Open Access
ARTICLE
SRResNet Performance Enhancement Using Patch Inputs and Partial Convolution-Based Padding
1 Division of Software, Hallym University, 1 Hallymdaehak-gil, Chuncheon, Gangwon-do, Korea
2 Department of Information Technology, University of Gujrat, Jalalpur Jattan Road, Gujrat, Pakistan
* Corresponding Author: Seong-Ho Song. Email:
Computers, Materials & Continua 2023, 74(2), 2999-3014. https://doi.org/10.32604/cmc.2023.032326
Received 14 May 2022; Accepted 22 June 2022; Issue published 31 October 2022
Abstract
Due to highly underdetermined nature of Single Image Super-Resolution (SISR) problem, deep learning neural networks are required to be more deeper to solve the problem effectively. One of deep neural networks successful in the Super-Resolution (SR) problem is ResNet which can render the capability of deeper networks with the help of skip connections. However, zero padding (ZP) scheme in the network restricts benefits of skip connections in SRResNet and its performance as the ratio of the number of pure input data to that of zero padded data increases. In this paper. we consider the ResNet with Partial Convolution based Padding (PCP) instead of ZP to solve SR problem. Since training of deep neural networks using patch images is advantageous in many aspects such as the number of training image data and network complexities, patch image based SR performance is compared with single full image based one. The experimental results show that patch based SRResNet SR results are better than single full image based ones and the performance of deep SRResNet with PCP is better than the one with ZP.Keywords
Cite This Article
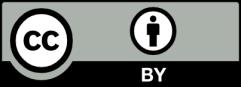