Open Access
ARTICLE
A Grey Simulation-Based Fuzzy Hierarchical Approach for Diagnosing Healthcare Service Quality
Faculty of Business, FPT University, Hanoi, 100000, Vietnam
* Corresponding Author: Phi-Hung Nguyen. Email:
Computers, Materials & Continua 2023, 74(2), 3231-3248. https://doi.org/10.32604/cmc.2023.031428
Received 18 April 2022; Accepted 27 June 2022; Issue published 31 October 2022
Abstract
This study aims to assess and rank the service quality of the healthcare system utilizing a Fuzzy Analytical Hierarchical Process (Fuzzy AHP) and Grey Relational Analysis (Fuzzy GRA) technique. In this study, the six primary characteristics of healthcare service quality, comprising Tangibles (A), Healthcare Staff (B), Responsiveness (C), Relationships (D), Support Service (E), and Accessibility (F), are examined through a case study of 20 private and public hospitals in Hanoi, Vietnam. The weighting results of Fuzzy AHP technique indicated that Responsiveness (C) has the highest ranking, followed by Relationships (D) and Healthcare Staff (B). Meanwhile, Tangibility has finally comprised the next priority for increasing satisfaction with the service quality in the healthcare industry. Additionally, the highest service quality rankings are top 5 private hospitals via the Fuzzy GRA approach. Notably, this proposed approach may be applied to a complex decision-making process, which often makes sense with subjective data or imprecise information.Keywords
Healthcare is a fundamental and essetial issue whose importance transcends all aspects of society. Consequently, it has medical, social, political, moral, and economic consequences [1]. Numerous nations emphasize spending on their healthcare systems. Vietnam’s healthcare industry has promise due to recent demographic and socioeconomic shifts. Rapid economic growth in Vietnam has increased the demand for higher quality and specialized healthcare services, particularly among the expanding middle class. The epidemic of coronavirus illness (COVID-19) has demonstrated that health is and will continue to be a top priority for most Vietnamese.
In addition, rising worries about food safety, pollution, and unsafe living and working environments have increased people’s willingness to invest in medicine and healthcare. As illustrated in Fig. 1, the number and distribution of public and private health facilities are available at all levels. Most private hospitals are small, urban institutions that account for 4.2% of all hospital beds in Vietnam. The private sector provides services to people in both urban and rural areas who are willing and able to pay. Even though they live near public health services, people frequently seek care at private health facilities. The private sector provides various services, such as pediatrics, care for the aged, sexual and reproductive health, prenatal care, family planning, and traditional medicine. In industries such as healthcare, where a patient’s knowledge of the technical facets of the service provided is frequently inadequate or nonexistent, service quality becomes even more crucial. Under these conditions, the functional aspect becomes more significant because patients evaluate the entire service based on its delivery [2].
Figure 1: Distribution of public and private health facilities in Vietnam
In the context of emerging countries such as Vietnam, recent publications have examined the quality of medical care; however, these investigations were limited to a single facility and a small sample size. Accordingly, various studies focused exclusively on objective patient-perspective influencing factors, with no systematic or complete examination of expert perspectives. Numerous indicators can be employed to evaluate the efficacy of the healthcare system, but it is difficult to improve them due to limited resources. If the most significant and impactful indicators are recognized, healthcare performance can be enhanced gradually. Evaluating the service quality of healthcare management from the patient’s perspective is beneficial for hospitals’ management in identifying fundamental variables influencing service quality and creating efficient strategies to remedy difficulties with hospital service quality. As far as we know, this is the first study to present a combined multi-criteria decision-making (MCDM) approach of AHP and GRA utilizing Fuzzy Sets to calculate the rankings of twenty private and public hospitals in Hanoi, Vietnam.
This study mainly focuses on an essential theoretical and key objectives as follows: (i) To identify determinants of service quality assessment in the case of Public and Private hospitals in Hanoi, Vietnam; (ii) To determine the importance (weight) of proposed service quality criteria in the case of Public and Private hospitals in Hanoi, Vietnam. (iii) To rank service quality in the case of Public and Private hospitals in Hanoi, Vietnam. To fulfill the mentioned purposes is to answer the following research questions: (i) Which are determinants of service quality assessment in the case of Public and Private hospitals in Hanoi, Vietnam? (ii) How do the proposed criteria influence service quality in the case of Public and Private hospitals in Hanoi, Vietnam? (iii) How do the proposed Fuzzy AHP and GRA methods evaluate service quality in the case of Public and Private hospitals in Hanoi, Vietnam?.
