Open Access
ARTICLE
Time Series Forecasting Fusion Network Model Based on Prophet and Improved LSTM
1 College of Urban Rall Translt and Logistics, Beijing Union University, Beijing, 100101, China
2 Beijing Key Laboratory of Information Service Engineering, Beijing Union University, Beijing, 100101, China
3 Faculty of Business, Economics, and Law, The University of Queensland, Brisbane, 4072, Australia
4 Department of Oncology, Wangjing Hospital, China Academy of Chinese Medical Sciences, Beijing, 100102, China
* Corresponding Author: Xin Yu. Email:
Computers, Materials & Continua 2023, 74(2), 3199-3219. https://doi.org/10.32604/cmc.2023.032595
Received 23 May 2022; Accepted 09 August 2022; Issue published 31 October 2022
Abstract
Time series forecasting and analysis are widely used in many fields and application scenarios. Time series historical data reflects the change pattern and trend, which can serve the application and decision in each application scenario to a certain extent. In this paper, we select the time series prediction problem in the atmospheric environment scenario to start the application research. In terms of data support, we obtain the data of nearly 3500 vehicles in some cities in China from Runwoda Research Institute, focusing on the major pollutant emission data of non-road mobile machinery and high emission vehicles in Beijing and Bozhou, Anhui Province to build the dataset and conduct the time series prediction analysis experiments on them. This paper proposes a P-gLSTNet model, and uses Autoregressive Integrated Moving Average model (ARIMA), long and short-term memory (LSTM), and Prophet to predict and compare the emissions in the future period. The experiments are validated on four public data sets and one self-collected data set, and the mean absolute error (MAE), root mean square error (RMSE), and mean absolute percentage error (MAPE) are selected as the evaluation metrics. The experimental results show that the proposed P-gLSTNet fusion model predicts less error, outperforms the backbone method, and is more suitable for the prediction of time-series data in this scenario.Keywords
Cite This Article
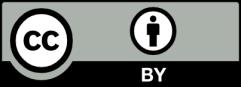