journal of Computing Sciences in Colleges, vol. 21, no. 1, pp. 50–63, 2005. [Google Scholar]
18. V. Lopez, M. Fernández, E. Motta and N. Stieler, “PowerAqua: Supporting users in querying and exploring the semantic web,” Semantic Web, vol. 3, no. 3, pp. 249–265, 2012. [Google Scholar]
19. J. Fang, P. Nevin, V. Kairys, Č. Venclovas, J. R. Engen et al., “Conformational analysis of processivity clamps in solution demonstrates that tertiary structure does not correlate with protein dynamics Structure,” NIH Public Access, vol. 22, no. 4, pp. 572–584, 2014. [Google Scholar]
20. P. Baker, C. Gabrielatos and T. McEnery, “Sketching Muslims: A corpus-driven analysis of representations around the word “Muslim” in the British press 1998–2009,” Applied Linguistics, vol. 34, no. 3, pp. 255–278, 2013. [Google Scholar]
21. V. Gupta, N. Joshi and I. Mathur, “Approach for multiword expression recognition and annotation in Urdu corpora,” in Proc. Int. Conf. on Image Information Processing, Beijing, China, pp. 1–6, 2018. [Google Scholar]
22. P. Oliveira and J. Rocha, “Semantic annotation tools survey,” in Proc. IEEE Symp. on Computational Intelligence and Data Mining, Orlando, FL, USA, pp. 301–307, 2013. [Google Scholar]
23. K. Riaz, “Rule-based named entity recognition in Urdu,” in Proc. Named Entities Workshop, Uppsala, Sweden, pp. 126–135, 2010. [Google Scholar]
24. U. Javed, K. Shaukat, I. A. Hameed, F. Iqbal, T. M. Alam et al., “A review of content-based and context-based recommendation systems,” International Journal of Emerging Technologies and Learning, vol. 16, no. 3, pp. 274, 2021. [Google Scholar]
25. K. Shaukat, A. T. Mehboob, M. Ahmed, S. Lou, I. A. Hameed et al., “A model to enhance governance issues through opinion extraction,” in Proc. Int. Conf. and Workshop on Computing & Communication, Guangzhou, China, pp. 511–516, 2020. [Google Scholar]
26. D. K. Shaukat, A. B. Shafat and H. M. Umair, “An efficient stop word elimination algorithm for Urdu language,” in Proc. Int. Conf. on Electrical Engineering/Electronics, Computer, Telecommunications and Information Technology, Thailand, pp. 911–914, 2017. [Google Scholar]
27. K. Shaukat, N. Masood and M. Khishi, “A novel approach to data extraction on hyperlinked webpages,” Applied Sciences, vol. 16, no. 5, pp. 317–329, 2019. [Google Scholar]
28. H. Sun and K. Grishman, “Employing lexicalized dependency paths for active learning of relation extraction,” Intelligent Automation & Soft Computing, vol. 34, no. 3, pp. 1415–1423, 2022. [Google Scholar]
29. M. Islam, M. Usman and M. Azhar, “Predictive analytics framework for accurate estimation of child mortality rates for Internet of Things enabled smart healthcare systems,” International Journal of Distributws Sensor Network, vol. 16, no. 5, pp. 415–426, 2020. [Google Scholar]
Cite This Article
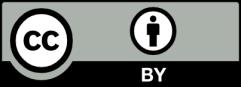
This work is licensed under a Creative Commons Attribution 4.0 International License , which permits unrestricted use, distribution, and reproduction in any medium, provided the original work is properly cited.