2.1 Service Quality in Healthcare Services
Consumer behavior in healthcare is more dynamic than in less professional services. Patients were frequently unable to make decisions, and treatment decisions were left to the discretion of the nurse or physician. The physical condition of the hospital (Tangibles) also considerably affects customer satisfaction [3]. Responsiveness, Assurance, Communication, Discipline, and Satisfaction are the characteristics used to assess service quality [4]. Clemes et al. [5] concluded that there is a difference in service quality perceptions with different Geographic, Demographic and Behavioral characteristics, and they are important. The personnel, front-line employees and the support staff are most important in providing better facilities and enhancing customer satisfaction. Barksdale et al. [6] developed a paradigm that emphasizes the process of maintaining the patient-physician connection.
How doctors treat, patients can affect their emotional disposition and level of contentment. When numerous doctors are assigned to a patient, the patient may not feel invested in the connection. Patients with a low level of education rated their performance as superior to those with a higher level of education [7]. Lee et al. [8] examined the relationship between the quality of medical treatment and consumer satisfaction. It is widely believed that the quality of medical care directly impacts a patient’s health. However, this belief is significantly lower in many low-income nations. Providers of healthcare in the private sector are highly responsive to customer expectations. The quality of care varies between wealthy and poor patients. The care provided to impoverished patients is of lower quality; most of them visit generic government hospitals and receive standard care with little physician engagement. Wealthier patients connect with more competent service providers and receive better care as a result [9]. There is a substantial relationship between hospital service quality, patient satisfaction, reputation, and performance. The hospital’s reputation can be enhanced by emphasizing patient care and being personable [10]. In recent years, the importance of effective customer service has tripled in the services industry due to intensifying competition from private enterprises, improved technology, and greater consumer satisfaction. Utilizing client happiness and loyalty effectively presents a tremendous opportunity for maximizing profits. Until now, the majority of measurement programs have focused on how external clients evaluate the quality of services provided by businesses. In the healthcare industry, quality of care is not simply a concept. It is now essential for patient health and economic survival. Çaha [11] examined the private healthcare market in Turkey and concluded that individuals chose private hospitals because they believed only private hospitals gave superior care. Despite the hazards posed by private healthcare providers, the demand for personal healthcare services is projected to increase soon. Patients’ contentment appears to be the most influential factor in their decision to utilize private healthcare services.
Consumers can measure the service experience based on their a priori preferences and use it to assess consistency, evaluate satisfaction, and shape expectations of potential consumption experiences [12]. Tseng [13] described service quality standards as service forecasts, an optimal level, an attribute of value, and customer appraisal of service quality. There have been numerous researchers studying this topic in various fields: manufacturing [14]; banking and finance [15]; healthcare service [16]; airline [17]; tourism and hospitality [18]; supply chain management [19]. Because of the intangibility, heterogeneity, and simultaneity of healthcare, determining the quality of service can be challenging. In addition, the diverse interests of healthcare professionals in providing healthcare services and the need for ethical considerations when faced with a problem contributing to the challenge of defining and evaluating quality. In addition, the service provider for a patient differs due to differences in background, experience, skills, and personal qualities of healthcare professionals. Healthcare services are simultaneously produced and consumed; they cannot be stored for later use. Therefore, a multidimensional definition of healthcare quality is required that encompasses the diverse perspectives of healthcare stakeholders. A systematic review of MCDM techniques used in healthcare service quality evaluation to understand the link between service quality and healthcare was investigated [20]. Stević et al. [21] proposed a new Measurement of Alternatives and Ranking according to COmpromise Solution (MARCOS) method for sustainable supplier selection in the healthcare industry in Bosnia and Herzegovina. Si et al. [22] proposed a hybrid MCDM approach of decision-making trial and evaluation laboratory (DEMATEL) to identify key performance indicators (KPIs) for holistic hospital management. It is essential to measure the occupational health and safety (OHS) performance quickly, objectively and realistically; to determine the effects of legislative changes and new OHS applications; to compare the performance of a company with others in the same sector to determine the OHS level of subcontractors. To obtain performance indices, PROMETHEE (Preference Ranking Organization Method for Enrichment Evaluations) and GRA (Grey Relational Analysis) methods were carried out in 27 hospitals in Korkusuz et al. [23]. Hospital site selection affects the hospital’s personnel, resources, environment, and even long-term benefit and cost. Technique for Order Preference by Similarity to Ideal Solution (TOPSIS), Evaluation based on Distance from Average Solution (EDAS), and COmbinative Distance-based Assessment (CODAS) methods which are distance-based MCDM methods were applied to the hospital site selection problem [24]. After reviewing the pertinent literature, this study contributes to the understanding of hospital service quality evaluation by introducing the following innovations:
Utilizing a fuzzy linguistic scale and pairwise comparison, a Fuzzy AHP technique was utilized for the first time in hospital environments to get importance weights for service quality criteria.
