Open Access
ARTICLE
Federation Boosting Tree for Originator Rights Protection
1 Harbin University of Science and Technology, Harbin, 150040, China
2 School of Cyberspace Science, Harbin Institute of Technology, Harbin, 150001, China
3 The Ohio State University, Columbus, 43202, USA
4 Heilongjiang Province Cyberspace Research Center, Harbin, 150001, China
* Corresponding Author: Chao Ma. Email:
Computers, Materials & Continua 2023, 74(2), 4043-4058. https://doi.org/10.32604/cmc.2023.031684
Received 24 April 2022; Accepted 29 June 2022; Issue published 31 October 2022
Abstract
The problem of data island hinders the application of big data in artificial intelligence model training, so researchers propose a federated learning framework. It enables model training without having to centralize all data in a central storage point. In the current horizontal federated learning scheme, each participant gets the final jointly trained model. No solution is proposed for scenarios where participants only provide training data in exchange for benefits, but do not care about the final jointly trained model. Therefore, this paper proposes a new boosted tree algorithm, called RPBT (the originator Rights Protected federated Boosted Tree algorithm). Compared with the current horizontal federal learning algorithm, each participant will obtain the final jointly trained model. RPBT can guarantee that the local data of the participants will not be leaked, while the final jointly trained model cannot be obtained. It is worth mentioning that, from the perspective of the participants, the scheme uses the batch idea to make the participants participate in the training in random batches. Therefore, this scheme is more suitable for scenarios where a large number of participants are jointly modeling. Furthermore, a small number of participants will not actually participate in the joint training process. Therefore, the proposed scheme is more secure. Theoretical analysis and experimental evaluations show that RPBT is secure, accurate and efficient.Keywords
Cite This Article
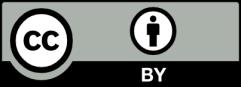