Open Access
ARTICLE
Hybrid Models for Breast Cancer Detection via Transfer Learning Technique
1 Department of Information Technology, JSS Academy of Technical Education, Noida, India
2 JSS Academy of Technical Education, Noida, India
3 Department of Electronics and Communication Engineering, JECRC University Jaipur, Rajasthan, India
4 Harcourt Butler Technological University Kanpur, India
5 Department of Electrical Engineering, Prince Sattam Bin Abdulaziz University, College of Engineering, Al Kharj, 16278, Saudi Arabia
6 Faculty of Engineering, Imperial College London, London, SW7 2AZ, UK
7 College of Arts, Media and Technology, Chiang Mai University, Chiang Mai, 50200, Thailand
* Corresponding Author: Orawit Thinnukool. Email:
Computers, Materials & Continua 2023, 74(2), 3063-3083. https://doi.org/10.32604/cmc.2023.032363
Received 15 May 2022; Accepted 27 June 2022; Issue published 31 October 2022
Abstract
Currently, breast cancer has been a major cause of deaths in women worldwide and the World Health Organization (WHO) has confirmed this. The severity of this disease can be minimized to the large extend, if it is diagnosed properly at an early stage of the disease. Therefore, the proper treatment of a patient having cancer can be processed in better way, if it can be diagnosed properly as early as possible using the better algorithms. Moreover, it has been currently observed that the deep neural networks have delivered remarkable performance for detecting cancer in histopathological images of breast tissues. To address the above said issues, this paper presents a hybrid model using the transfer learning to study the histopathological images, which help in detection and rectification of the disease at a low cost. Extensive dataset experiments were carried out to validate the suggested hybrid model in this paper. The experimental results show that the proposed model outperformed the baseline methods, with F-scores of 0.81 for DenseNet + Logistic Regression hybrid model, (F-score: 0.73) for Visual Geometry Group (VGG) + Logistic Regression hybrid model, (F-score: 0.74) for VGG + Random Forest, (F-score: 0.79) for DenseNet + Random Forest, and (F-score: 0.79) for VGG + Densenet + Logistic Regression hybrid model on the dataset of histopathological images.Keywords
Cite This Article
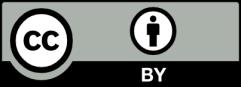
This work is licensed under a Creative Commons Attribution 4.0 International License , which permits unrestricted use, distribution, and reproduction in any medium, provided the original work is properly cited.