In the existing literature, no attempt has been made to evaluate the service quality performance of public and private hospitals from the perspective of different expert groups. Consequently, this study aims to fill the gap in this area utilizing the provided method (Fuzzy AHP and Fuzzy GRA). This method enables hospital performance evaluations that are more cognizant of experts’ subjective judgments.
It is expected to represent a basis for decisions and policies that hospital authorities must take as part of their health delivery processes.
2.3 Criteria of Healthcare Service Quality
Referring to previously related works from the existing literature, healthcare service quality management can be categorized into six main aspects, as shown in Fig. 2.
Figure 2: Proposed criteria
Tangibles (A): Tangibility represents the service physically. It is defined as the physical appearance of facilities, workers’ appearance, and the communication materials used to give services to them. Businesses frequently use tangibility to emphasize their image and quality [2].
Healthcare Staff (B): The organized body of licensed physicians and other healthcare providers permitted by law and a hospital to provide medical care within that hospital or facility. They perform promised services about healthcare accurately on time [11].
Responsiveness (C): It refers to a person’s willingness or availability to help customers and provide timely service. This dimension emphasizes attentiveness and promptness in dealing with customer requests, questions, complaints and problems [13].
Support Services (D): All services performed, provided, or arranged by the Services to promote, improve, conserve, or restore customers’ mental or physical well-being [23].
Relationships (E): It is defined as the relationship between patients and healthcare staff. This relationship represents the caring, individual attention a firm provides to its customers [23].
Accessibility (F): It refers to the ease of reaching activity areas or urban services from a certain location or by an individual. Healthcare accessibility is an important component of public facilities since they supply people with essential medical services, and their distribution and accessibility directly impact customers’ health [17].
In order to deal with the ambiguity and fuzziness caused by subjective perception and human experience during the decision-making process, decision-makers typically discover that interval judgments are more secure than fixed-value judgments. This is primarily because of the ambiguous nature of the comparison process, which prevents them from expressing their views explicitly. This paper presents a novel methodology combining Fuzzy AHP and GRA methodologies. This methodology uses Fuzzy AHP to evaluate the weights of criteria, and Fuzzy GRA is then applied to rank the alternatives. Fig. 3 depicts the proposed method in detail.
Figure 3: Proposed approach
The fuzzy triangular numbers (TFN) can be described as (l, m, u), indicating the least likely (l), most promising (m), and largest conceivable (u) values in TFN (Tab. 1).
TFN can be defined as follows:
(a˜M)={0,ifa<m,a−lm−lifl≤a≤m,u−au−mifm≤a≤u,0,ifa>u,(1)
˜M=(Mo(y),Mi(y))=[l+(m−l)y,u+(m−u)y],y∈[0,1](2)
where o(y) and i(y) denote a fuzzy number’s left and right sides, respectively. The following illustrates fundamental computations involving two positive TFN, (l1,m1,u1) and (l2,m2,u).
(l1,m1,u1)+(l2,m2,u2)=(l1+l2,m1+m2,u1+u2)
((l1,m1,u1))−(l2,m2,u2)=(l1−l2,m1−m2,u1−u)
((l1,m1,u1))×(l2,m2,u2)=(l1×l2,m1×m2,u1×u2)
(l1,m1,u1)(l2,m2,u2)=(l1/l2,m1/m2,u1/u2)(3)
This study adopts Buckley’s Fuzzy AHP approach [25] to generate criterion weights since extending to the fuzzy situation is straightforward and ensures a unique solution for the reciprocal comparison matrix. The steps of this method are more straightforward than those of previous Fuzzy-AHP methods. The following is a summary of Buckley’s Fuzzy AHP algorithm’s steps:
Step 1: The fuzzy pairwise comparison matrix ˜D=[˜aˆij] is constructed as follows:
˜D=[(1,1,1)˜a12…˜a1n˜a21(1,1,1)…˜a2n⋮⋮⋱⋮˜an1˜an2…(1,1,1)](4)
where˜aijטaji≈1and˜aij≅wiwj,i,j=1,2,…,n.
