Open Access
ARTICLE
Developing a Secure Framework Using Feature Selection and Attack Detection Technique
Department of Electrical Engineering and Computer Science, College of Engineering and Applied Science, University of Cincinnati, 2600 Clifton Ave, Cincinnati, OH 45221, USA
* Corresponding Author: Mahima Dahiya. Email:
Computers, Materials & Continua 2023, 74(2), 4183-4201. https://doi.org/10.32604/cmc.2023.032430
Received 18 May 2022; Accepted 12 July 2022; Issue published 31 October 2022
Abstract
Intrusion detection is critical to guaranteeing the safety of the data in the network. Even though, since Internet commerce has grown at a breakneck pace, network traffic kinds are rising daily, and network behavior characteristics are becoming increasingly complicated, posing significant hurdles to intrusion detection. The challenges in terms of false positives, false negatives, low detection accuracy, high running time, adversarial attacks, uncertain attacks, etc. lead to insecure Intrusion Detection System (IDS). To offset the existing challenge, the work has developed a secure Data Mining Intrusion detection system (DataMIDS) framework using Functional Perturbation (FP) feature selection and Bengio Nesterov Momentum-based Tuned Generative Adversarial Network (BNM-tGAN) attack detection technique. The data mining-based framework provides shallow learning of features and emphasizes feature engineering as well as selection. Initially, the IDS data are analyzed for missing values based on the Marginal Likelihood Fisher Information Matrix technique (MLFIMT) that identifies the relationship among the missing values and attack classes. Based on the analysis, the missing values are classified as Missing Completely at Random (MCAR), Missing at random (MAR), Missing Not at Random (MNAR), and handled according to the types. Thereafter, categorical features are handled followed by feature scaling using Absolute Median Division based Robust Scalar (AMD-RS) and the Handling of the imbalanced dataset. The selection of relevant features is initiated using FP that uses ‘3’ Feature Selection (FS) techniques i.e., Inverse Chi Square based Flamingo Search (ICS-FSO) wrapper method, Hyperparameter Tuned Threshold based Decision Tree (HpTT-DT) embedded method, and Xavier Normal Distribution based Relief (XavND-Relief) filter method. Finally, the selected features are trained and tested for detecting attacks using BNM-tGAN. The Experimental analysis demonstrates that the introduced DataMIDS framework produces an accurate diagnosis about the attack with low computation time. The work avoids false alarm rate of attacks and remains to be relatively robust against malicious attacks as compared to existing methods.Keywords
Cite This Article
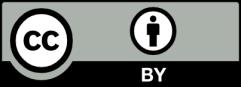
This work is licensed under a Creative Commons Attribution 4.0 International License , which permits unrestricted use, distribution, and reproduction in any medium, provided the original work is properly cited.