Open Access
ARTICLE
A Dual Attention Encoder-Decoder Text Summarization Model
1 Jazan University, Computer Science Department, College of Computer Science and Information Technology, Jazan, Saudi Arabia
2 Department of Computer Sciences, College of Computer and Information Sciences, Princess Nourah bint Abdulrahman University, P.O. Box 84428, Riyadh, 11671, Saudi Arabia
* Corresponding Author: Hanan Ahmed Hosni Mahmoud. Email:
Computers, Materials & Continua 2023, 74(2), 3697-3710. https://doi.org/10.32604/cmc.2023.031525
Received 20 April 2022; Accepted 12 June 2022; Issue published 31 October 2022
Abstract
A worthy text summarization should represent the fundamental content of the document. Recent studies on computerized text summarization tried to present solutions to this challenging problem. Attention models are employed extensively in text summarization process. Classical attention techniques are utilized to acquire the context data in the decoding phase. Nevertheless, without real and efficient feature extraction, the produced summary may diverge from the core topic. In this article, we present an encoder-decoder attention system employing dual attention mechanism. In the dual attention mechanism, the attention algorithm gathers main data from the encoder side. In the dual attention model, the system can capture and produce more rational main content. The merging of the two attention phases produces precise and rational text summaries. The enhanced attention mechanism gives high score to text repetition to increase phrase score. It also captures the relationship between phrases and the title giving them higher score. We assessed our proposed model with or without significance optimization using ablation procedure. Our model with significance optimization achieved the highest performance of 96.7% precision and the least CPU time among other models in both training and sentence extraction.Keywords
Cite This Article
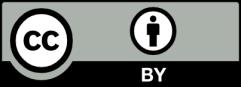
This work is licensed under a Creative Commons Attribution 4.0 International License , which permits unrestricted use, distribution, and reproduction in any medium, provided the original work is properly cited.