Step 2: The fuzzy geometric mean value ˜ri, for each criterion i is computed as follows:
˜ri=(˜ai1טai2ׅטain)1/n(5)
Step 3: The fuzzy weight ˜wi, for each criterion i is calculated as follows:
˜wi=˜ri×(˜r1+˜r2+˜r3…+˜rn)−1
where˜rk=(lk,mk,uk)and(˜rk)−1=(1/uk,1/mk,1/lk)(6)
Step 4: The fuzzy weights ˜wi=(li,mi,ui) are defuzzified by any defuzzification method; here we use the Center of Area (COA) method as follows:
The center-of-area defuzzification method is a way of transforming fuzzy triangular numbers into crisp numbers. For a convex fuzzy number →γ, a real number x* corresponding to its center of area of →γ can be estimated as follows:
x∗=∫μγ(x)xd(x)∫μγ(x)d(x)(7)
˜wi=li+mi+ui3(8)
Besides, the consistency rate of the matrix (CR) should be calculated, and CR values should be obtained lower than 0.1. It means matrix A in terms of decision-maker judgment is acceptable. CR can be calculated using Eqs. (9)–(13).
WSV=CM×MEANNM=CM×NMn(9)
CV=WSVMEAN(10)
λmax=CVn(11)
CI=λmax−nn−1(12)
CR=CIRI(13)
Step 1: In the first step, a panel of decision makers (DMs) who are knowledgeable about the HES process is established. In a group that has k DMs (i.e., DM1, DM2,…, DMk) are responsible for ranking (yjk) of each criterion (i.e., C1, C2,…, Cn) in increasing order using Eq. (14):
˜xij=1K[˜x1ij+⋯+˜xKij]=1K∑Ke=1˜xeij(14)
Step 2: Calculate the normalized decision matrix R. Given ˜xij=(˜aij,˜bij,˜cij) the normalized performance rating can be calculated using Eqs. (15)–(16):
˜rij=(aijc+j,bijc+j,cijc+j),i=1,⋯,m;j=1,⋯,nforJ∈B(15)
˜rij=(a−jcij,a−jbij,a−jaij),i=1,⋯,mandj=1,⋯,nforJ∈C
wherec+j=maxi{cij}anda−j=mini{aij}∀ii=1,…,m(16)
Step 3: Determine the reference series. The reference series can be defined using Eq. (17):
˜R0=[˜r01,˜r02,…,˜r0n,]where˜r0j=max(˜rij)j=1,…,n(17)
Step 4. Establish the distance matrix. The distance ˜δij between the reference value and each comparison value is given in Eq. (18):
˜δij=|˜r0j−˜rij|(18)
Step 5: Calculate the fuzzy grey relational coefficient. The fuzzy grey relational coefficient ˜ξij is defined using Eq. (19):
˜ξij=˜δmin+ζ˜δmax˜δij+ζ˜δmax˜δmax=max(˜δij),˜δmin=min(˜δij)andζresolvingcoefficientζϵ[0,1].(19)
Step 6: Estimate the fuzzy grey relational grade ˜γi by the relation using Eq. (20):
˜γi=∑nj=1˜wj˜ξij, i=1,…, m
where˜wjistheweightofthejthcriterion,andn∑j=1˜wj=˜1.(20)
The α-cut method is used in our paper to validate the methodology results and performance of the proposed solution. The α-cut method compares two fuzzy numbers A and B in terms of their α-cuts:
Aα=[aα−,aα+]andBβ=[bβ−,bβ+](21)
The α-cuts can be employed to turn the total weighted performance matrices into interval performance matrices, which provide left and right for each alternative.
Pα=(aleft1aright1aleft2aright2……aleftmarightm)
aleft=α × (m − l) + l
aright=u−a×(u−m)(22)
And then the interval matrices are converted into crisp values. It is done by applying the and λ values are ranged between 0 and 1:
Cλ=(Cλ1Cλ2….Cλm)
Cλ=λ×aright+(1−λ)×aleft(23)
Step 8. Rank the alternatives in accordance with the value of grey relational grade; the bigger the value is, the better is among the alternatives.
This study examines the relationship between service quality and patient satisfaction within the Vietnamese healthcare system. In this study, 20 hospitals numbered H1 through H20 were selected to validate the model’s efficacy and robustness. First, the Fuzzy AHP approach is applied to weight each criterion of service quality dimensions in the healthcare industry based on the literature and the opinions of industry experts. Second, the Fuzzy GRA method is adopted to rank the healthcare service quality of selected Hanoi-based hospitals because the northern area of Vietnam has one of the most significant healthcare expenditures and is one of the top healthcare performers.
The quality structure of the healthcare service has been described based on a comprehensive review of the relevant literature in the field and extensive consultation with several healthcare managers and experts, physicians, and a representative sample of in-patients and out-patients. Remarkably, the complete structure of healthcare service quality is divided into six principal dimensions: Tangibles (A), Healthcare Staff (B), Responsiveness (C), Relationships (D), Support Service (E), and Accessibility (F).
Pair-wise comparison matrices of criteria was conducted by a group of 12 experts as decision-makers using linguistic terms (Tab. 1). The following Fuzzy AHP method includes calculating the six main dimensions to demonstrate the consistency ratio. Tabs. 2–5 illustrates the computation process of FAHP model, respectively.
To calculate the performance rating score’s consistency ratio (CR), the linguistic assessments in terms of triangular fuzzy numbers are transformed to a crisp value using the pessimistic (lower bound) and optimistic (upper bound) values of the fuzzy comparison matrix. The non-fuzzy comparison matrix for the primary criteria is shown in Tab. 4 using Eqs. (6)–(7):
To obtain the priority vectors for the Fuzzy AHP model’s criteria, the normalized pairwise comparison matrix is constructed by dividing each column sum by the column sum of each column. The priority vector is then determined by averaging the row elements in the normalized matrix, as shown in Tab. 4. The CR computations are used to determine the consistency of the pairwise comparison matrix using Eqs. (9)–(13):
A12=SIA12SUMB2=1.1898.230=0.145
MEANA1=(0.111+0.145+0.097+0.100+0.136+0.088)/6=0.113
WSV=[10.8411.6032.4261.4771.6581.18911.5651.5501.4631.4630.6240.63911.2602.1820.7490.4120.6450.79410.6670.6030.6770.6840.4581.54210.6240.6030.6841.3351.6581.6031]×[0.1130.1180.1670.2410.2310.149]=[0.0690.7221.0201.4821.3150.911]
CV=[0.1130.1180.1670.2410.2310.149]/[0.0690.7221.0201.4821.3150.911]=[6.1286.1276.1086.1516.1886.110]
The largest eigenvector (max) is computed in order to obtain the consistency index (CI), the random index (RI), and the consistency ratio (CR):
λmax=6.128+6.127+6.108+6.151+6.188+6.1106=6.134
CI=λmax−nn−1=6.134−66−1=0.027
with RI = 1.199 and n = 6, the CR value is calculated as below:
CR=CIRI=0.0271.199=0.023
Consistency ratio CR = 0.023 < 0.1 indicates that the result is satisfactory.
As shown in Tab. 5, Resposiveness was identified as the most important dimension to assess service quality in the healthcare sector according to the weight (0.236). The Relationship was placed in the second rank, considering its lower weight at 0.206 and following by Healthcare Staff (0.165), Support Service (0.152), Accessibility (0.123) and Tangibility (0.118) were finally comprised the next priorities for increasing satisfaction with the service quality in the healthcare industry. Our findings are consistent with the results of previous studies [26–28]. Their results showed that the most robust correlation relationships with patients’ satisfaction emerge with responsiveness and assurance dimensions, the weakest–empathy. On the other hand, as studied by Pekkaya et al. [26], Tangibility was measured as the highest. And their results suggested that patients demographically differentiate healthcare service quality in terms of age, income, and service type, but not for gender, marital status, educational level, and profession. Moreover, reliability is observed as the most determinant dimension for outpatients’ satisfaction. Furthermore, Shafei et al. [29] also confirmed an eight-construct framework: hospital premises and employees; doctor medical service; nursing medical service; diagnostic medical service; admission; discharge; rooms and housekeeping; and meals that had a significant effect on healthcare service quality. Healthcare Staff had a significant effect on healthcare service quality. These findings align with prior studies [30–32].
4.2 Results of Fuzzy GRA Method
The fuzzy relational grade is calculated Eq. (13) with respect to the criteria weights, which are obtained by Fuzzy-AHP shown in Tab. 5. The Fuzzy Grey Relational Coefficient (FGRC) is calculated by applying Eq. (12) and it is shown in Tab. 6. Here, we use the resolving coefficient ζ = 0, 5 to calculate the FGRC using Eqs. (14)–(20).
Tab. 7 showed that the top 5 hospitals, including H12, H10, H20, H19, and H18. Hospital H12 and H10 has several service quality aspects that have the same rating values such as availability of marked signs in the hospital, service staff with good communication skills, the cordial attitude of service staff, consideration of individual needs of patients by medical staff, taking the advice of medical staffs to patients staying at home, trusted medical staff with professional competence of healthcare, lighting condition of the ward, and number & quality of available bathrooms in the hospital. These hospitals, however, still have two worst aspects: the quality and cleanliness of the bed linen and ventilation and sanitation of the ward. When evaluated the physical capacities of the hospitals, it has clearly stated that H20 and H18 have more space and medical resources than others. Hospital H10 is a public hospital and has 500 beds and 200 doctors. The second public hospital evaluated in this study is Hospital H1, and it serves 300 beds and 183 doctors. The other hospital Hospital H6 serves 205 beds and 162 doctors. As an overall evaluation, hospital H12 is in a better position in providing service quality from the viewpoints of the health experts. The experts who evaluated these institutions concerning the criteria are more experienced staff in their hospitals. This study also proved that the service quality of private hospitals is better than that of public hospitals. Although governmental departments rarely subsidize private hospitals, they must provide better services to retain patients’ loyalty and create innovations that are better than those developments that the government has made in health in recent years have strengthened the serviceability of public hospitals. Unlike private hospitals, the operations budgets of public hospitals are guaranteed mainly by governmental subsidization and taxpayers’ tax. They have the financial and managerial power to provide health care at a reasonable level. As a creative contribution to this application case study, the weights of main and sub-criteria and ranking orders for different evaluation groups are analyzed.
First, the concepts of sensitivity analysis are used to verify that the proposed method has rationality and stability, when the conditions of resolving coefficient value and defuzzification methods do not affect the results. This study uses each resolving coefficient value to demonstrate that each of them does not affect the results. The resolving coefficient values on the x axis and the defuzzified results of the proposed methodology on the y axis are given. x* (COA) and Cλ (α-cut) method results are shown in the figure, separately. The resolving coefficient values are used to examine the proposed approach between ζ = 0.1 and ζ = 1 using Eqs. (21)–(23). The results show that the variation of the x* and Cλvalues of each alternative by using various resolving coefficient values, and also that the ranking orders of the three alternatives are the same, despite changing from a resolving coefficient value of ζ = 0.1 to ζ = 1. Therefore, this study can confirm that the results of the ranking orders of all alternatives by using the proposed approach are reliable. Then, this study highlights that various resolving coefficient values do not affect the results of ranking order of the 20 hospitals.
In this study, a model proposal is presented based on the concept of Fuzzy AHP and GRA techniques to provide an accurate decision-making process for evaluating the hospital service quality under a uncertainty and vagueness environment. A case study including 6 service quality criteria and 20 public and private hospitals in Hanoi, Vietnam, assessed by 12 evaluators is performed to demonstrate the applicability and validity of the proposed approach. The main advantage of this study can be sort out: (i), an integrated model produces reliable and suggestive outcomes better representing the vagueness of the decision-making process. (ii) Improving the top criteria can help them provide better hospital service quality, the most concern to all hospitals and the authority. (ii), The acquired result can also be used to analyze the weaknesses and strengths between the private and public healthcare system and in each chosen hospital.
In the light of practical implications, strategic efforts toward the service quality improvement should concern the following service criteria: Healthcare staff and Responsiveness and, particularly: Staff capacity to work as a team, Ability of doctors to understand patient needs, Staff reliability and Swiftness of registration and admission procedures. Conversely, the obtained discrepancies characterizing managers’ viewpoints on service quality highlight that the importance of the service criteria Responsiveness, Healthcare staff and Relationships is not correctly perceived by them. Thus, actions should focus on improvements in marketing research, direct interactions between managers and stakeholders, and bottom-up internal communications to understand what stakeholders want correctly. Furthermore, effective internal communications of achievements in service quality should reduce the discrepancies between stakeholders’ needs and how staff perceive those needs. According to Vietnam’s National Health Policy and the Hanoi Health Department, healthcare will be made more accessible to the community on an equitable basis with provisions for meeting specific health needs, the quality of healthcare will be improved to a level acceptable to the community and service providers, and healthcare will be made more efficient and cost-effective. The primary purpose of the government’s health strategy is to improve patient care by reorganising the healthcare delivery system, especially at the district and provincial levels. The National Quality Policy of Vietnam proposes an all-encompassing approach to quality management, encompassing all economic sectors and social groups. Improvements in Service Quality Delivery is one of the most crucial parts of the Master Plan for Health. According to the study, a quality assurance strategy would be developed to support high-quality services that are fundamental to the culture of the health sector. The Ministry of Health will take the initiative in establishing quality assurance in a methodical manner that builds on existing quality improvement efforts while fostering team spirit and patient and customer focus. Clinical accountability, the creation of peer-group reviews and clinical audits, and ways of monitoring patient satisfaction and comprehensive quality management of services will all be part of this strategy. The necessity for a system of ongoing professional education for physicians, nurses, and other healthcare workers shall be considered with relevant professional and regulatory authorities at regular intervals. To improve and sustain public and private sector standards, defined norms and accreditation systems will be implemented. Professional organizations, medical schools, and service providers will all be involved in the development process to assure long-term viability.
While the proposed approach has added operational value to the healthcare service, there are still some limitations to this study. First, this study is heavily reliant on expert opinions. As a result, the findings are based on individual perspectives, knowledge, and judgment. Second, the authors disregarded potential interactions and relationships among the criteria. Another notable limitation is that this study tested the robustness of the proposed model using sensitive analysis by α-cut method; however, there is no comparison between previous methods; thus, future research should conduct a comparative analysis with the established methods from the literature to have more robustness.
Acknowledgement: The author would like to thank the experts who contributed to the article.
Funding Statement: The authors received no specific funding for this study.
Conflicts of Interest: The authors declares that there are no conflicts of interest to report regarding the present study.
References
1. T. Lupo, “A fuzzy framework to evaluate service quality in the healthcare industry: An empirical case of public hospital service evaluation in sicily,” Applied Soft Computing, vol. 40, pp. 468–478, 2016. [Google Scholar]
2. P. H. Nguyen, “A fuzzy analytic hierarchy process (FAHP) based on SERVQUAL for hotel service quality management: Evidence from Vietnam,” The Journal of Asian Finance, Economics, and Business, vol. 8, no. 2, pp. 1101–1109, 2021. [Google Scholar]
3. C. White and Y. T. Yu, “Satisfaction emotions and consumer behavioral intentions,” Journal of Services Marketing, vol. 19, no. 6, pp. 411–420, 2005. [Google Scholar]
4. S. S. Andaleeb, “Determinants of customer satisfaction with hospitals: A managerial model,” International Journal of Health Care Quality Assurance, vol. 11, no. 6, pp. 181–187, 1998. [Google Scholar]
5. M. D. Clemes, L. K. Ozanne and W. L. Laurensen, “Patients’ perceptions of service quality dimensions: An empirical examination of health care in New Zealand,” Health Marketing Quarterly, vol. 19, no. 1, pp. 3–22, 2001. [Google Scholar]
6. H. C. Barksdale Jr, J. T. Johnson and M. Suh, “A relationship maintenance model: A comparison between managed health care and traditional fee-for-service,” Journal of Business Research, vol. 40, no. 3, pp. 237–247, 1997. [Google Scholar]
7. K. Braunsberger and R. H. Gates, “Patient/enrollee satisfaction with healthcare and health plan,” Journal of Consumer Marketing, vol. 19, no. 7, pp. 575–590, 2002. [Google Scholar]
8. W. I. Lee, C. W. Chen and C. -H. Wu, “Relationship between quality of medical treatment and customer satisfaction-A case study in dental clinic association,” International Journal of Innovative Computing, Information and Control, vol. 6, no. 4, pp. 1805–1822, 2010. [Google Scholar]
9. J. Das and J. Hammer, “Money for nothing: The dire straits of medical practice in Delhi, India,” Journal of Development Economics, vol. 83, no. 1, pp. 1–36, 2007. [Google Scholar]
10. C. E. Hegji, D. R. Self and C. S. C. Findley, “The link between hospital quality and services profitability,” International Journal of Pharmaceutical and Healthcare Marketing, vol. 1, no. 4, pp. 290–303, 2007. [Google Scholar]
11. H. Çaha, “Service quality in private hospitals in Turkey,” Journal of Economic & Social Research, vol. 9, no. 1, pp. 55–69, 2007. [Google Scholar]
12. K. E. Clow and D. W. Vorhies, “Building a competitive advantage for service firms: Measurement of consumer expectations of service quality,” Journal of Services Marketing, vol. 7, no. 1, pp. 22–32, 1993. [Google Scholar]
13. M. L. Tseng, “A causal and effect decision making model of service quality expectation using grey-fuzzy DEMATEL approach,” Expert Systems with Applications, vol. 36, no. 4, pp. 7738–7748, 2009. [Google Scholar]
14. T. L. Nguyen, P. H. Nguyen, H. A. Pham, T. G. Nguyen, D. T. Nguyen et al., “A novel integrating data envelopment analysis and spherical fuzzy MCDM approach for sustainable supplier selection in steel industry,” Mathematics, vol. 10, no. 11, pp. 1897, 2022. [Google Scholar]
15. P. H. Nguyen, T. T. Dang, K. A. Nguyen and H. A. Pham, “Spherical fuzzy WASPAS-based entropy objective weighting for international payment method selection,” Computers, Materials & Continua, vol. 72, no. 1, pp. 2055–2075, 2022. [Google Scholar]
16. P. H. Nguyen, “A hybrid grey DEMATEL and PLS-SEM model to investigate COVID-19 vaccination intention,” Computers, Materials & Continua, vol. 72, no. 3, pp. 5059–5078, 2022. [Google Scholar]
17. J. J. H. Liou, C. C. Hsu, W. C. Yeh and R. H. Lin, “Using a modified grey relation method for improving airline service quality,” Tourism Management, vol. 32, no. 6, pp. 1381–1388, 2011. [Google Scholar]
18. P. H. Nguyen, “Spherical fuzzy decision-making approach integrating delphi and TOPSIS for package tour provider selection,” Mathematical Problems in Engineering, vol. 2022, pp. 29, 2022. http://www.10.1155/2022/4249079. [Google Scholar]
19. P. H. Nguyen, “Agricultural supply chain risks evaluation with spherical fuzzy analytic hierarchy process,” Computers, Materials & Continua, vol. 73, no. 2, pp. 4211–4229, 2022. [Google Scholar]
20. M. Mutlu, G. Tuzkaya and B. Sennaroğlu, “Multi-criteria decision making techniques for healthcare service quality evaluation: A literature review,” Sigma Journal of Engineering and Natural Sciences, vol. 35, no. 3, pp. 501–512, 2017. [Google Scholar]
21. Ž. Stević, D. Pamučar, A. Puška and P. Chatterjee, “Sustainable supplier selection in healthcare industries using a new MCDM method: Measurement of alternatives and ranking according to Compromise solution (MARCOS),” Computers & Industrial Engineering, vol. 140, pp. 106231, 2020. [Google Scholar]
22. S. L. Si, X. Y. You, H. C. Liu and J. Huang, “Identifying key performance indicators for holistic hospital management with a modified DEMATEL approach,” International Journal of Environmental Research and Public Health, vol. 14, no. 8, pp. 934–951, 2017. [Google Scholar]
23. A. Y. Korkusuz, U. H. İnan, Y. Özdemir and H. Başlıgil, “Occupational health and safety performance measurement in healthcare sector using integrated multi criteria decision making methods,” Journal of the Faculty of Engineering and Architecture of Gazi University, vol. 35, no. 1, pp. 81–96, 2020. [Google Scholar]
24. E. A. Adalı and A. Tuş, “Hospital site selection with distance-based multi-criteria decision-making methods,” International Journal of Healthcare Management, vol. 14, no. 2, pp. 534–544, 2021. [Google Scholar]
25. J. J. Buckley, “Fuzzy hierarchical analysis,” Fuzzy Sets and Systems, vol. 17, no. 3, pp. 233–247, 1985. [Google Scholar]
26. M. Pekkaya, Ö. Pulat İmamoğlu and H. Koca, “Evaluation of healthcare service quality via servqual scale: An application on a hospital,” International Journal of Healthcare Management, vol. 12, no. 4, pp. 340–347. 2019. [Google Scholar]
27. V. Buckė, J. Ruževičius and R. Buckus, “Service quality management at Lithuanian healthcare institutions,” Calitatea, vol. 21, no. 174, pp. 49–55, 2020. [Google Scholar]
28. A. Shabbir, S. A. Malik and S. A. Malik, “Measuring patients’ healthcare service quality perceptions, satisfaction, and loyalty in public and private sector hospitals in Pakistan,” International Journal of Quality & Reliability Management, vol. 33, no. 5, 2016. https://doi.org/10.1108/IJQRM-06-2014-0074. [Google Scholar]
29. I. Shafei, J. A. Walburg and A. F. Taher, “Healthcare service quality: What really matters to the female patient?” International Journal of Pharmaceutical and Healthcare Marketing, vol. 9, no. 4, pp. 369–39, 2015. [Google Scholar]
30. Đ. O. Došen, V. Škare, V. Cerfalvi, Ž Benceković and T. Komarac, “Assessment of the quality of public hospital healthcare services by using SERVQUAL,” Acta Clinica Croatica, vol. 59, no. 2, pp. 285–292, 2020. [Google Scholar]
31. A. M. Mosadeghrad, “Healthcare service quality: Towards a broad definition,” International Journal of Health Care Quality Assurance, vol. 26, no. 3, pp. 203–219, 2013. [Google Scholar]
32. C. H. Lin, S. F. Siao, H. H. Tung, K. P. Chung and S. C. Shun, “The gaps of healthcare service quality in nurse practitioner practice and its associated factors from the patients’ perspective,” Journal of Nursing Scholarship, vol. 53, no. 3, pp. 378–386, 2021. [Google Scholar]
Cite This Article
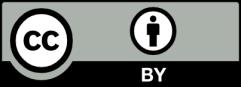
This work is licensed under a Creative Commons Attribution 4.0 International License , which permits unrestricted use, distribution, and reproduction in any medium, provided the original work is properly cited.